What is predictive behavioral modeling?
Predictive behavioral modeling leverages data analysis and statistical algorithms to predict future actions based on historical data. This approach allows marketers to tailor their strategies to meet the needs of their target audience more effectively, thereby increasing the likelihood of achieving their marketing objectives.
Understanding predictive behavioral modeling requires a deep dive into its core components, the methodologies used, its applications in marketing, and the benefits and challenges associated with its implementation. This article provides a comprehensive exploration of these aspects to provide a clear understanding of what predictive behavioral modeling entails.
Concept of Predictive Behavioral Modeling
Predictive behavioral modeling is rooted in the idea that past behavior is a strong indicator of future actions. It involves the use of statistical techniques to analyze historical data and identify patterns that can be used to forecast future behavior. The primary goal of this approach is to enable marketers to make informed decisions that will drive positive consumer responses.
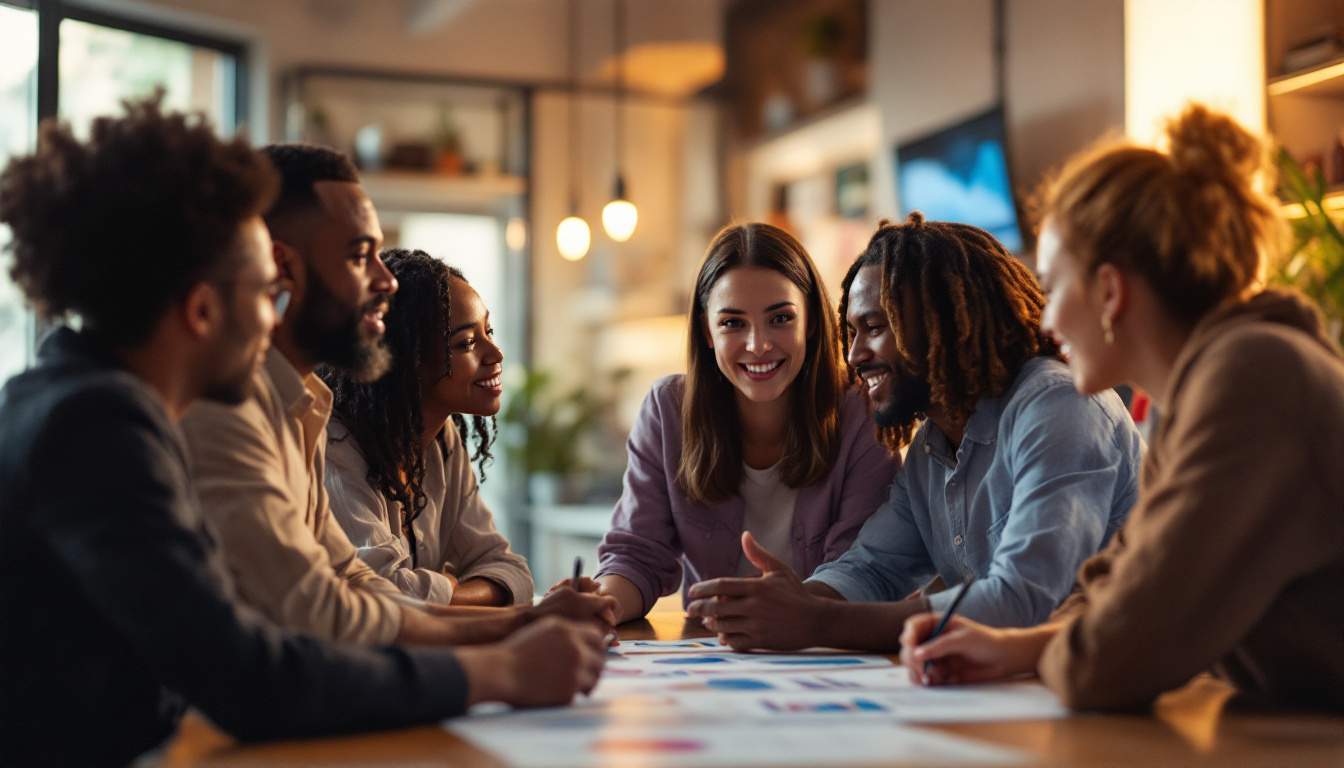
The concept of predictive behavioral modeling is not new; it has been used in various fields such as finance, healthcare, and social sciences. However, its application in marketing has gained significant traction in recent years due to the proliferation of data and advancements in data analysis technologies.
