What is propensity modeling?
Propensity modeling is a statistical approach used in marketing to predict the likelihood of a given individual to purchase a product, respond to a marketing campaign, or engage in any other kind of behavior that is beneficial to a business. This predictive modeling technique is based on historical data and various algorithms to forecast future behavior.
Propensity models are a key tool in the arsenal of modern marketers, enabling them to target their efforts more effectively and efficiently. By understanding the likelihood of different outcomes, marketers can tailor their strategies to the individuals most likely to respond positively, thereby maximizing their return on investment.
Understanding Propensity Modeling
At its core, propensity modeling is about predicting behavior. It uses a combination of historical data and statistical algorithms to forecast the likelihood of a particular outcome. This can be anything from the probability of a customer making a purchase, to the likelihood of a user clicking on a particular ad.
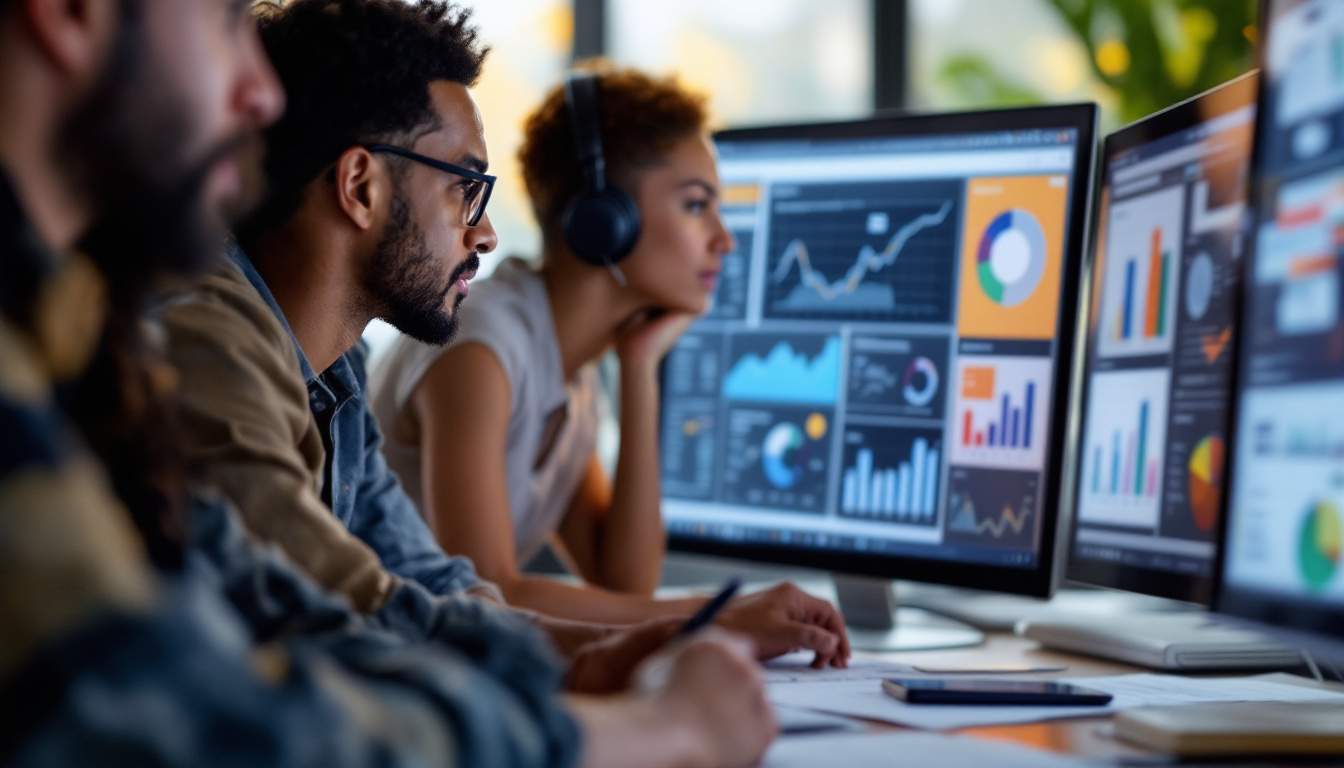
The power of propensity modeling lies in its ability to provide actionable insights. By understanding the likelihood of different outcomes, marketers can tailor their strategies to the individuals most likely to respond positively. This not only improves the effectiveness of marketing campaigns, but also helps to reduce wasted resources.
