What is predictive lead scoring?
Predictive lead scoring is a marketing technique that uses statistical algorithms and machine learning to predict which leads are most likely to convert into customers. This method provides marketers with a quantitative way to prioritize leads, helping them to focus their efforts on the most promising prospects and improve their conversion rates.
Traditionally, lead scoring was a manual process, based on a marketer’s intuition and experience. However, with the advent of big data and advanced analytics, predictive lead scoring has emerged as a more efficient and accurate alternative. This approach leverages historical data and predictive analytics to assign scores to leads, indicating their likelihood of becoming customers.
Understanding Predictive Lead Scoring
Predictive lead scoring is based on the principle that past behavior is a good predictor of future behavior. It uses machine learning algorithms to analyze historical data on leads and customers, identifying patterns and trends that can be used to predict future outcomes. The predictive model is then used to assign scores to new leads, indicating their likelihood of converting into customers.
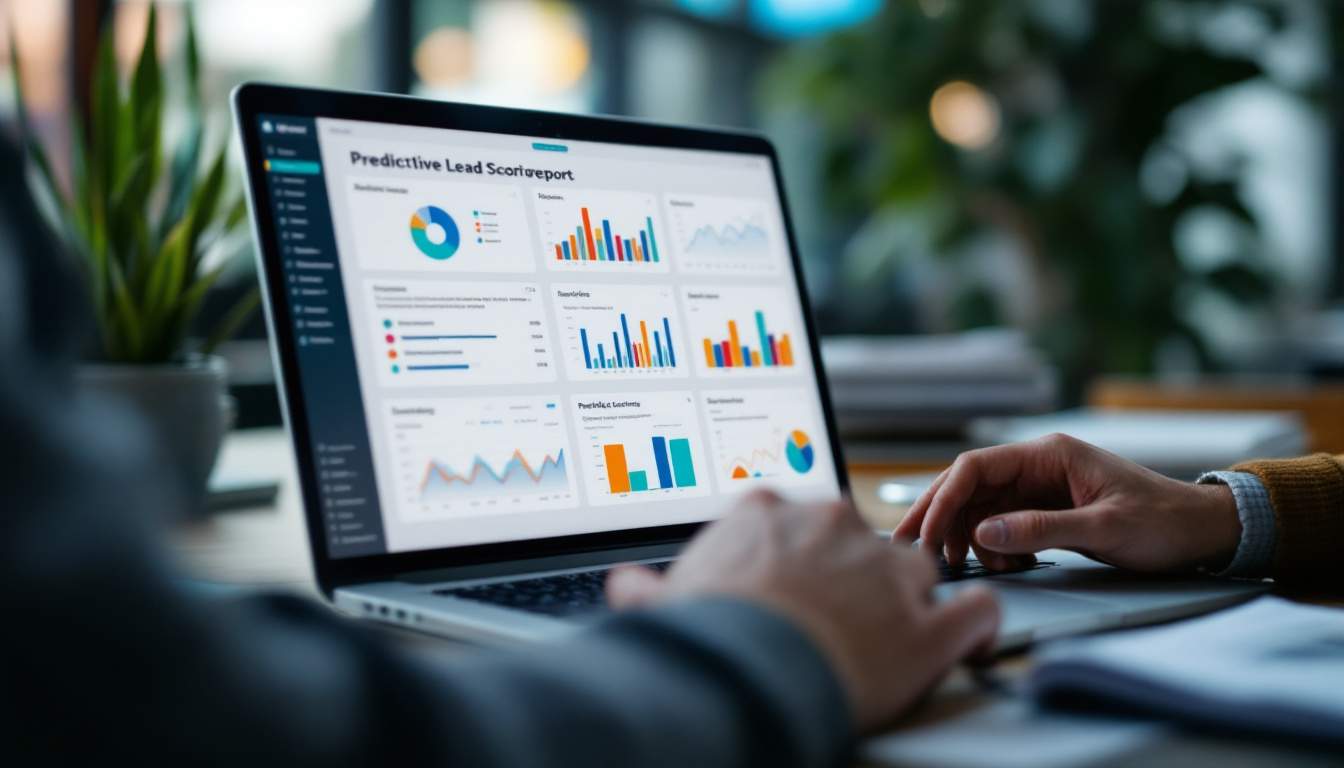
The predictive lead scoring process begins with data collection. Marketers gather data on their leads from various sources, including their website, social media platforms, and customer relationship management (CRM) systems. This data can include demographic information, online behavior, and engagement with marketing campaigns.
Data Analysis in Predictive Lead Scoring
Once the data has been collected, it is analyzed using machine learning algorithms. These algorithms are capable of processing large volumes of data and identifying complex patterns that would be difficult for a human to detect. The algorithms learn from the data, improving their predictions over time.
The result of this analysis is a predictive model, which can be used to assign scores to new leads. The model takes into account a wide range of factors, including the lead’s demographic profile, online behavior, and engagement with marketing campaigns. The score assigned to each lead represents the probability that they will become a customer.
