What is predictive churn modeling?
Predictive churn modeling uses advanced analytics and machine learning to predict customer behavior, specifically, their likelihood to stop doing business with a company. This concept is crucial for businesses to understand as it helps them identify and retain customers who are at risk of churning, thereby improving customer retention and increasing profitability.
The process involves analyzing historical customer data, identifying patterns and trends, and using this information to predict future customer behavior. By understanding which customers are likely to churn, businesses can take proactive steps to retain these customers, such as offering personalized incentives or improving customer service.
The Importance of Predictive Churn Modeling
Predictive churn modeling plays a significant role in customer retention strategies. By identifying customers who are likely to churn, businesses can focus their retention efforts on these customers, thereby reducing churn rate and increasing customer lifetime value. This not only helps businesses save on customer acquisition costs but also improves their overall profitability.
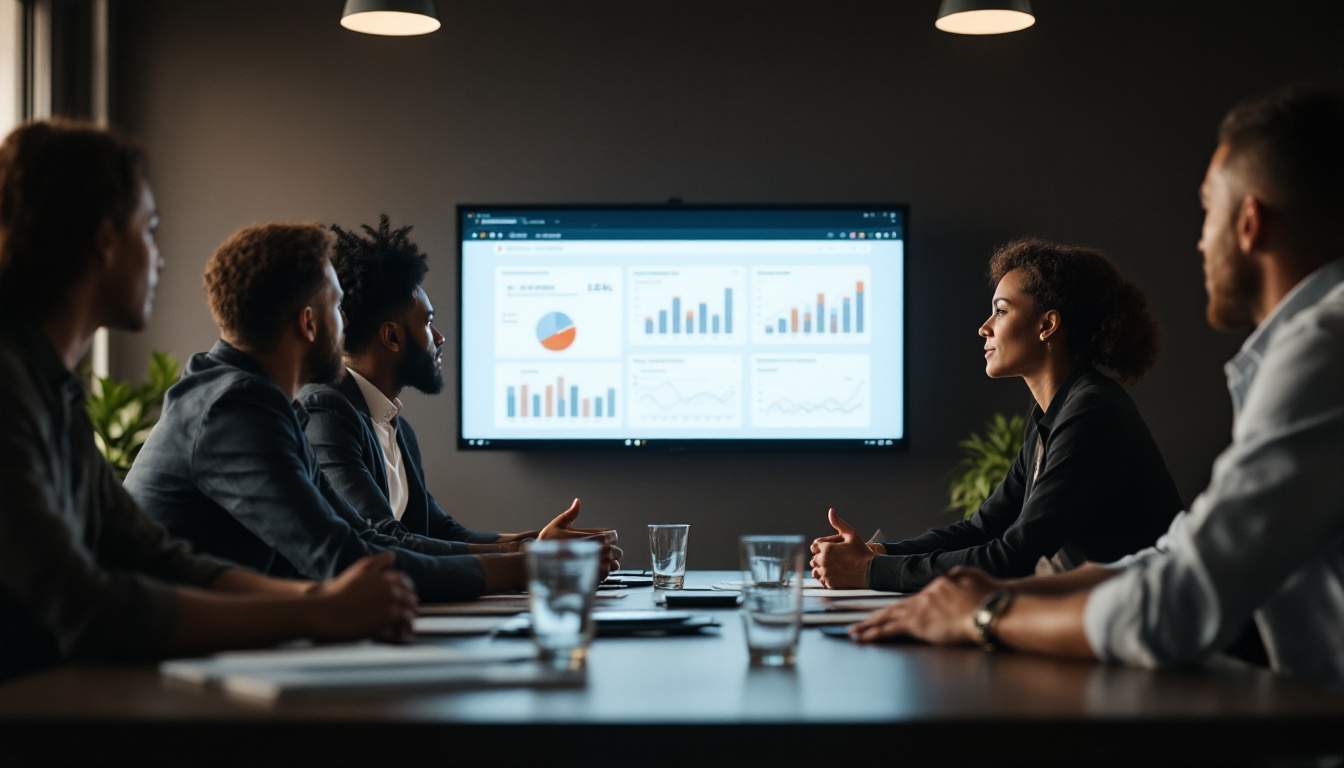
Moreover, predictive churn modeling provides businesses with valuable insights into customer behavior. By understanding why customers churn, businesses can identify areas of improvement in their products or services and make necessary changes to enhance customer satisfaction and loyalty.
