What is revenue attribution?
Revenue attribution refers to the process of identifying and assigning credit to the marketing touchpoints that lead to a conversion or sale. This process helps marketers understand which of their efforts are most effective in driving revenue, allowing them to optimize their strategies for maximum return on investment. The concept of revenue attribution is deeply intertwined with the broader marketing landscape, touching on areas such as customer journey mapping, multi-channel marketing, and data analytics.
Understanding revenue attribution requires a deep dive into the various methodologies used, the technologies that enable it, and the challenges that marketers face in implementing it. This comprehensive glossary entry will provide an in-depth look at all these aspects, offering a thorough understanding of what revenue attribution is and how it works. Let’s start by exploring the different types of revenue attribution models that are commonly used in marketing.
Types of Revenue Attribution Models
There are several different models that marketers can use to assign credit to their various marketing touchpoints. These models range from simple, single-touch models to more complex, multi-touch models. The choice of model can significantly impact the insights gained from the attribution process, and thus, the subsequent marketing strategy.
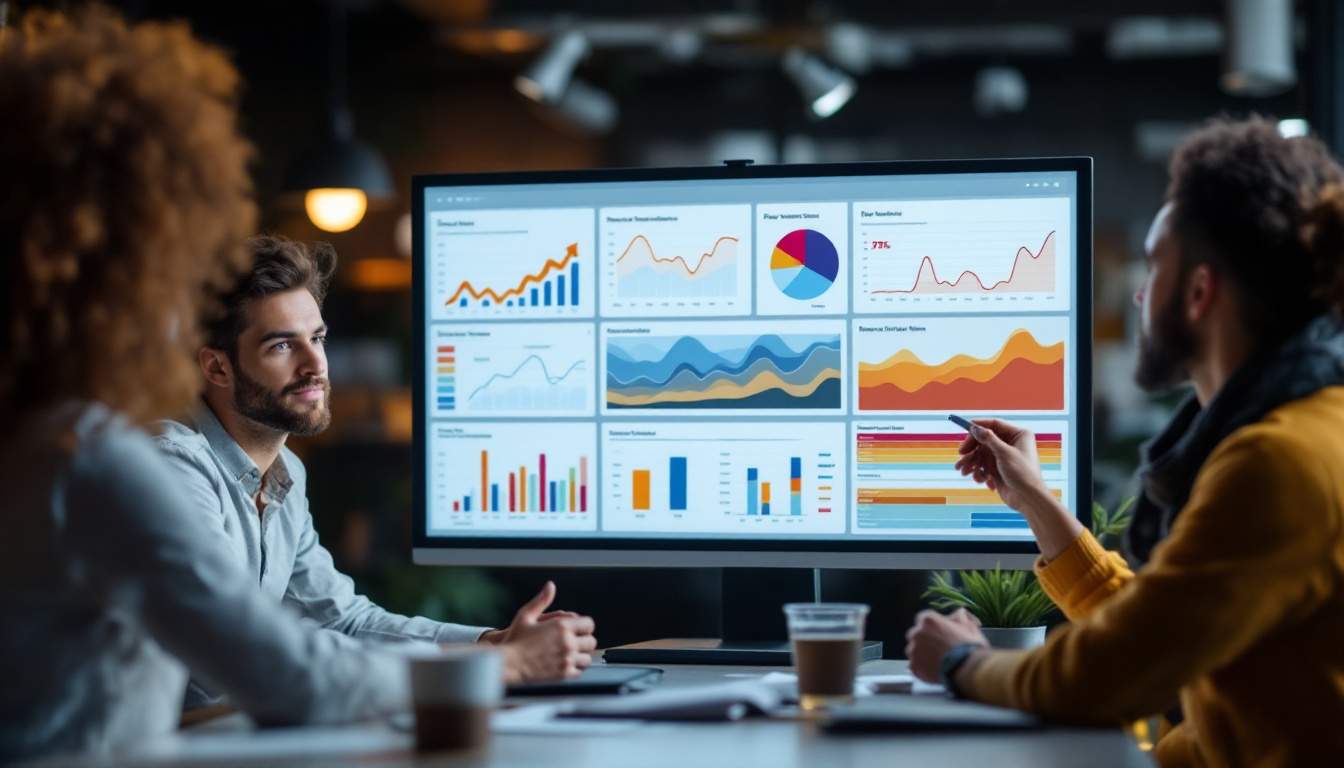
Each model has its strengths and weaknesses, and the best choice often depends on the specific circumstances of the business and its marketing efforts. Let’s delve into the details of some of the most common revenue attribution models.
