What is a product recommendation engine?
The term ‘product recommendation engine’ refers to a system that uses data analysis techniques to suggest products or services that a customer might be interested in. This technology has become increasingly prevalent in the digital age, where businesses have access to vast amounts of data about their customers’ preferences and behaviors.
Product recommendation engines are a key component of personalized marketing strategies, which aim to tailor the customer experience to individual needs and preferences. By providing personalized product recommendations, businesses can increase customer engagement, boost sales, and build customer loyalty.
How Product Recommendation Engines Work
Product recommendation engines work by analyzing data about customers’ past behavior, such as their browsing history, purchase history, and ratings or reviews they’ve given. This data is then used to predict what products a customer might be interested in, and these products are recommended to the customer.
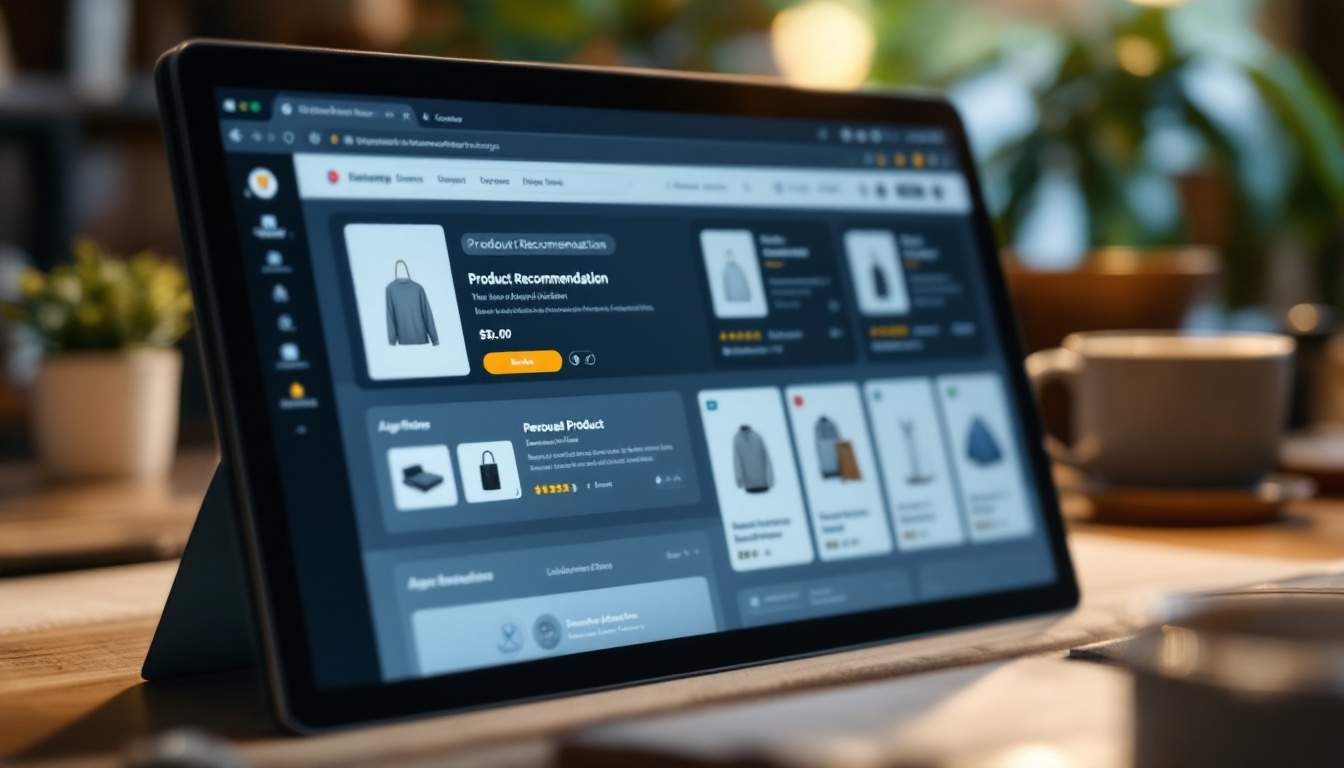
The specific techniques used by product recommendation engines can vary, but they typically involve some form of machine learning or artificial intelligence. These technologies are capable of identifying patterns in large datasets and making predictions based on these patterns.