Benefits of Predictive Lead Scoring
Predictive lead scoring offers several benefits over traditional lead scoring methods. Firstly, it provides a quantitative way to prioritize leads, helping marketers to focus their efforts on the most promising prospects. This can improve conversion rates and increase the efficiency of marketing campaigns.
Secondly, predictive lead scoring is more accurate than manual lead scoring methods. Because it uses machine learning algorithms to analyze data, it can identify patterns and trends that a human might miss. This leads to more accurate predictions and better decision-making.
Implementing Predictive Lead Scoring
Implementing predictive lead scoring requires a combination of data, technology, and expertise. Marketers need access to a large volume of high-quality data, a machine learning platform to analyze the data, and the skills to interpret the results and apply them to their marketing strategies.
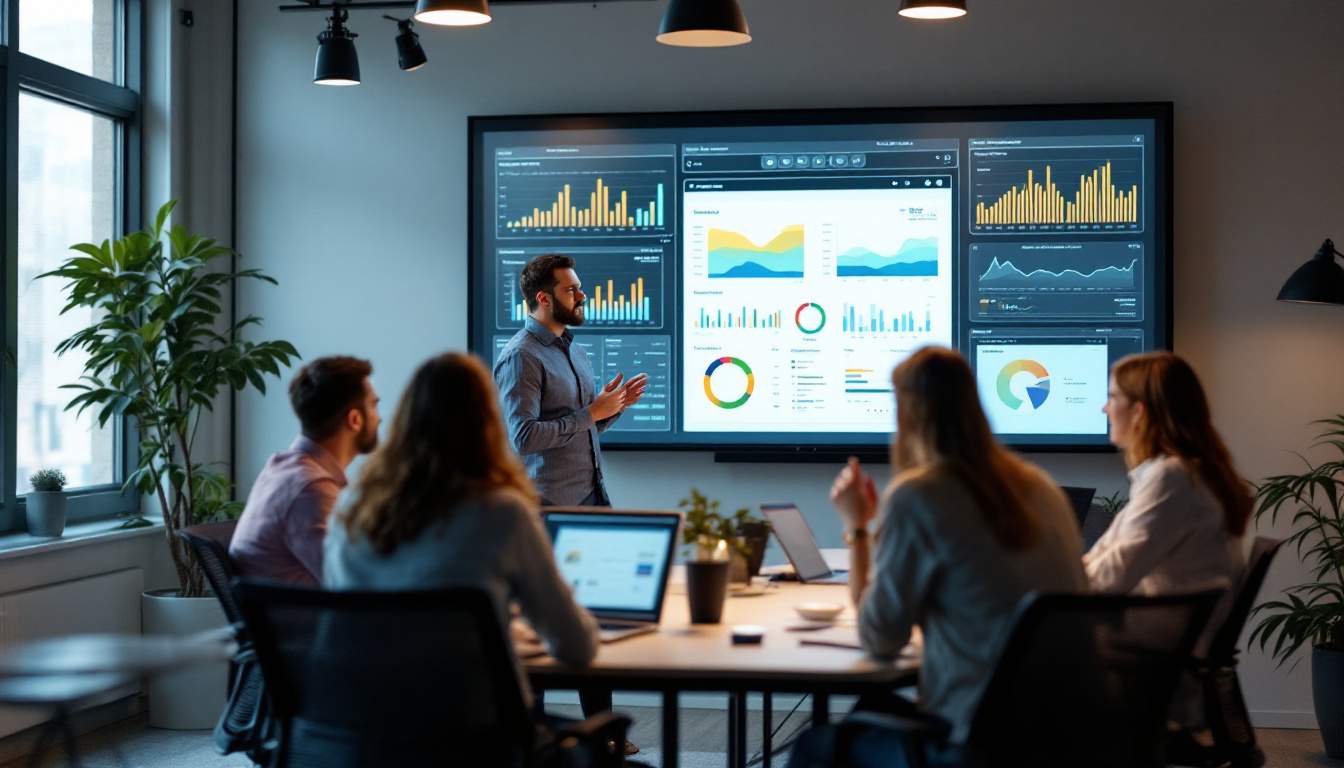
The first step in implementing predictive lead scoring is data collection. Marketers need to gather data on their leads from a variety of sources, including their website, social media platforms, and CRM systems. This data should include demographic information, online behavior, and engagement with marketing campaigns.
Data Preparation for Predictive Lead Scoring
Once the data has been collected, it needs to be prepared for analysis. This involves cleaning the data to remove errors and inconsistencies, and transforming it into a format that can be processed by the machine learning algorithms. This is a crucial step in the process, as the quality of the data can significantly impact the accuracy of the predictive model.
After the data has been prepared, it is divided into a training set and a test set. The training set is used to train the machine learning algorithms, while the test set is used to evaluate the performance of the predictive model.
Model Training and Evaluation
The next step is to train the machine learning algorithms using the training set. The algorithms learn from the data, identifying patterns and trends that can be used to predict future outcomes. This process is iterative, with the algorithms continually improving their predictions as they learn from the data.
Once the algorithms have been trained, the predictive model is evaluated using the test set. This involves comparing the model’s predictions with the actual outcomes, to assess the accuracy of the model. If the model’s performance is satisfactory, it can be used to assign scores to new leads. If not, the model may need to be refined or retrained.