Single-Touch Attribution Models
Single-touch attribution models assign all the credit for a conversion to a single touchpoint. These models are straightforward and easy to implement, but they often oversimplify the customer journey. There are two main types of single-touch attribution models: first-touch and last-touch.
The first-touch model assigns all the credit to the first marketing touchpoint that the customer interacted with, while the last-touch model assigns all the credit to the last touchpoint before the conversion. Both of these models can provide valuable insights, but they also risk ignoring the impact of other touchpoints that may have influenced the customer’s decision.
Multi-Touch Attribution Models
Multi-touch attribution models, on the other hand, attempt to account for all the touchpoints that a customer interacts with on their journey to conversion. These models are more complex and can provide a more nuanced view of the customer journey. However, they also require more data and sophisticated analysis techniques.
There are several types of multi-touch attribution models, including linear, time decay, and U-shaped models. The linear model assigns equal credit to all touchpoints, the time decay model assigns more credit to touchpoints closer to the conversion, and the U-shaped model assigns more credit to the first and last touchpoints, with the remaining credit distributed equally among the other touchpoints.
Technologies for Revenue Attribution
Implementing revenue attribution requires the use of various technologies to collect, analyze, and visualize data. These technologies range from customer relationship management (CRM) systems to data analytics platforms. Understanding these technologies is crucial for effectively implementing and leveraging revenue attribution.
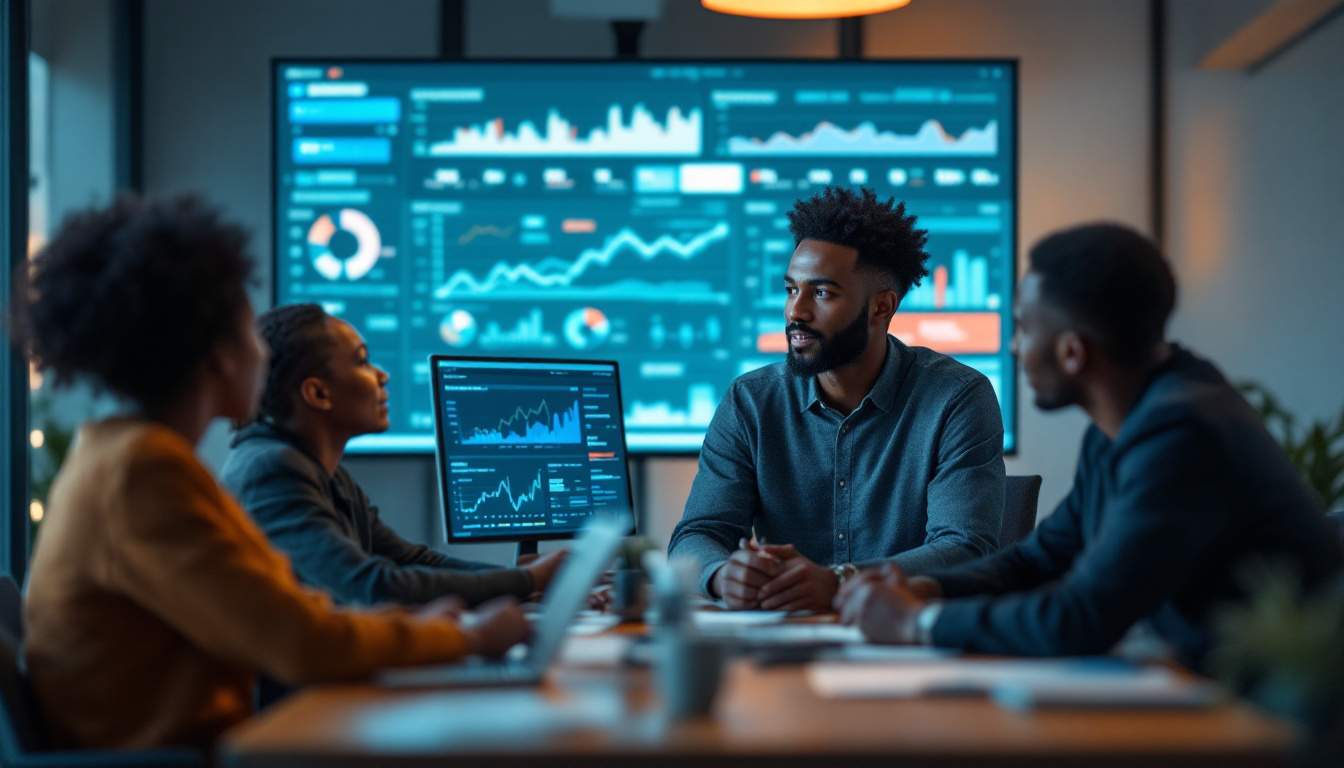