Cost Efficiency
Acquiring new customers is often more expensive than retaining existing ones. By using predictive churn modeling to identify customers at risk of churning, businesses can focus their marketing efforts on these customers, thereby reducing customer acquisition costs.
Additionally, by understanding the reasons behind customer churn, businesses can address these issues and prevent future churn, further reducing costs and improving profitability.
Customer Satisfaction and Loyalty
Predictive churn modeling can also help businesses improve customer satisfaction and loyalty. By identifying the reasons behind customer churn, businesses can address these issues and enhance their products or services to meet customer needs and expectations.
Furthermore, by taking proactive steps to retain customers at risk of churning, businesses can show these customers that they value their business, thereby improving customer satisfaction and loyalty.
How Predictive Churn Modeling Works
Predictive churn modeling involves several steps, including data collection, data preprocessing, feature selection, model training, and model evaluation. Each of these steps plays a crucial role in the predictive churn modeling process and contributes to the accuracy of the predictions.
It’s important to note that predictive churn modeling is not a one-size-fits-all solution. The process may vary depending on the specific needs and circumstances of the business. However, the general steps involved in predictive churn modeling are as follows.
Data Collection
The first step in predictive churn modeling is data collection. This involves gathering historical customer data, such as purchase history, customer demographics, and customer behavior. The quality and quantity of the data collected can significantly impact the accuracy of the predictions.
It’s also important to collect data over a sufficient period to capture seasonal trends and other patterns in customer behavior. This can help improve the accuracy of the predictive churn model.
Data Preprocessing
Once the data has been collected, the next step is data preprocessing. This involves cleaning the data, handling missing values, and transforming the data into a format suitable for model training. Data preprocessing is a crucial step in the predictive churn modeling process as it can significantly impact the accuracy of the predictions.