Components of Predictive Behavioral Modeling
The process of predictive behavioral modeling involves several components. The first is data collection, which involves gathering information about consumers’ past behavior. This can include data on purchasing behavior, browsing history, social media interactions, and other relevant information.
The next component is data analysis. This involves using statistical techniques to identify patterns and trends in the collected data. The insights derived from this analysis form the basis for predicting future behavior.
The final component is the application of the predictive model. This involves using the insights derived from the data analysis to inform marketing strategies. This can include tailoring marketing messages to individual consumers, optimizing marketing channels, and adjusting pricing strategies, among other things.
Methodologies Used in Predictive Behavioral Modeling
Several methodologies are used in predictive behavioral modeling. These methodologies are chosen based on the nature of the data, the specific objectives of the analysis, and the resources available. Some of the most commonly used methodologies include regression analysis, decision trees, and neural networks.
Regression analysis is a statistical technique used to determine the relationship between a dependent variable (the variable being predicted) and one or more independent variables (the variables used to make the prediction). This technique is commonly used in predictive behavioral modeling to identify the factors that influence consumer behavior.
Decision Trees
Decision trees are a type of predictive modeling technique that uses a tree-like model of decisions and their possible consequences. They are particularly useful in predictive behavioral modeling as they allow for the visualization of multiple potential outcomes based on different decision paths.
The nodes in a decision tree represent decisions, while the branches represent the outcomes of those decisions. The end points of the branches, known as leaves, represent the final outcomes. By tracing the paths from the root (the initial decision) to the leaves, marketers can predict the likely outcomes of different marketing strategies.
Neural Networks
Neural networks are a type of machine learning technique inspired by the human brain. They consist of interconnected layers of nodes, or “neurons,” that process information and make decisions. Neural networks are particularly effective in predictive behavioral modeling as they can handle large amounts of data and identify complex patterns.
Neural networks learn from the data they process, improving their predictions over time. This makes them a powerful tool for marketers, as they can adapt to changes in consumer behavior and continually refine their marketing strategies.
Applications of Predictive Behavioral Modeling in Marketing
Predictive behavioral modeling has a wide range of applications in marketing. One of the most common applications is in customer segmentation. By analyzing consumer behavior data, marketers can identify distinct groups of consumers with similar behaviors and preferences. This allows them to tailor their marketing strategies to meet the needs of each segment, thereby increasing the effectiveness of their marketing efforts.
Another application of predictive behavioral modeling is in campaign optimization. By predicting how consumers will respond to different marketing strategies, marketers can optimize their campaigns to maximize their return on investment. This can involve adjusting the timing of campaigns, the channels used, and the messages conveyed, among other things.
Customer Retention
Predictive behavioral modeling can also be used to improve customer retention. By predicting which customers are most likely to churn, marketers can implement strategies to retain these customers. This can involve offering special promotions, improving customer service, or addressing specific issues that are causing dissatisfaction.
Retaining existing customers is often more cost-effective than acquiring new ones, making this a valuable application of predictive behavioral modeling. Furthermore, satisfied customers are more likely to become brand advocates, further enhancing a company’s marketing efforts.
Product Development
Finally, predictive behavioral modeling can inform product development. By predicting what features or attributes consumers value most, companies can design products that meet these needs. This can lead to increased customer satisfaction, higher sales, and a stronger competitive position.
Predictive behavioral modeling can also help companies identify gaps in the market that they can exploit. This can lead to the development of innovative products that give the company a competitive edge.
Benefits of Predictive Behavioral Modeling
Predictive behavioral modeling offers several benefits to marketers. One of the key benefits is the ability to make data-driven decisions. By basing their strategies on solid data analysis, marketers can increase the likelihood of achieving their objectives.