CRM systems, for example, can track customer interactions across various channels and touchpoints, providing the raw data needed for attribution analysis. Data analytics platforms, on the other hand, can process this data and apply the chosen attribution model, generating actionable insights for marketers.
Data Collection Technologies
Data collection is the first step in the revenue attribution process. This involves tracking customer interactions across various channels and touchpoints, from initial contact to conversion. Various technologies can facilitate this process, including cookies, pixel tags, and CRM systems.
Cookies and pixel tags can track online interactions, such as website visits and ad clicks, while CRM systems can track offline interactions, such as phone calls and in-store purchases. These technologies provide the raw data needed for attribution analysis, making them a crucial part of the revenue attribution infrastructure.
Data Analysis Technologies
Once the data has been collected, it needs to be analyzed to assign credit to the various touchpoints. This involves applying the chosen attribution model to the data, a process that can be facilitated by various data analysis technologies.
These technologies can range from simple spreadsheet software to sophisticated data analytics platforms. The choice of technology often depends on the complexity of the attribution model and the volume of data to be analyzed. Regardless of the specific technology used, the goal is to generate actionable insights that can inform marketing strategy.
Challenges in Implementing Revenue Attribution
Implementing revenue attribution is not without its challenges. These can range from technical issues, such as data collection and analysis, to strategic issues, such as choosing the right attribution model. Understanding these challenges can help marketers better navigate the revenue attribution landscape.