Components of Propensity Models
Propensity models are built using a variety of data sources. This can include demographic information, past purchase history, browsing behavior, and any other data that might be relevant to the behavior being predicted. The more data that is available, the more accurate the model is likely to be.
Once the data has been collected, it is analyzed using statistical algorithms. These algorithms look for patterns and relationships in the data that can be used to predict future behavior. The specific algorithms used can vary depending on the nature of the data and the behavior being predicted.
Types of Propensity Models
There are several different types of propensity models, each designed to predict a different kind of behavior. Some of the most common types include purchase propensity models, which predict the likelihood of a customer making a purchase, and response propensity models, which predict the likelihood of a customer responding to a marketing campaign.
Other types of propensity models include churn propensity models, which predict the likelihood of a customer discontinuing their use of a product or service, and upsell propensity models, which predict the likelihood of a customer purchasing a higher-value product or service.
Benefits of Propensity Modeling
Propensity modeling offers a number of benefits to marketers. One of the most significant is the ability to target marketing efforts more effectively. By understanding the likelihood of different outcomes, marketers can focus their resources on the individuals most likely to respond positively. This not only improves the effectiveness of marketing campaigns, but also helps to reduce wasted resources.
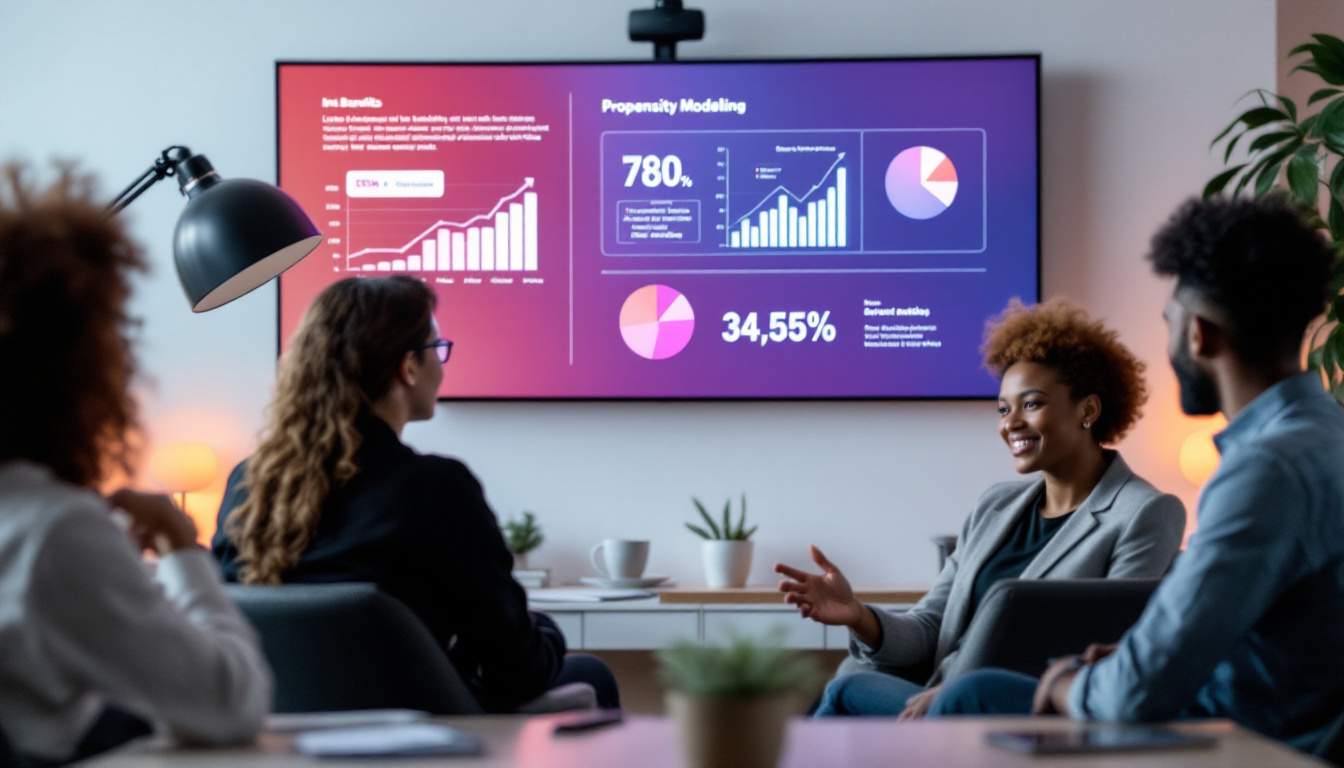
Another benefit of propensity modeling is the ability to personalize marketing messages. By understanding the behaviors and preferences of individual customers, marketers can tailor their messages to resonate more deeply with their audience. This can lead to higher engagement rates, increased customer loyalty, and ultimately, higher sales.