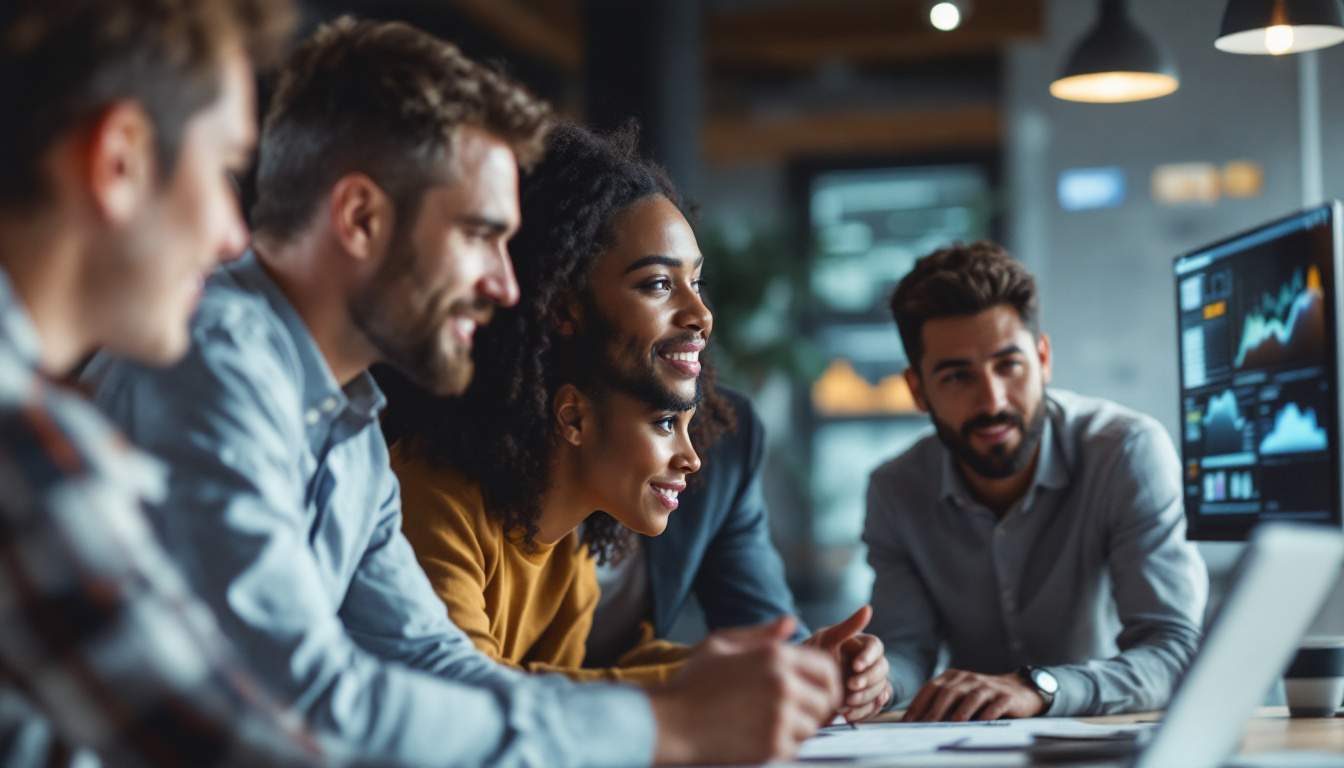
Another benefit of predictive behavioral modeling is the ability to personalize marketing efforts. By understanding the behaviors and preferences of individual consumers, marketers can tailor their messages to resonate with each consumer. This can lead to increased engagement, higher conversion rates, and improved customer loyalty.
Improved Efficiency
Predictive behavioral modeling can also improve efficiency. By predicting the likely outcomes of different strategies, marketers can allocate their resources more effectively. This can reduce waste, lower costs, and increase the return on investment.
Furthermore, predictive behavioral modeling can automate certain aspects of the marketing process. For example, it can automate the process of segmenting customers, allowing marketers to focus on other aspects of their strategies.
Competitive Advantage
Finally, predictive behavioral modeling can provide a competitive advantage. By leveraging data to make informed decisions, companies can stay ahead of their competitors. This can lead to increased market share, higher profits, and long-term success.
Moreover, predictive behavioral modeling can help companies identify opportunities and threats before their competitors do. This can allow them to react quickly and seize opportunities or mitigate threats, further enhancing their competitive position.
Challenges of Predictive Behavioral Modeling
Despite its benefits, predictive behavioral modeling also presents several challenges. One of the key challenges is the need for high-quality data. The accuracy of the predictions made by the model depends on the quality of the data used. If the data is inaccurate or incomplete, the predictions will be unreliable.
