What are predictive analytics models?
Predictive analytics models are mathematical algorithms that use historical and current data to predict future outcomes. These models are used to forecast customer behavior, market trends, and business performance, among other things. By leveraging predictive analytics models, businesses can make data-driven decisions and optimize their strategies for maximum effectiveness and efficiency.
The use of predictive analytics models in marketing is not a new concept, but with the advent of big data and advanced machine learning techniques, their importance and utility have grown exponentially. They are now an integral part of any sophisticated marketing strategy, helping businesses to understand their customers better, target their marketing efforts more effectively, and ultimately drive more sales and profits.
Types of Predictive Analytics Models
There are several types of predictive analytics models, each with its own strengths and weaknesses. The choice of model depends on the specific business problem at hand, the type of data available, and the desired level of accuracy.
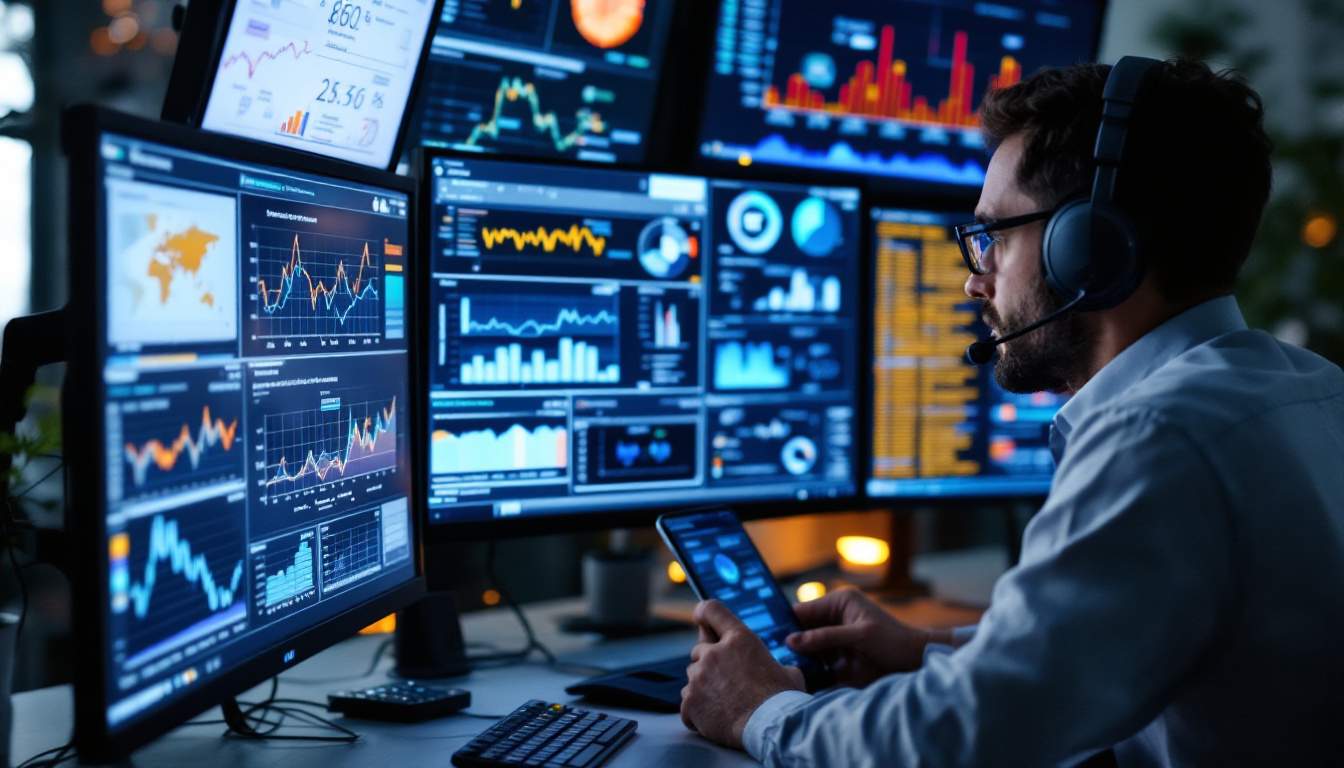
Some of the most common types of predictive analytics models used in marketing include regression models, time series models, decision trees, and neural networks. Each of these models has its own unique characteristics and is best suited to certain types of predictive tasks.
Regression Models
Regression models are a type of predictive analytics model that is used to predict a continuous outcome variable based on one or more predictor variables. In marketing, regression models are often used to predict sales based on factors such as advertising spend, price, and product features.
There are several types of regression models, including linear regression, logistic regression, and multiple regression. Each of these models has its own strengths and weaknesses, and the choice of model depends on the specific business problem at hand.
Time Series Models
Time series models are a type of predictive analytics model that is used to analyze and forecast data that is collected over time. In marketing, time series models are often used to forecast sales, customer demand, and market trends.