Data preprocessing may also involve feature engineering, which is the process of creating new features from the existing data to improve the predictive power of the model.
Feature Selection
Feature selection is the process of selecting the most relevant features for model training. This involves identifying the features that have the most significant impact on customer churn and excluding irrelevant or redundant features.
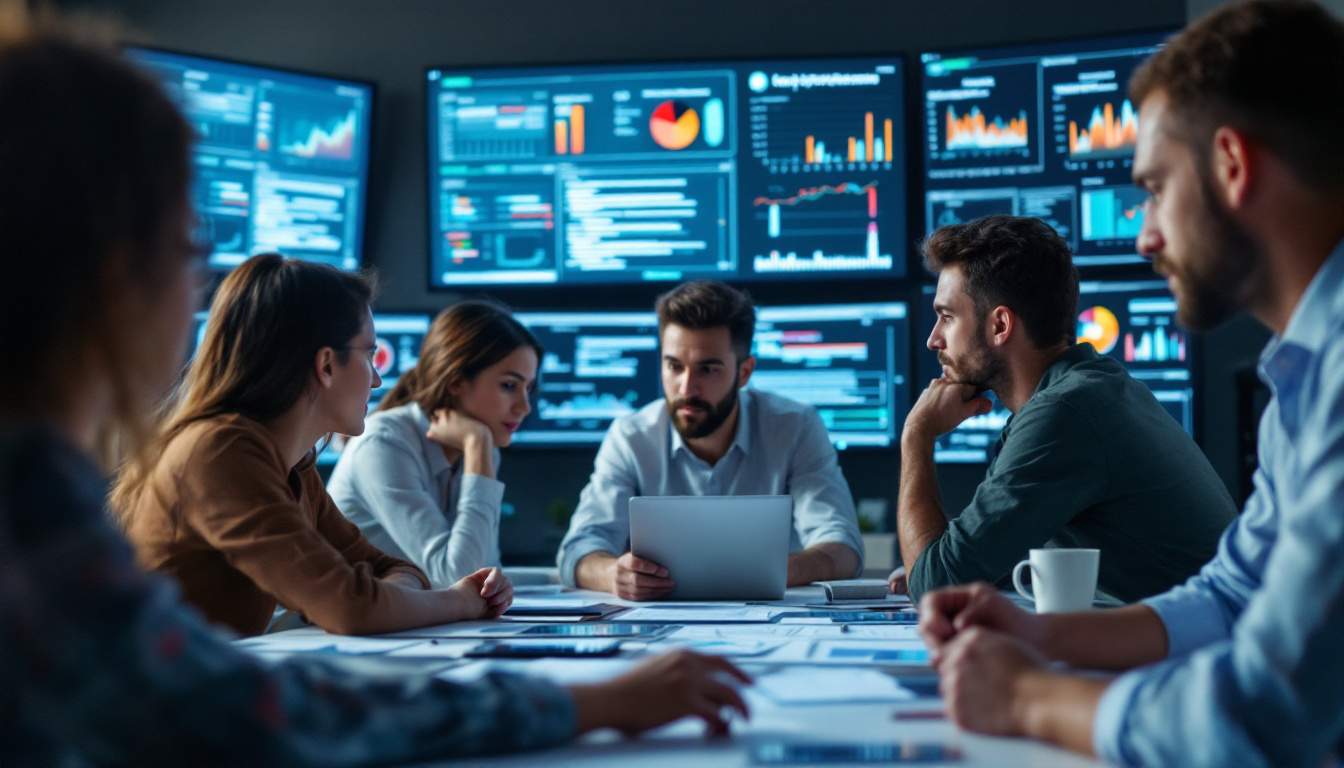
Feature selection is a critical step in the predictive churn modeling process as it can significantly impact the accuracy of the predictions. By selecting the most relevant features, businesses can improve the predictive power of the model and make more accurate predictions.
Model Training
Once the relevant features have been selected, the next step is model training. This involves using the preprocessed data and the selected features to train a predictive churn model. The model is trained using a machine learning algorithm, such as logistic regression, decision trees, or neural networks.
The choice of algorithm depends on the specific needs and circumstances of the business. However, it’s important to choose an algorithm that can handle the complexity of the data and make accurate predictions.
Model Evaluation
The final step in the predictive churn modeling process is model evaluation. This involves testing the model on a separate set of data to evaluate its performance. The model’s performance is typically evaluated using metrics such as accuracy, precision, recall, and the area under the receiver operating characteristic curve (AUC-ROC).
Model evaluation is a crucial step in the predictive churn modeling process as it provides businesses with an understanding of how well the model is likely to perform in a real-world setting. If the model’s performance is not satisfactory, businesses may need to revisit the previous steps and make necessary adjustments.
Challenges in Predictive Churn Modeling
While predictive churn modeling offers numerous benefits, it also presents several challenges. These include data quality issues, the complexity of customer behavior, and the need for continuous model updating.
