What is sentiment analysis?
Sentiment analysis, also known as opinion mining, is a branch of Natural Language Processing (NLP) that uses machine learning and text analysis to identify and extract subjective information from source materials. It is a powerful tool used in marketing to understand customer sentiment towards products, brands, or services by analyzing online conversations and feedback.
Sentiment analysis can be applied to any text data, including social media posts, reviews, surveys, and more. It helps businesses to understand their customers better, improve their products and services, and make informed decisions. This article will delve into the details of sentiment analysis, its types, applications, benefits, and challenges.
Understanding Sentiment Analysis
Sentiment analysis is a computational study of people’s opinions, appraisals, and emotions towards entities such as products, services, organizations, individuals, issues, events, topics, and their attributes. It involves the use of natural language processing, text analysis, and computational linguistics to identify and extract subjective information from source materials.
It is an automated process that analyzes the text data to understand the sentiments behind them. It can be positive, negative, or neutral. The main goal of sentiment analysis is to classify the polarity of a given text at the document, sentence, or feature/aspect level.
Components of Sentiment Analysis
Sentiment analysis involves several components, each playing a crucial role in the process. These include natural language processing, machine learning, and text analysis. Natural language processing is a field of artificial intelligence that enables computers to understand and interpret human language. It is used in sentiment analysis to process and analyze the text data.
Machine learning, on the other hand, is used to train the sentiment analysis model. It involves feeding the model with a large amount of text data, along with the corresponding sentiments. The model learns from this data and uses it to predict the sentiment of new, unseen text data. Text analysis is the process of transforming unstructured text data into structured data for analysis.
Types of Sentiment Analysis
There are several types of sentiment analysis, each with its own unique approach and use cases. These include fine-grained sentiment analysis, emotion detection, aspect-based sentiment analysis, and intent analysis. Fine-grained sentiment analysis involves not only categorizing the sentiment as positive, negative, or neutral but also the intensity of the sentiment.