Improved Targeting
One of the key benefits of propensity modeling is the ability to improve targeting. By understanding the likelihood of different outcomes, marketers can focus their resources on the individuals most likely to respond positively. This not only improves the effectiveness of marketing campaigns, but also helps to reduce wasted resources.
For example, a company might use a purchase propensity model to identify customers who are most likely to make a purchase in the near future. They could then target these customers with special offers or personalized messages, increasing the likelihood of a sale.
Personalized Marketing
Another key benefit of propensity modeling is the ability to personalize marketing messages. By understanding the behaviors and preferences of individual customers, marketers can tailor their messages to resonate more deeply with their audience. This can lead to higher engagement rates, increased customer loyalty, and ultimately, higher sales.
For example, a company might use a response propensity model to identify customers who are most likely to respond to a particular marketing campaign. They could then tailor the campaign to these customers, increasing the likelihood of a positive response.
Challenges of Propensity Modeling
While propensity modeling offers many benefits, it also presents a number of challenges. One of the most significant is the need for large amounts of data. In order to build accurate models, marketers need access to a wide range of data, including demographic information, past purchase history, browsing behavior, and more.
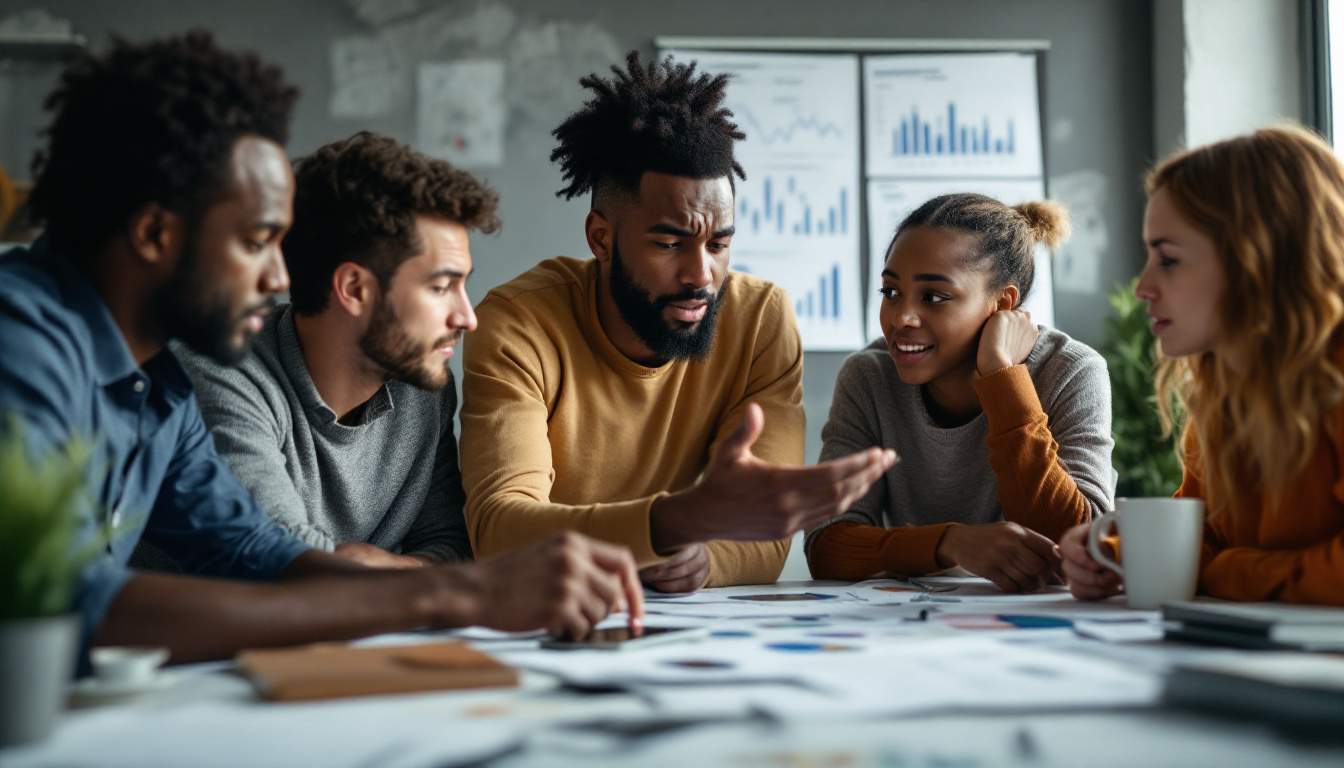
Another challenge is the complexity of the modeling process. Building propensity models requires a deep understanding of statistical algorithms and data analysis techniques. This can be a barrier for companies that do not have the necessary expertise in-house.
Data Requirements
One of the key challenges of propensity modeling is the need for large amounts of data. In order to build accurate models, marketers need access to a wide range of data, including demographic information, past purchase history, browsing behavior, and more.
Collecting this data can be a significant undertaking, particularly for smaller businesses. It requires a robust data collection infrastructure, as well as strict data privacy practices to ensure compliance with regulations.
Modeling Complexity
Another challenge of propensity modeling is the complexity of the modeling process. Building propensity models requires a deep understanding of statistical algorithms and data analysis techniques. This can be a barrier for companies that do not have the necessary expertise in-house.