Types of Data Used
There are several types of data that can be used by a product recommendation engine. The most common is transactional data, which includes information about a customer’s past purchases. This can provide insights into what types of products a customer is likely to be interested in.
Another type of data that can be used is behavioral data, which includes information about a customer’s interactions with a website or app. This can include things like what pages a customer visits, how long they spend on each page, and what items they add to their shopping cart.
Algorithms Used
The algorithms used by product recommendation engines can be broadly divided into two categories: collaborative filtering and content-based filtering. Collaborative filtering algorithms make recommendations based on the behavior of similar customers, while content-based filtering algorithms make recommendations based on the characteristics of the products themselves.
Many product recommendation engines also use hybrid algorithms, which combine elements of both collaborative filtering and content-based filtering. These algorithms can provide more accurate recommendations by taking into account both the behavior of similar customers and the characteristics of the products.
Benefits of Product Recommendation Engines
Product recommendation engines offer a number of benefits for businesses. One of the most significant is the ability to provide personalized recommendations, which can enhance the customer experience and increase customer satisfaction.
By recommending products that are tailored to a customer’s individual preferences and needs, businesses can make the shopping process more convenient and enjoyable for the customer. This can lead to increased customer loyalty and repeat purchases.
Increased Sales
Product recommendation engines can also help to increase sales by encouraging customers to purchase additional products. By recommending products that are related to those a customer has already shown an interest in, businesses can increase the likelihood that the customer will make additional purchases.
Furthermore, by recommending products that a customer might not have discovered on their own, businesses can introduce customers to new products and increase the overall amount of sales.
Improved Customer Engagement
Product recommendation engines can also improve customer engagement by providing a more interactive and personalized shopping experience. By presenting customers with products that are relevant to their interests, businesses can keep customers engaged and encourage them to spend more time on their website or app.