Emotion detection, as the name suggests, involves detecting emotions like happiness, frustration, anger, sadness, etc., from the text data. Aspect-based sentiment analysis involves identifying different aspects or features mentioned in the text and the sentiment associated with each aspect. Intent analysis involves identifying the intent behind the sentiment, such as whether the user intends to purchase a product or not.
Applications of Sentiment Analysis
Sentiment analysis has a wide range of applications, particularly in the field of marketing. It is used to monitor social media and online reviews to understand customer sentiment towards products, brands, or services. This helps businesses to understand their customers better, improve their products and services, and make informed decisions.
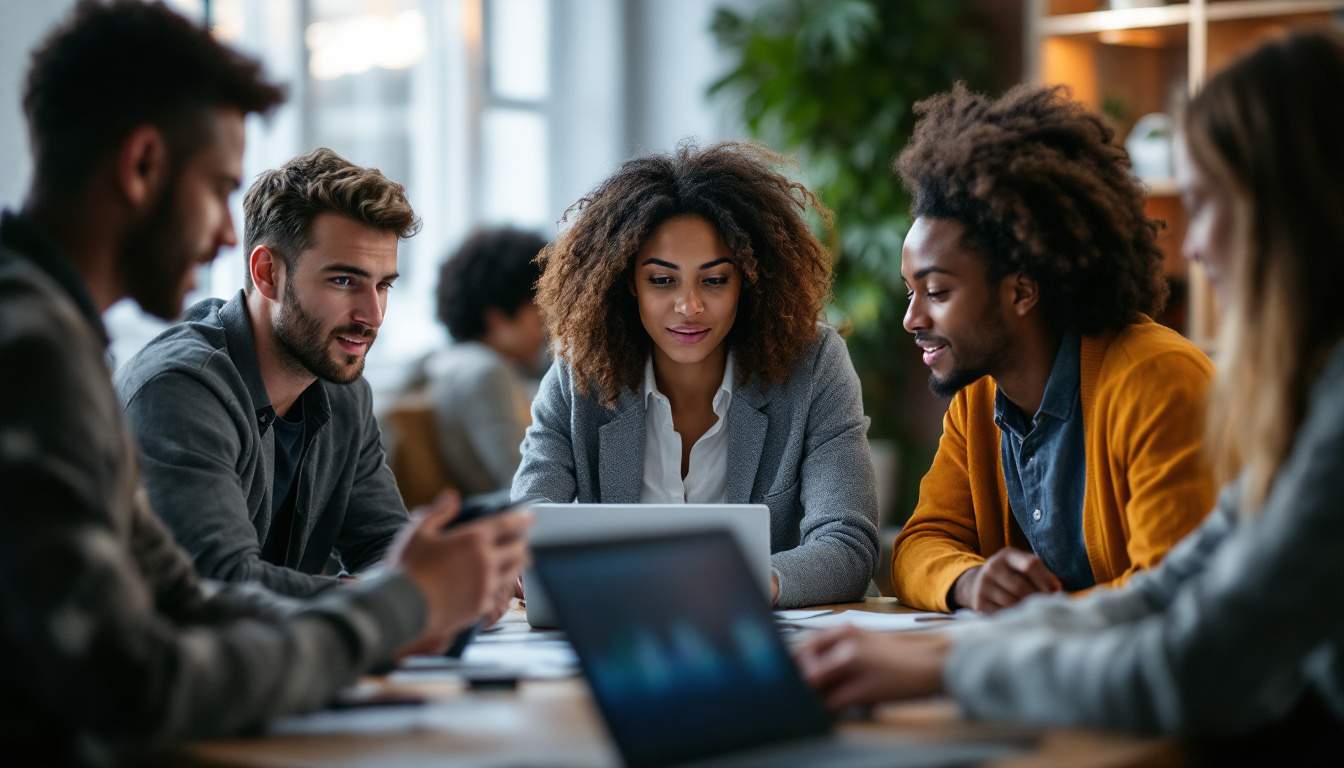
It is also used in customer service to identify and prioritize urgent issues based on the sentiment of the customer feedback. In public relations, sentiment analysis is used to monitor public sentiment towards a company or brand. It is also used in politics to understand public sentiment towards candidates or issues.
Social Media Monitoring
Social media is a goldmine of customer sentiment data. Businesses use sentiment analysis to monitor social media platforms for mentions of their brand, products, or services and analyze the sentiment behind these mentions. This helps them to understand how their customers feel about their brand and respond to any negative sentiment before it escalates.
It also helps them to identify positive sentiment and leverage it for marketing purposes. For example, if a customer posts a positive review of a product on social media, the business can share this review on their own social media platforms to promote the product.
Customer Service
Customer service is another area where sentiment analysis is widely used. It helps businesses to identify and prioritize urgent issues based on the sentiment of the customer feedback. For example, if a customer posts a negative review of a product on social media, the business can use sentiment analysis to identify this negative sentiment and respond to the customer promptly.
It also helps businesses to understand the overall sentiment of their customer feedback and use this information to improve their customer service. For example, if the overall sentiment of the customer feedback is negative, the business can take steps to improve their customer service and address the issues raised by the customers.
Benefits of Sentiment Analysis
Sentiment analysis offers several benefits to businesses. It helps businesses to understand their customers better, improve their products and services, and make informed decisions. It also helps businesses to identify and respond to negative sentiment before it escalates, and leverage positive sentiment for marketing purposes.