There are several types of time series models, including autoregressive models, moving average models, and autoregressive integrated moving average models. Each of these models has its own strengths and weaknesses, and the choice of model depends on the specific business problem at hand.
Applications of Predictive Analytics Models in Marketing
Predictive analytics models have a wide range of applications in marketing. They can be used to predict customer behavior, forecast market trends, optimize marketing strategies, and much more.
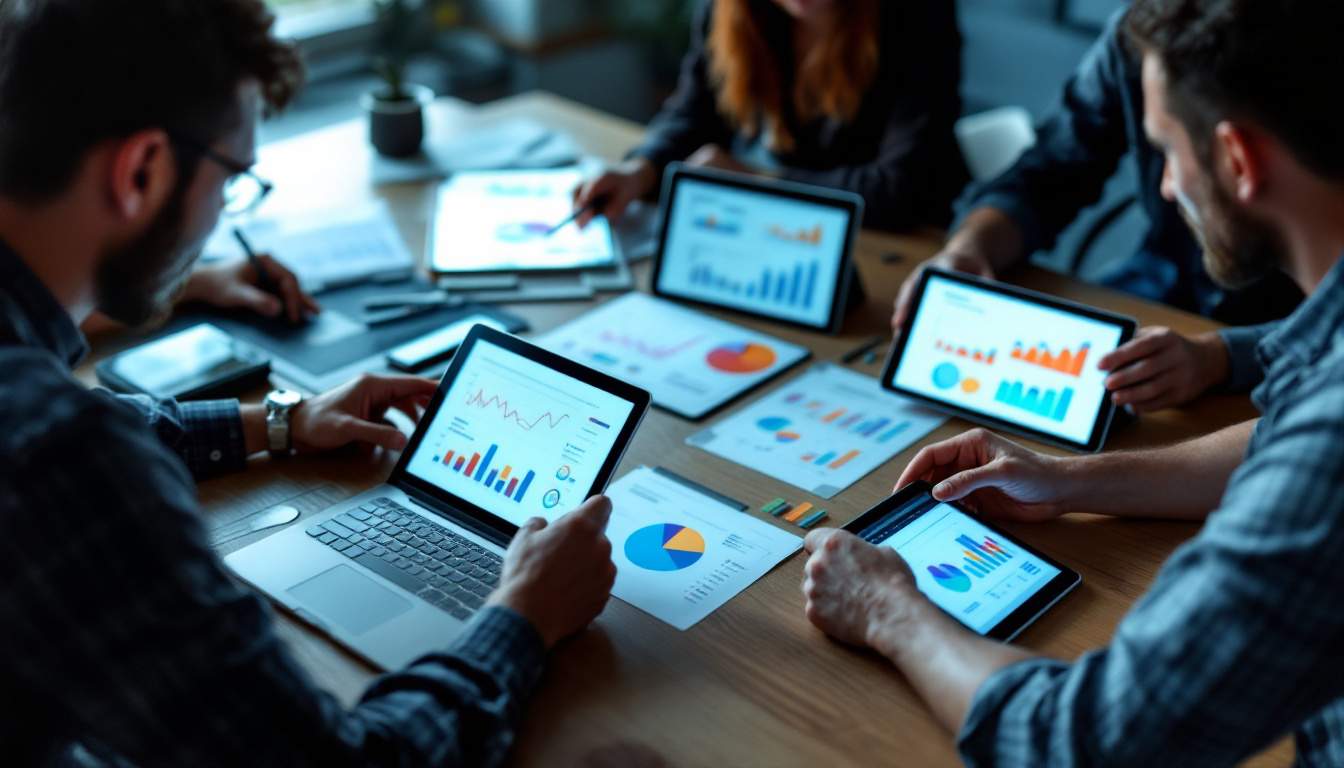
Some of the most common applications of predictive analytics models in marketing include customer segmentation, churn prediction, customer lifetime value prediction, and marketing mix modeling. Each of these applications has its own unique benefits and challenges, and the choice of application depends on the specific business objectives and resources available.
Customer Segmentation
Customer segmentation is the process of dividing a company’s customer base into distinct groups based on common characteristics such as demographics, buying behavior, and psychographics. Predictive analytics models can be used to identify these segments and predict their behavior, helping businesses to target their marketing efforts more effectively.
There are several types of predictive analytics models that can be used for customer segmentation, including clustering models, decision trees, and neural networks. Each of these models has its own strengths and weaknesses, and the choice of model depends on the specific business problem at hand.
Churn Prediction
Churn prediction is the process of identifying customers who are likely to stop doing business with a company in the near future. Predictive analytics models can be used to predict churn, helping businesses to retain their customers and reduce customer acquisition costs.
There are several types of predictive analytics models that can be used for churn prediction, including logistic regression models, decision trees, and neural networks. Each of these models has its own strengths and weaknesses, and the choice of model depends on the specific business problem at hand.