Additionally, by providing personalized recommendations, businesses can make customers feel valued and understood, which can increase customer satisfaction and loyalty.
Challenges and Limitations of Product Recommendation Engines
While product recommendation engines offer many benefits, they also come with some challenges and limitations. One of the main challenges is the need for large amounts of data. In order to make accurate recommendations, product recommendation engines need access to detailed data about customers’ behavior and preferences.
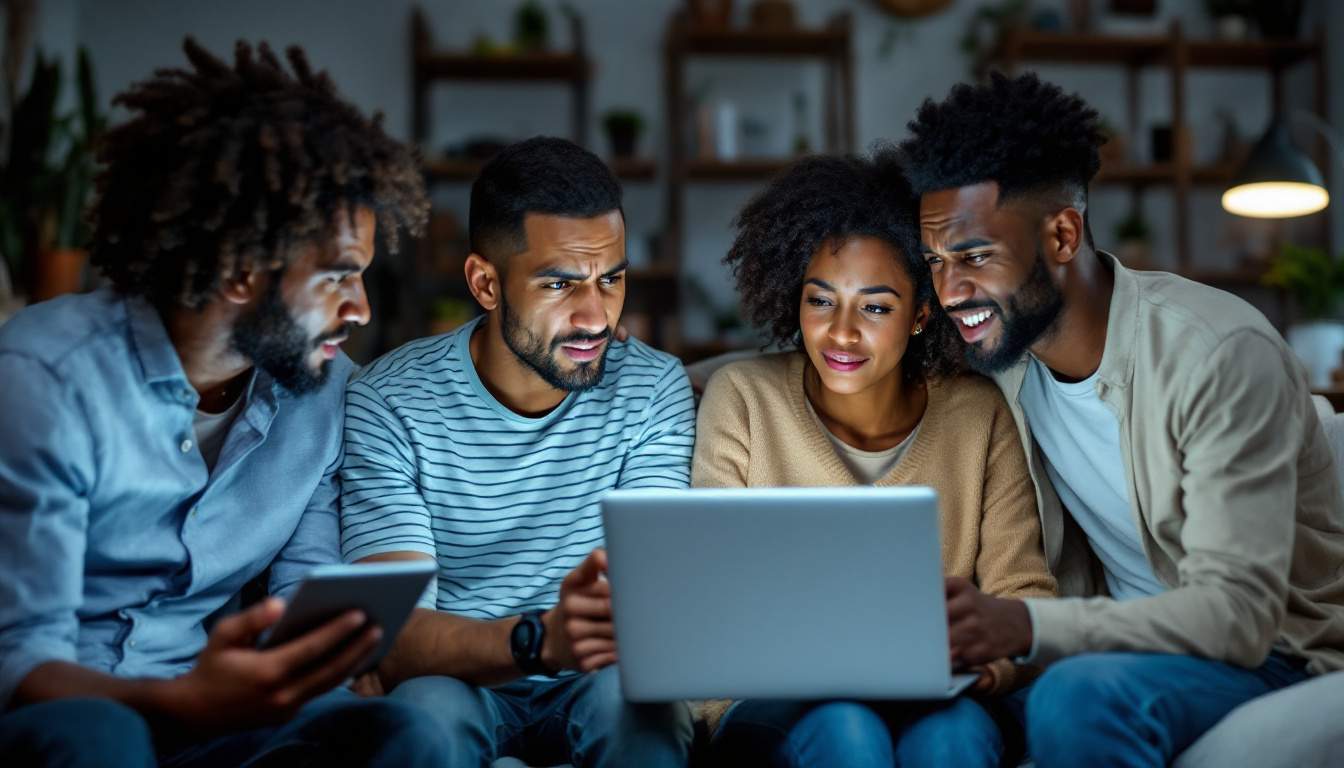
Another challenge is the complexity of the algorithms used by product recommendation engines. These algorithms need to be able to handle large datasets and make accurate predictions, which requires advanced technical skills and resources.
Data Privacy Concerns
One of the main limitations of product recommendation engines is the potential for data privacy concerns. In order to make personalized recommendations, product recommendation engines need access to detailed data about customers’ behavior and preferences. This can raise concerns about data privacy and security, particularly if the data is not handled properly.
Businesses need to ensure that they are complying with all relevant data privacy laws and regulations, and that they are taking appropriate measures to protect their customers’ data. This can include things like encrypting data, using secure servers, and obtaining customers’ consent before collecting their data.
Accuracy of Recommendations
Another limitation of product recommendation engines is the potential for inaccurate recommendations. While these systems are designed to make accurate predictions about what products a customer might be interested in, they are not always 100% accurate.
This can be due to a variety of factors, including the quality of the data used, the complexity of the algorithms, and the unpredictability of human behavior. Businesses need to continually monitor and adjust their product recommendation engines to ensure that they are providing accurate and relevant recommendations.
Future of Product Recommendation Engines
The future of product recommendation engines looks promising, with advancements in technology and data analysis techniques opening up new possibilities for personalized marketing. One of the main trends in this area is the increasing use of artificial intelligence and machine learning in product recommendation engines.
