What is predictive customer analytics?
Predictive customer analytics refers to the use of data, statistical algorithms, and machine learning techniques to identify the likelihood of future outcomes based on historical data. The goal is to go beyond knowing what has happened to providing a best assessment of what will happen in the future. This article will delve into the depths of predictive customer analytics, exploring its definition, importance, applications, benefits, challenges, and future trends.
Definition of Predictive Customer Analytics
Predictive customer analytics is a branch of advanced analytics that involves the use of data, statistical algorithms, and machine learning techniques to predict future events. At its core, predictive analytics revolves around the idea of using past data to predict future outcomes. It involves many techniques from data mining, statistics, modeling, machine learning, and artificial intelligence to analyze current data and make predictions about the future.
This type of analytics can be used in many industries to predict and strategize for any number of future outcomes. In the context of marketing, predictive customer analytics is used to analyze customer behavior, trends, and decisions, and predict future buying patterns. This can help businesses to anticipate customer needs and preferences, and tailor their strategies accordingly.
Importance of Predictive Customer Analytics
In today’s highly competitive business environment, predictive customer analytics has become an essential tool for companies to stay ahead of the competition. By predicting future customer behavior, businesses can make proactive, data-driven decisions that can lead to improved customer satisfaction, increased revenue, and business growth.
Moreover, predictive customer analytics allows businesses to identify potential risks and opportunities, optimize marketing campaigns, and improve overall business performance. It can also help businesses to understand the impact of different factors on customer behavior, and make strategic decisions based on these insights.
Improving Customer Experience
One of the key benefits of predictive customer analytics is the ability to improve customer experience. By predicting customer behavior, businesses can anticipate customer needs and preferences, and provide personalized experiences that meet these expectations. This can lead to increased customer satisfaction, loyalty, and retention.
For example, a retail company can use predictive analytics to anticipate the products that a customer is likely to buy in the future, and provide personalized product recommendations based on these predictions. This can enhance the shopping experience for the customer, and increase the likelihood of repeat purchases.
Increasing Revenue
Predictive customer analytics can also help businesses to increase revenue. By predicting future buying patterns, businesses can optimize their marketing strategies to target the right customers with the right products at the right time. This can lead to increased sales, revenue, and profitability.
For instance, a telecom company can use predictive analytics to predict the likelihood of a customer churn, and take proactive measures to retain the customer. This can reduce customer churn rate, and increase customer lifetime value.
Applications of Predictive Customer Analytics
Predictive customer analytics has a wide range of applications in the field of marketing. It can be used to predict customer behavior, optimize marketing campaigns, personalize customer experiences, and much more. Here are some of the key applications of predictive customer analytics in marketing.
Firstly, predictive customer analytics can be used to predict customer behavior. This can help businesses to anticipate customer needs and preferences, and tailor their strategies accordingly. For example, a retail company can use predictive analytics to anticipate the products that a customer is likely to buy in the future, and provide personalized product recommendations based on these predictions.
Optimizing Marketing Campaigns
Predictive customer analytics can also be used to optimize marketing campaigns. By predicting the likelihood of customer response to different marketing strategies, businesses can optimize their marketing campaigns to target the right customers with the right messages at the right time. This can lead to improved marketing effectiveness, and increased return on investment (ROI).
For instance, a telecom company can use predictive analytics to predict the likelihood of a customer responding to a particular marketing campaign, and tailor the campaign accordingly. This can increase the effectiveness of the campaign, and reduce marketing costs.
Personalizing Customer Experiences
Another key application of predictive customer analytics is in personalizing customer experiences. By predicting customer behavior, businesses can provide personalized experiences that meet customer expectations. This can lead to increased customer satisfaction, loyalty, and retention.
For example, a hotel chain can use predictive analytics to anticipate the preferences of a guest, and provide personalized services based on these predictions. This can enhance the guest experience, and increase the likelihood of repeat visits.
Benefits of Predictive Customer Analytics
Predictive customer analytics offers a multitude of benefits to businesses. It allows businesses to make proactive, data-driven decisions, improve customer experience, increase revenue, and much more. Here are some of the key benefits of predictive customer analytics.