Using Predictive Lead Scoring in Marketing Strategies
Predictive lead scoring can be integrated into a marketing strategy in several ways. One common approach is to use the lead scores to prioritize leads for follow-up. Leads with high scores are considered to be high-priority, and are followed up first. This helps to ensure that the most promising leads are not overlooked, and can improve conversion rates.
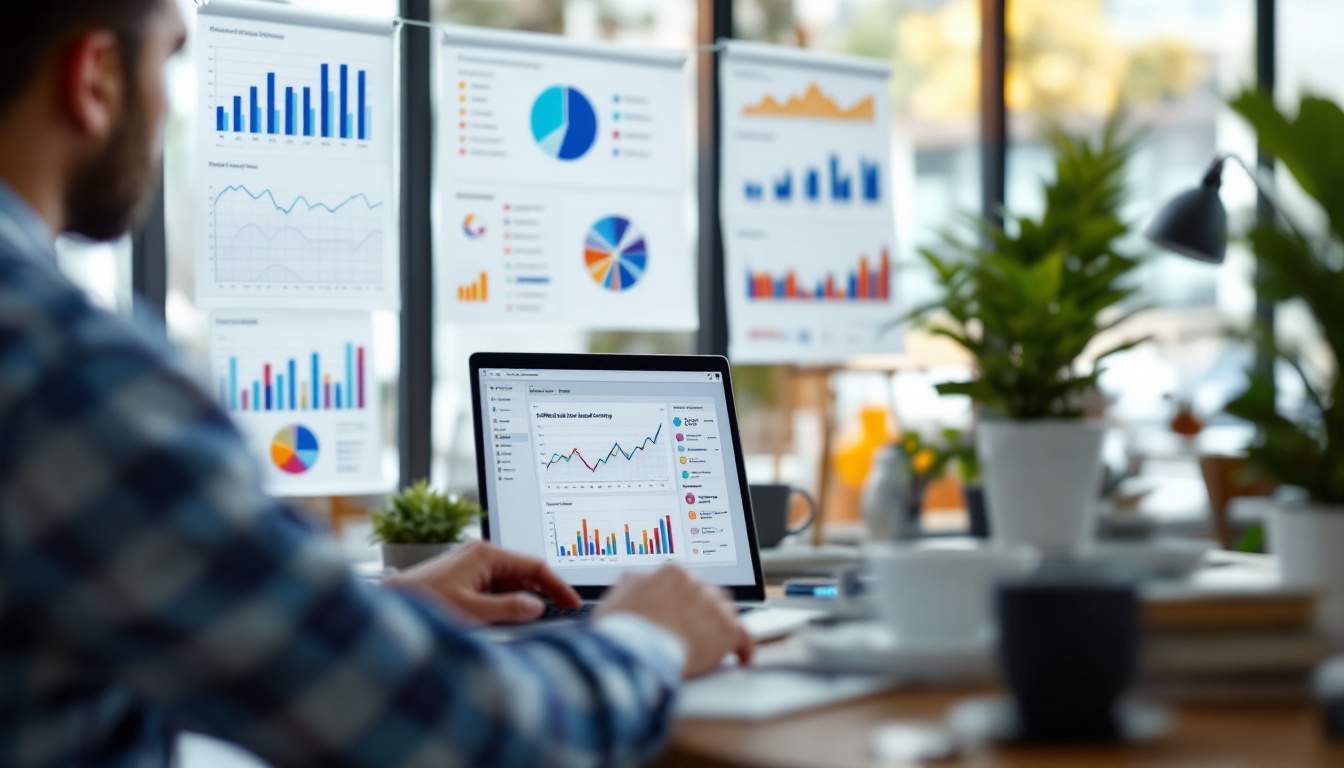
Another approach is to use the lead scores to segment leads for targeted marketing campaigns. For example, a company might target a marketing campaign at leads with a particular demographic profile, or at leads who have shown a high level of engagement with previous marketing campaigns. By targeting their marketing efforts in this way, companies can improve the effectiveness of their campaigns and increase their return on investment.
Challenges in Using Predictive Lead Scoring
While predictive lead scoring offers many benefits, it also presents some challenges. One of the main challenges is the need for high-quality data. If the data used to train the predictive model is inaccurate or incomplete, the model’s predictions may be unreliable. Therefore, it is crucial to ensure that the data is clean and comprehensive before it is used for predictive lead scoring.
Another challenge is the need for expertise in data analysis and machine learning. Predictive lead scoring involves complex algorithms and statistical techniques, and requires a deep understanding of these methods to implement effectively. Therefore, companies may need to invest in training or hire specialists to implement predictive lead scoring.
Future of Predictive Lead Scoring
The future of predictive lead scoring looks promising, with advances in technology and analytics making it increasingly accessible and effective. As machine learning algorithms become more sophisticated, they are likely to become even better at predicting which leads will convert into customers.
Furthermore, as more companies adopt predictive lead scoring, it is likely to become a standard practice in marketing. This will drive further innovation in the field, leading to even more effective and efficient lead scoring methods.