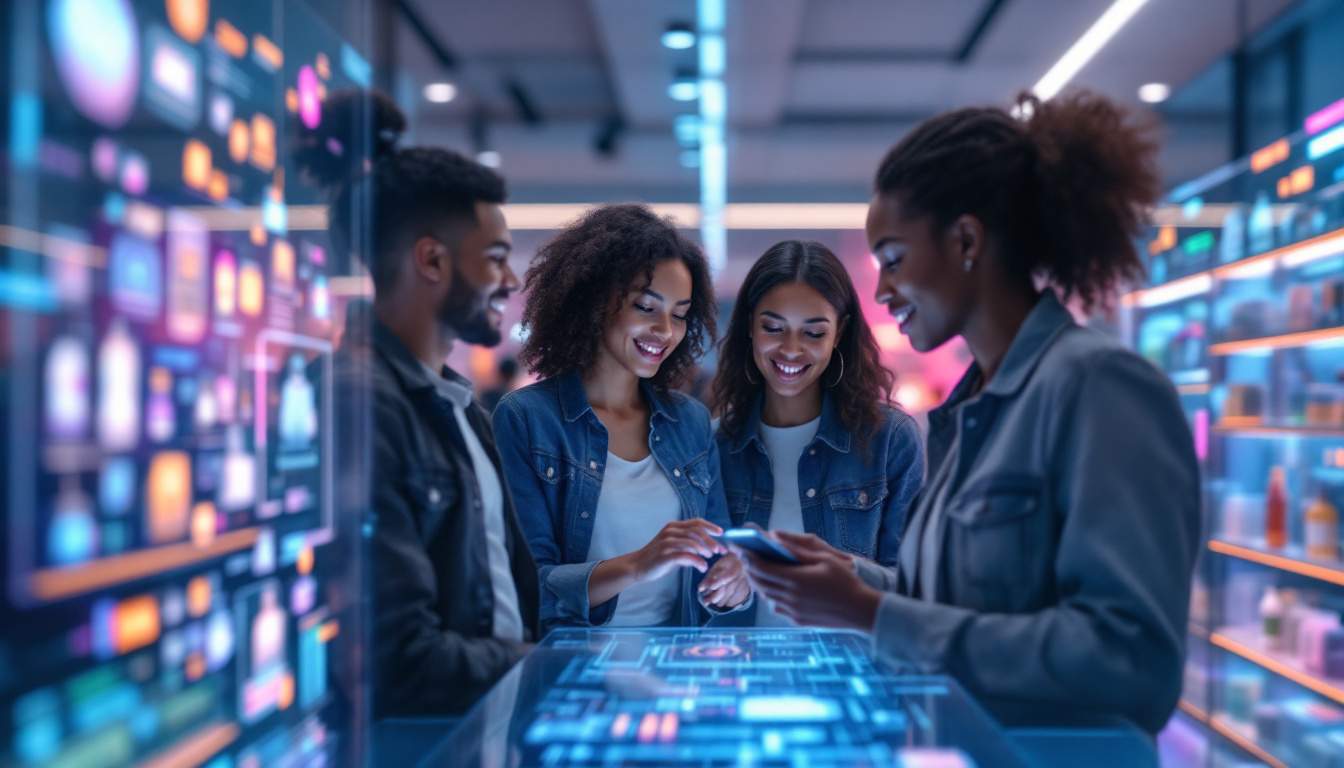
These technologies can analyze large amounts of data more quickly and accurately than traditional methods, and can identify complex patterns and relationships that might not be apparent to human analysts. This can lead to more accurate and personalized product recommendations.
Integration with Other Technologies
Another trend in the field of product recommendation engines is the integration with other technologies, such as virtual reality and augmented reality. These technologies can provide a more immersive and interactive shopping experience, and can be used in conjunction with product recommendation engines to provide personalized product recommendations in real-time.
For example, a customer could use a virtual reality headset to browse a virtual store, and the product recommendation engine could suggest products based on the customer’s interactions with the virtual environment.
Increased Use of Big Data
The increasing availability of big data is also likely to have a significant impact on the future of product recommendation engines. Big data refers to extremely large datasets that can be analyzed to reveal patterns, trends, and associations, particularly relating to human behavior and interactions.
By analyzing big data, product recommendation engines can gain deeper insights into customers’ behavior and preferences, and can make more accurate and personalized product recommendations. This can lead to improved customer satisfaction, increased sales, and a competitive advantage for businesses.