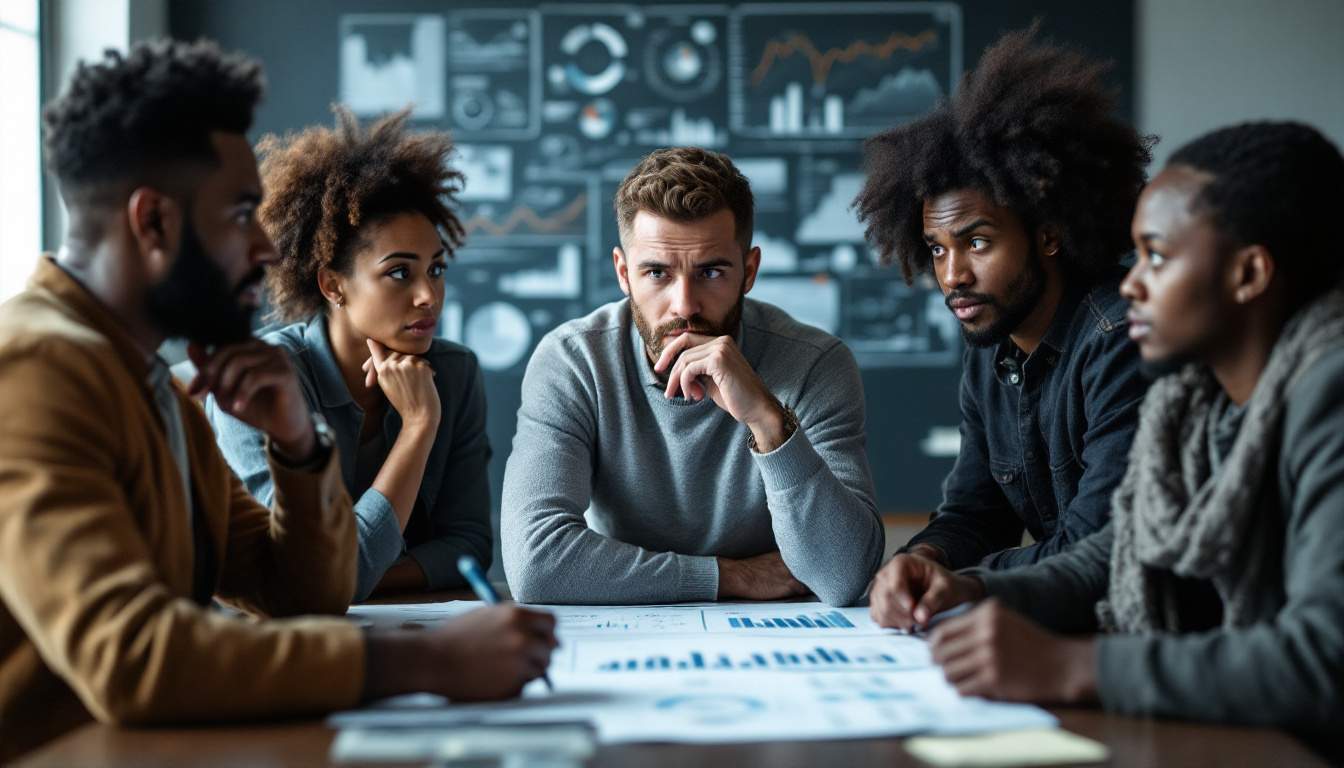
Another challenge is the complexity of the modeling process. Predictive behavioral modeling involves sophisticated statistical techniques and requires a deep understanding of data analysis. This can make it difficult for companies without the necessary expertise to implement this approach.
Data Privacy Concerns
Data privacy is another challenge associated with predictive behavioral modeling. The use of consumer data raises concerns about privacy and data protection. Companies must ensure that they comply with all relevant laws and regulations to avoid legal issues and damage to their reputation.
Furthermore, consumers are becoming increasingly aware of their data rights and may be reluctant to share their data. This can limit the amount of data available for analysis and affect the accuracy of the predictions.
Changing Consumer Behavior
Finally, the dynamic nature of consumer behavior presents a challenge for predictive behavioral modeling. Consumer behavior can change rapidly due to various factors such as changes in market conditions, societal trends, and personal circumstances. This can make it difficult to make accurate predictions.
However, this challenge can be mitigated by continually updating the predictive model with new data. This allows the model to adapt to changes in consumer behavior and maintain its accuracy.
Conclusion
Predictive behavioral modeling is a powerful tool that can enhance marketing strategies and drive business success. It offers numerous benefits, including the ability to make data-driven decisions, personalize marketing efforts, improve efficiency, and gain a competitive advantage. However, it also presents several challenges, including the need for high-quality data, the complexity of the modeling process, data privacy concerns, and the dynamic nature of consumer behavior.
Despite these challenges, the potential benefits of predictive behavioral modeling make it a valuable approach for marketers. By understanding and effectively leveraging this tool, marketers can optimize their strategies and achieve their objectives.