Challenges and Limitations of Predictive Analytics Models
While predictive analytics models offer many benefits, they also have their challenges and limitations. Understanding these challenges and limitations is crucial for businesses to effectively leverage predictive analytics models in their marketing strategies.
Some of the most common challenges and limitations of predictive analytics models include data quality issues, model overfitting, and the black box problem. Each of these challenges and limitations has its own implications for the use of predictive analytics models in marketing.
Data Quality Issues
Data quality is a critical factor in the success of any predictive analytics model. If the data used to train the model is incomplete, inaccurate, or biased, the model’s predictions will also be inaccurate or biased.
Ensuring data quality requires careful data collection, cleaning, and preprocessing. This can be a time-consuming and resource-intensive process, but it is crucial for the success of any predictive analytics model.
Model Overfitting
Model overfitting is a common problem in predictive analytics. It occurs when a model is too complex and fits the training data too closely, resulting in poor performance on new, unseen data.
Preventing model overfitting requires careful model selection and validation. This can be a complex process, but it is crucial for the success of any predictive analytics model.
Conclusion
Predictive analytics models are a powerful tool for modern marketing strategies. They offer a wealth of benefits, from improved customer understanding to optimized marketing strategies. However, they also come with their own challenges and limitations, and businesses must be aware of these in order to effectively leverage predictive analytics in their marketing efforts.
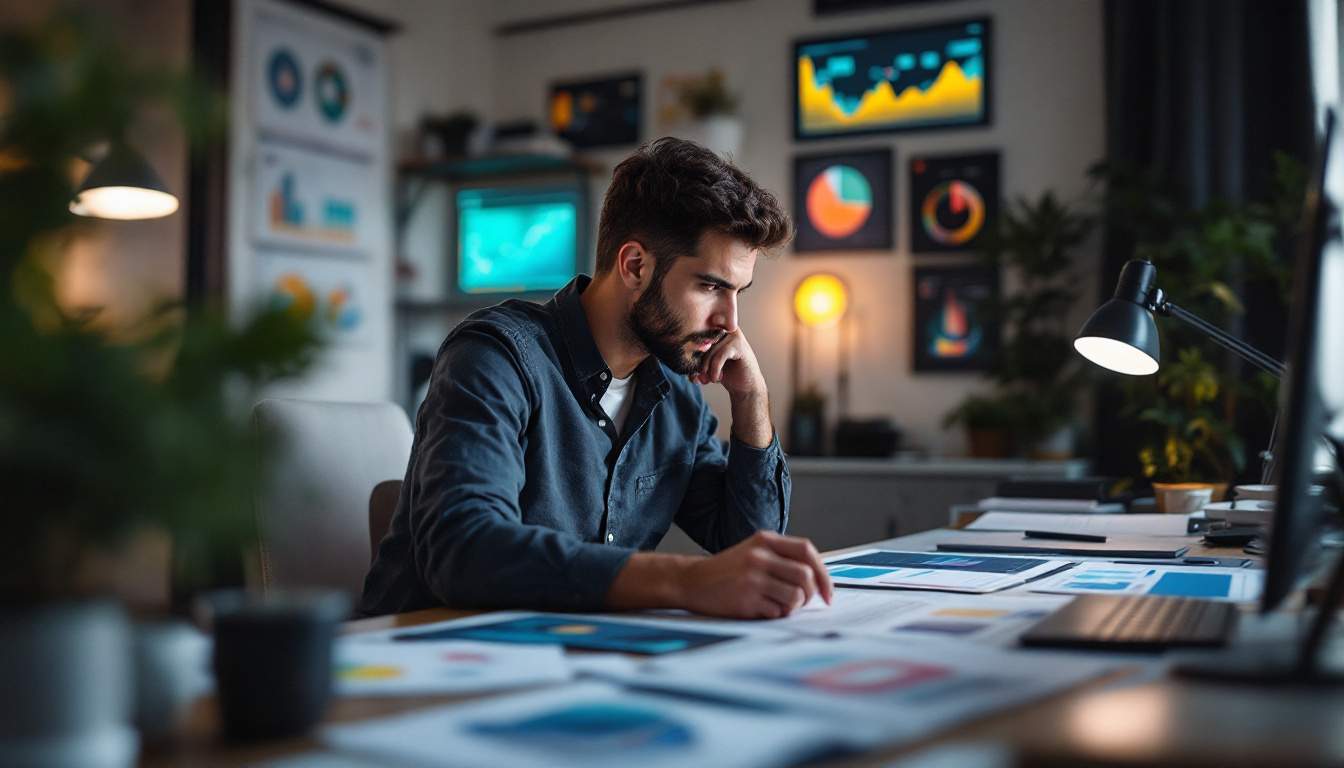
With the right approach, predictive analytics models can provide businesses with valuable insights and a competitive edge in today’s data-driven marketplace. As the field of predictive analytics continues to evolve, businesses that are able to effectively leverage these models will be well-positioned for success.