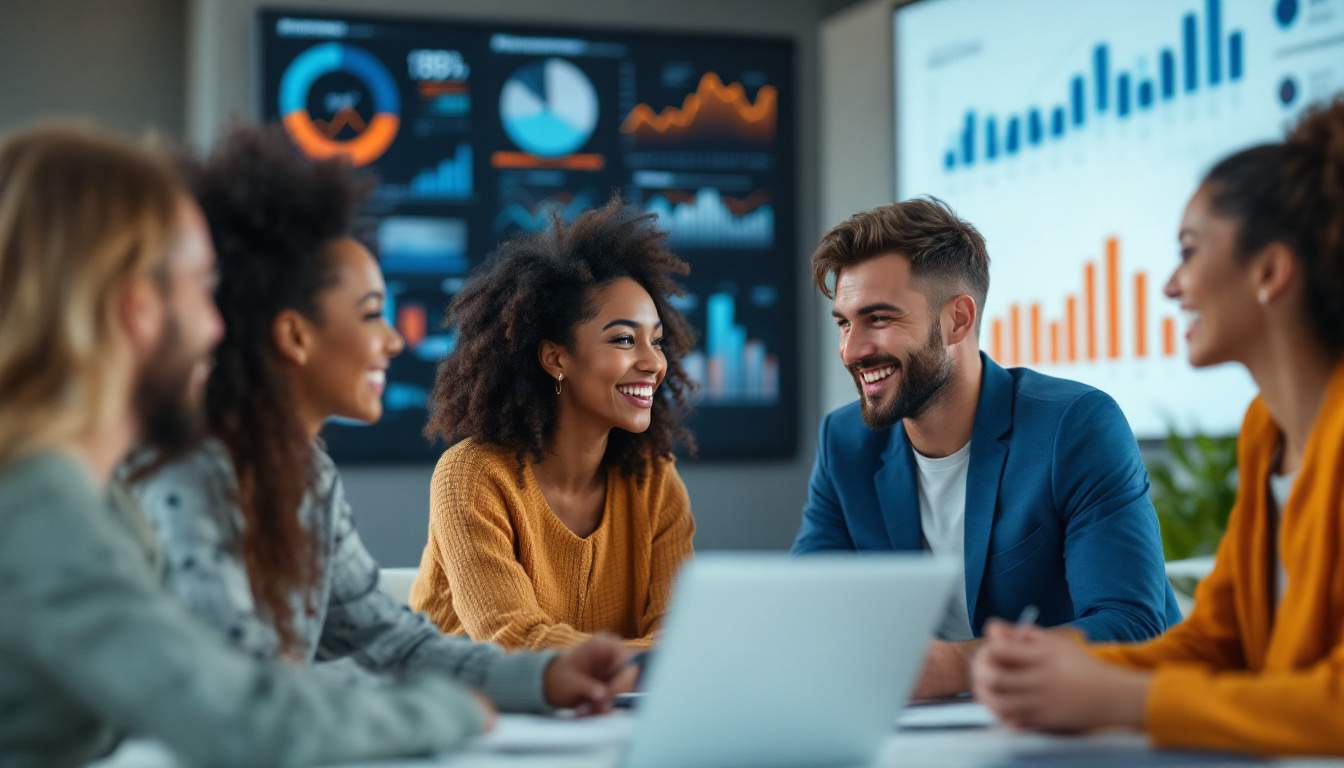
Firstly, predictive customer analytics allows businesses to make proactive decisions. By predicting future outcomes, businesses can make proactive, data-driven decisions that can lead to improved business performance. For example, a retail company can use predictive analytics to anticipate future sales trends, and adjust their inventory levels accordingly.
Improving Customer Experience
As mentioned earlier, one of the key benefits of predictive customer analytics is the ability to improve customer experience. By predicting customer behavior, businesses can anticipate customer needs and preferences, and provide personalized experiences that meet these expectations. This can lead to increased customer satisfaction, loyalty, and retention.
For instance, a telecom company can use predictive analytics to anticipate the needs of a customer, and provide personalized services based on these predictions. This can enhance the customer experience, and increase customer loyalty.
Increasing Revenue
Another major benefit of predictive customer analytics is the potential to increase revenue. By predicting future buying patterns, businesses can optimize their marketing strategies to target the right customers with the right products at the right time. This can lead to increased sales, revenue, and profitability.
For example, a hotel chain can use predictive analytics to anticipate the likelihood of a guest booking a room, and adjust their pricing strategy accordingly. This can increase room bookings, and boost revenue.
Challenges of Predictive Customer Analytics
While predictive customer analytics offers numerous benefits, it also comes with its own set of challenges. These include data quality issues, lack of skilled personnel, privacy concerns, and more. Here are some of the key challenges of predictive customer analytics.