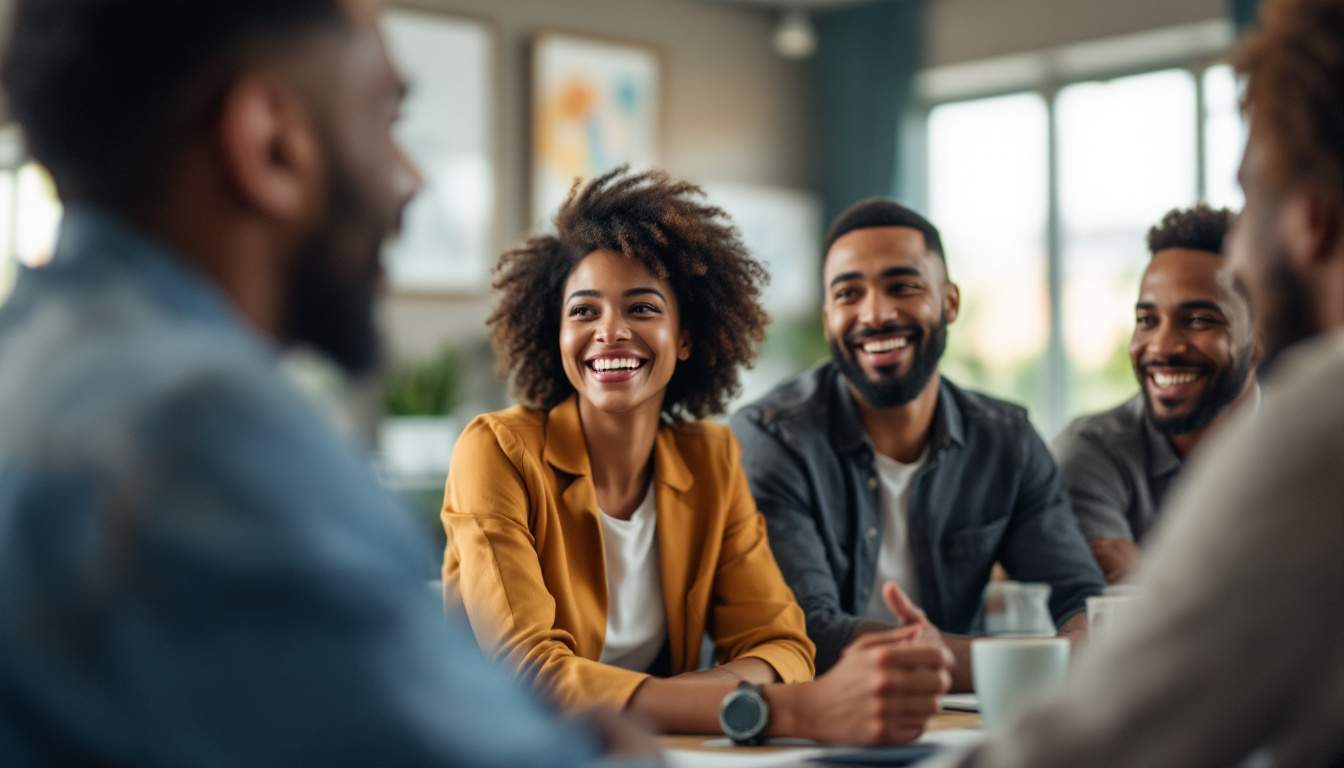
By understanding customer sentiment, businesses can gain insights into customer preferences, needs, and expectations. This can help them to tailor their products and services to meet customer needs and expectations, leading to increased customer satisfaction and loyalty.
Improved Customer Understanding
One of the key benefits of sentiment analysis is improved customer understanding. By analyzing customer sentiment, businesses can gain insights into customer preferences, needs, and expectations. This can help them to tailor their products and services to meet customer needs and expectations, leading to increased customer satisfaction and loyalty.
For example, if a business finds that customers are expressing negative sentiment towards a particular product feature, they can use this information to improve the feature and meet customer expectations.
Proactive Issue Resolution
Sentiment analysis also enables proactive issue resolution. By identifying negative sentiment, businesses can respond to issues before they escalate. This can help to prevent damage to the brand reputation and improve customer satisfaction.
For example, if a customer posts a negative review of a product on social media, the business can use sentiment analysis to identify this negative sentiment and respond to the customer promptly. This can help to resolve the issue and prevent it from escalating.
Challenges of Sentiment Analysis
Despite its benefits, sentiment analysis also has its challenges. These include the complexity of human language, the need for large amounts of training data, and the difficulty of interpreting the results. Understanding these challenges can help businesses to implement sentiment analysis more effectively.
Human language is complex and nuanced, with many different ways to express the same sentiment. This makes it challenging for sentiment analysis algorithms to accurately identify and classify sentiment. Additionally, sentiment can be influenced by context, which can be difficult for algorithms to understand.
Complexity of Human Language
The complexity of human language is one of the main challenges of sentiment analysis. Human language is complex and nuanced, with many different ways to express the same sentiment. This makes it challenging for sentiment analysis algorithms to accurately identify and classify sentiment.
For example, sarcasm and irony can be difficult for algorithms to detect. A statement that is sarcastic or ironic can have the opposite sentiment to what the words alone would suggest. Additionally, sentiment can be influenced by context, which can be difficult for algorithms to understand.