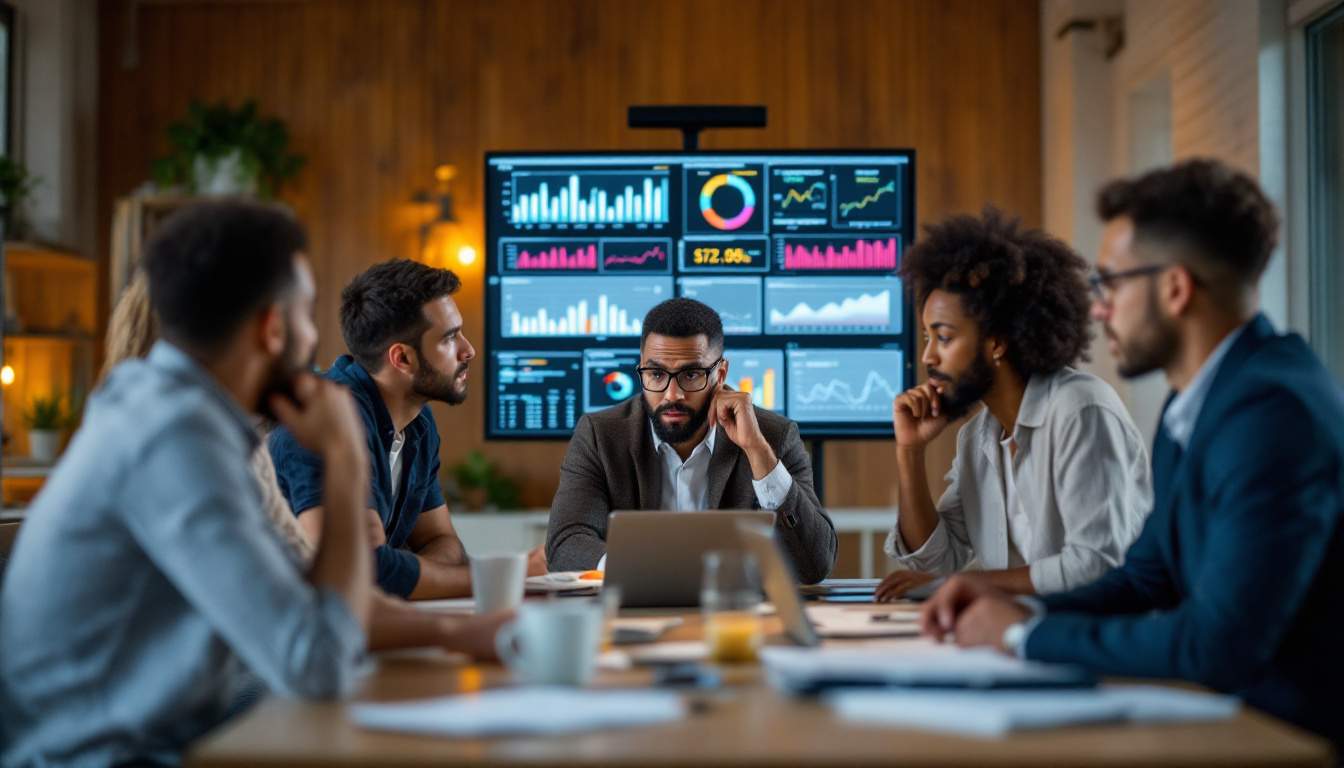
Understanding these challenges can help businesses implement effective predictive churn modeling strategies and make more accurate predictions.
Data Quality Issues
Data quality is a significant challenge in predictive churn modeling. Inaccurate or incomplete data can lead to inaccurate predictions, which can negatively impact customer retention efforts. Therefore, it’s crucial for businesses to ensure the quality and accuracy of the data used in predictive churn modeling.
Moreover, businesses need to collect data over a sufficient period to capture seasonal trends and other patterns in customer behavior. This can help improve the accuracy of the predictive churn model.
Complexity of Customer Behavior
Customer behavior is complex and can be influenced by a variety of factors, including personal preferences, economic conditions, and market trends. This complexity can make it challenging to accurately predict customer churn.
Therefore, it’s crucial for businesses to use advanced analytics and machine learning techniques that can handle the complexity of customer behavior and make accurate predictions.
Need for Continuous Model Updating
Predictive churn models need to be continuously updated to reflect changes in customer behavior and market conditions. This requires ongoing data collection and model retraining, which can be resource-intensive.
However, continuous model updating is crucial for maintaining the accuracy of the predictions and ensuring the effectiveness of customer retention efforts.
Conclusion
Predictive churn modeling is a powerful tool for customer retention. By using advanced analytics and machine learning to predict customer churn, businesses can take proactive steps to retain customers at risk of churning, thereby improving customer retention and increasing profitability.
However, predictive churn modeling presents several challenges, including data quality issues, the complexity of customer behavior, and the need for continuous model updating. Understanding these challenges can help businesses implement effective predictive churn modeling strategies and make more accurate predictions.