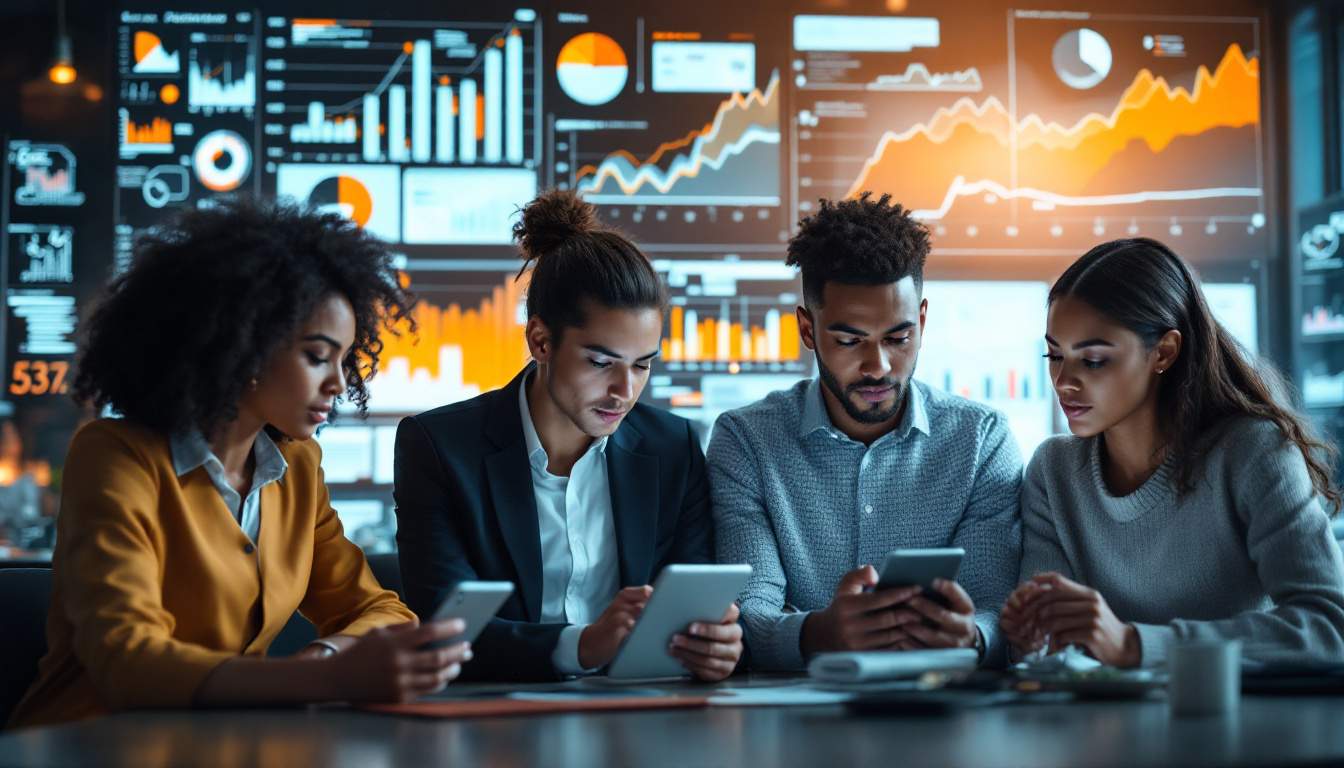
Firstly, data quality is a major challenge in predictive customer analytics. For predictive analytics to be effective, it requires high-quality, accurate, and relevant data. However, many businesses struggle with data quality issues, such as incomplete or inaccurate data, which can affect the accuracy of predictions.
Lack of Skilled Personnel
Another challenge in predictive customer analytics is the lack of skilled personnel. Predictive analytics requires a certain level of expertise in data analysis, statistics, and machine learning. However, many businesses lack the necessary skills and expertise to effectively implement and use predictive analytics.
For instance, a retail company may have access to a wealth of customer data, but lack the necessary skills to analyze this data and make accurate predictions. This can limit the effectiveness of predictive customer analytics.
Privacy Concerns
Privacy concerns are another major challenge in predictive customer analytics. With the increasing use of customer data in predictive analytics, there are growing concerns about data privacy and security. Businesses need to ensure that they are complying with data privacy regulations, and that customer data is securely stored and handled.
For example, a telecom company using predictive analytics needs to ensure that customer data is securely stored and handled, and that it is complying with data privacy regulations. Failure to do so can lead to legal issues, and damage the company’s reputation.
Future Trends in Predictive Customer Analytics
The field of predictive customer analytics is constantly evolving, with new trends and technologies emerging all the time. Here are some of the key future trends in predictive customer analytics.