Even with the necessary expertise, building accurate models can be a time-consuming process. It requires careful data preparation, model selection, and validation to ensure the accuracy of the predictions.
Future of Propensity Modeling
Despite the challenges, the future of propensity modeling looks bright. Advances in technology are making it easier than ever to collect and analyze large amounts of data, making propensity models more accurate and accessible.
Furthermore, the rise of machine learning and artificial intelligence is opening up new possibilities for propensity modeling. These technologies can automate much of the modeling process, making it faster and more efficient. They can also uncover complex patterns and relationships in the data that would be difficult to detect using traditional methods.
Technological Advances
Advances in technology are making propensity modeling more accessible than ever. Modern data collection tools make it easier to gather the large amounts of data needed for propensity models. At the same time, advances in data storage and processing technology make it possible to handle this data more efficiently.
Furthermore, the rise of cloud computing has made it possible for even small businesses to access the computational power needed for propensity modeling. This has leveled the playing field, allowing businesses of all sizes to take advantage of this powerful tool.
Machine Learning and AI
The rise of machine learning and artificial intelligence is opening up new possibilities for propensity modeling. These technologies can automate much of the modeling process, making it faster and more efficient. They can also uncover complex patterns and relationships in the data that would be difficult to detect using traditional methods.
For example, machine learning algorithms can be used to automatically select the most relevant features for a propensity model, reducing the time and effort required for data preparation. They can also be used to automatically tune the model parameters, improving the accuracy of the predictions.
Conclusion
In conclusion, propensity modeling is a powerful tool for modern marketers. It provides a way to predict customer behavior, enabling marketers to target their efforts more effectively and efficiently. While it does present some challenges, advances in technology and the rise of machine learning and AI are making it more accessible than ever.
As the field of marketing continues to evolve, propensity modeling will likely play an increasingly important role. By understanding the likelihood of different outcomes, marketers can tailor their strategies to the individuals most likely to respond positively, maximizing their return on investment and driving business growth.