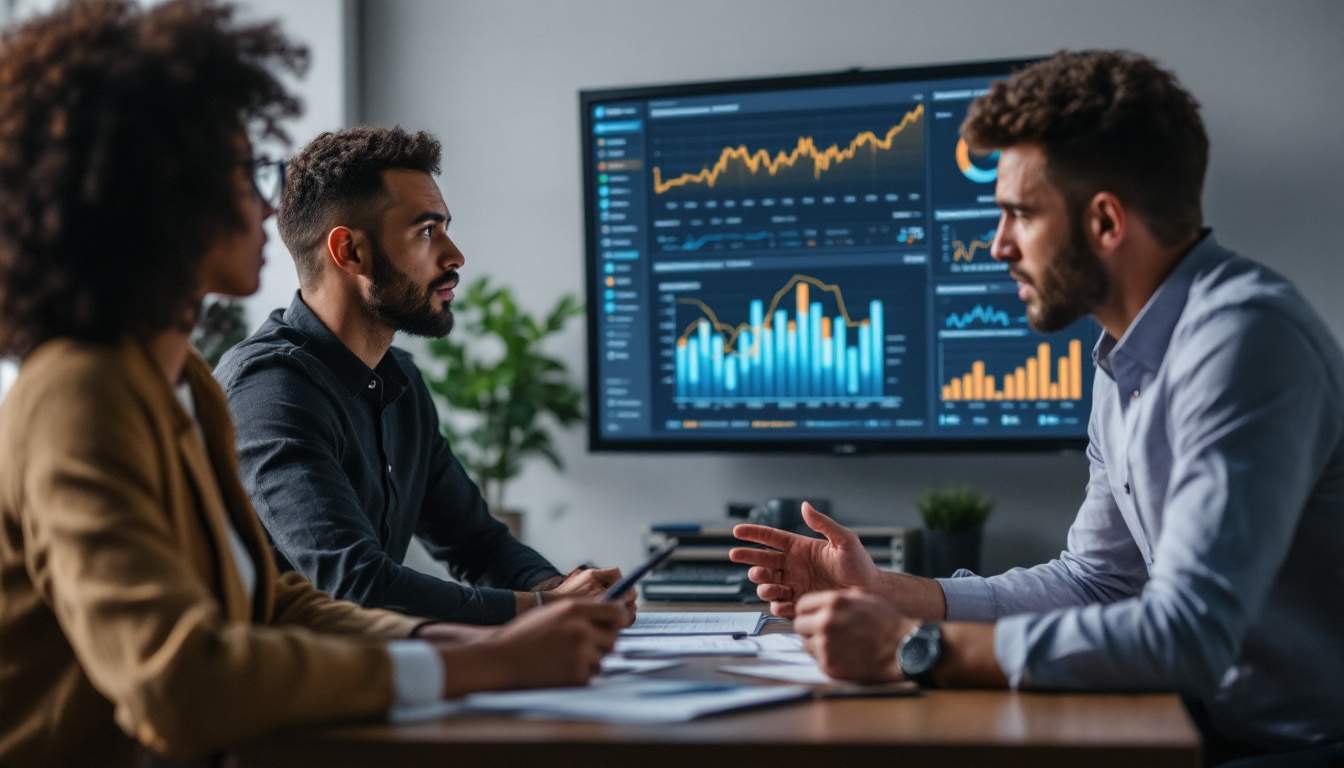
One of the main challenges in implementing revenue attribution is data collection. Tracking customer interactions across multiple channels and touchpoints can be technically complex, especially in a multi-device, multi-platform environment. This challenge can be exacerbated by privacy regulations, which can limit the types of data that can be collected and how it can be used.
Choosing the Right Attribution Model
Another challenge in implementing revenue attribution is choosing the right attribution model. As discussed earlier, each model has its strengths and weaknesses, and the best choice often depends on the specific circumstances of the business and its marketing efforts.
Choosing the wrong model can lead to skewed insights, which can in turn lead to suboptimal marketing strategies. Therefore, it’s crucial for marketers to understand the nuances of the different attribution models and to choose the one that best fits their needs.
Data Analysis and Interpretation
Data analysis and interpretation is another challenge in implementing revenue attribution. Even with the right data and the right model, interpreting the results can be complex. This is especially true for multi-touch attribution models, which can generate a large volume of data and require sophisticated analysis techniques.
Interpreting the results correctly is crucial for generating actionable insights. This often requires a deep understanding of the business and its customers, as well as the ability to translate data into strategic recommendations.
Conclusion
Revenue attribution is a powerful tool for marketers, allowing them to understand which of their efforts are most effective in driving revenue. By tracking customer interactions across multiple touchpoints and assigning credit to those that lead to a conversion, marketers can optimize their strategies for maximum return on investment.
However, implementing revenue attribution is not without its challenges. From data collection and analysis to choosing the right attribution model, there are many factors to consider. But with a thorough understanding of the concept and the right technologies, marketers can successfully navigate these challenges and leverage revenue attribution to its full potential.