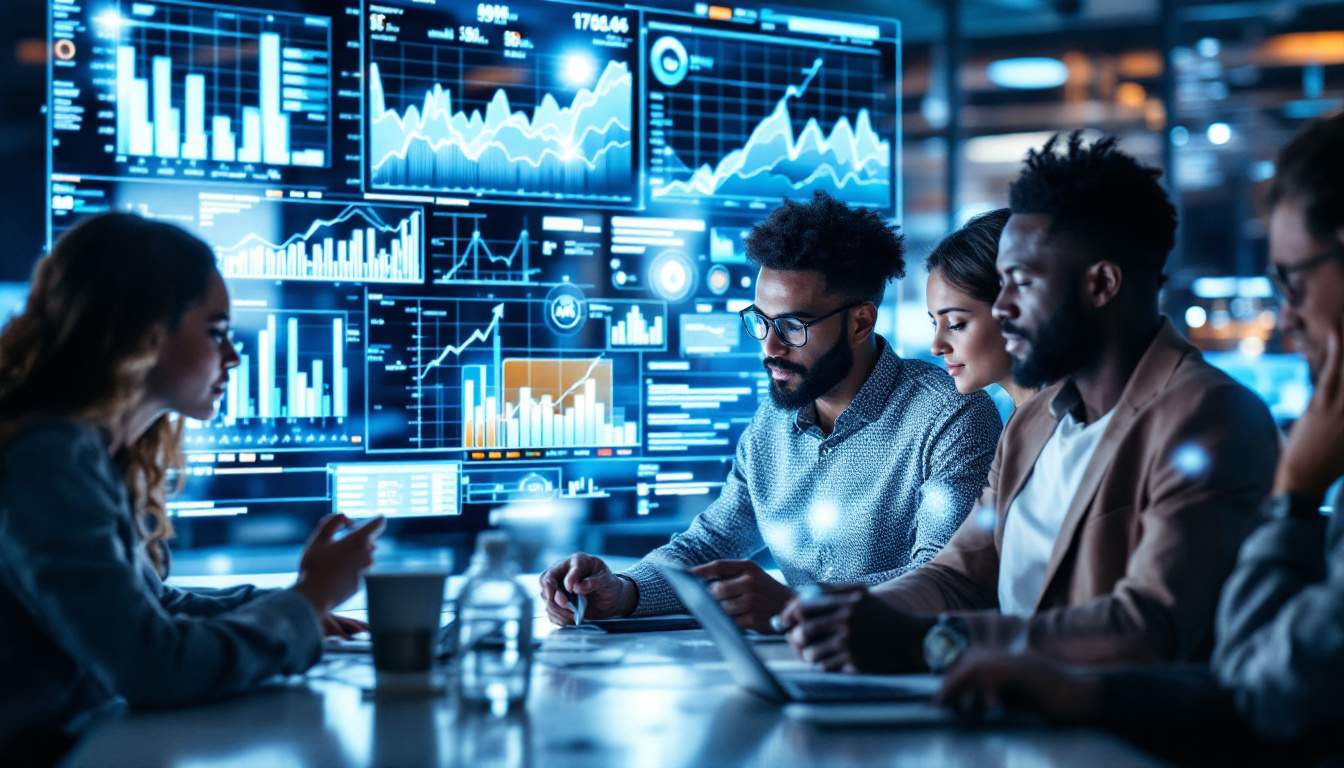
Firstly, the use of artificial intelligence (AI) and machine learning in predictive customer analytics is expected to increase in the future. These technologies can help to analyze large volumes of data, and make accurate predictions at a much faster rate than traditional methods.
Real-Time Predictive Analytics
Another key trend is the move towards real-time predictive analytics. With the increasing availability of real-time data, businesses are looking to make predictions in real-time, to respond to customer needs and preferences as they arise. This can lead to improved customer experience, and increased business performance.
For instance, a retail company can use real-time predictive analytics to anticipate the needs of a customer as they are shopping, and provide personalized product recommendations based on these predictions. This can enhance the shopping experience for the customer, and increase the likelihood of a purchase.
Increased Use of Predictive Analytics in Marketing
Finally, the use of predictive analytics in marketing is expected to increase in the future. With the increasing availability of customer data, businesses are looking to use predictive analytics to optimize their marketing strategies, and target the right customers with the right messages at the right time.
For example, a telecom company can use predictive analytics to predict the likelihood of a customer responding to a particular marketing campaign, and tailor the campaign accordingly. This can increase the effectiveness of the campaign, and reduce marketing costs.