Need for Large Amounts of Training Data
Another challenge of sentiment analysis is the need for large amounts of training data. To accurately identify and classify sentiment, sentiment analysis algorithms need to be trained on a large amount of text data, along with the corresponding sentiments. This can be time-consuming and resource-intensive.
Additionally, the training data needs to be representative of the data that the algorithm will be analyzing. If the training data is not representative, the algorithm may not perform well on the actual data.
Conclusion
Sentiment analysis is a powerful tool that can help businesses to understand their customers better, improve their products and services, and make informed decisions. Despite its challenges, it offers numerous benefits and has a wide range of applications, particularly in the field of marketing.
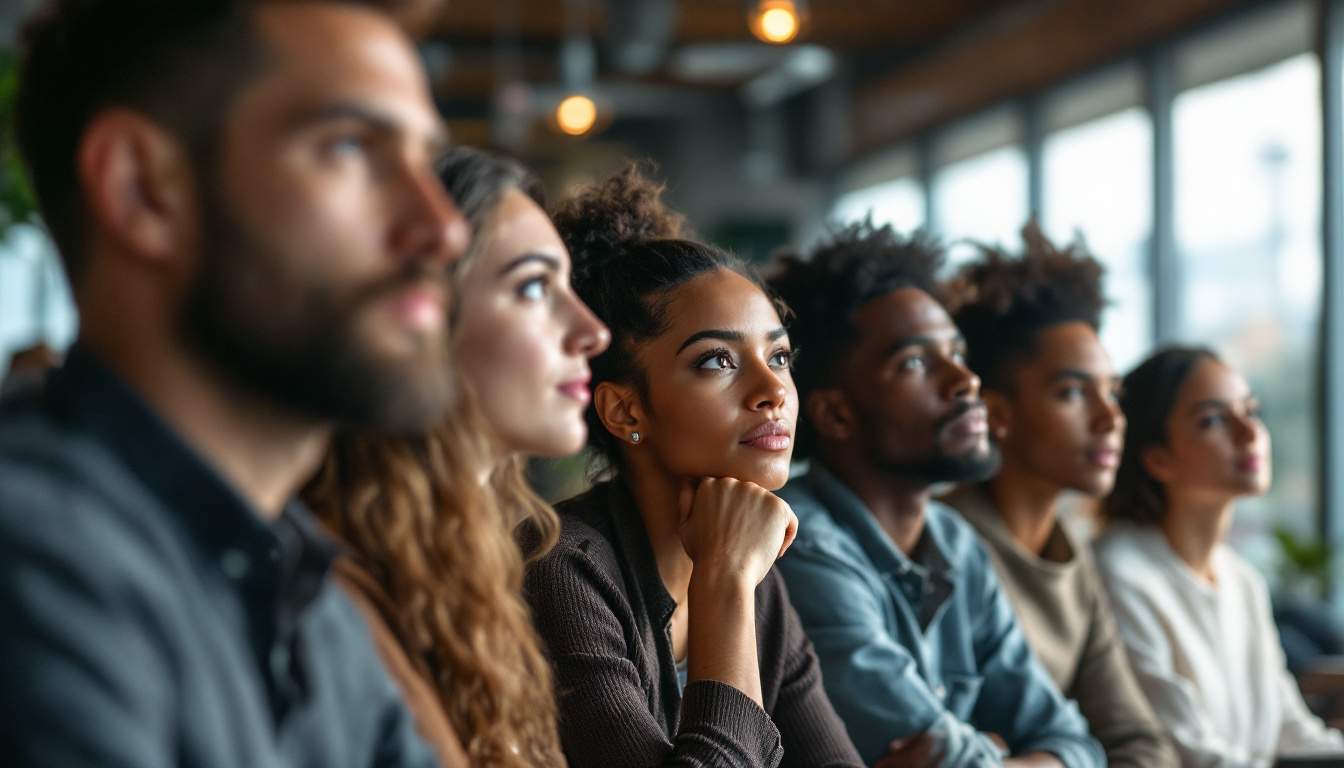
By understanding the components, types, applications, benefits, and challenges of sentiment analysis, businesses can implement it more effectively and leverage it to gain a competitive edge.