What is predictive analytics?
Predictive analytics is a branch of advanced analytics that uses both new and historical data to forecast future activity, behavior, and trends. It involves applying statistical analysis techniques, analytical queries, and automated machine learning algorithms to data sets to create predictive models that place a numerical value, or score, on the likelihood of a particular event happening.
This form of analytics is a multidisciplinary field that combines several techniques and processes from data mining, statistics, modeling, machine learning, and artificial intelligence (AI) to analyze current data and make predictions about the future. It is used in numerous industries to allow companies and organizations to become proactive, forward-looking, and knowledge-driven.
Understanding Predictive Analytics
Predictive analytics is a form of data analytics that uses data, statistical algorithms, and machine learning techniques to identify the likelihood of future outcomes based on historical data. The goal is to go beyond knowing what has happened to provide the best assessment of what will happen in the future.
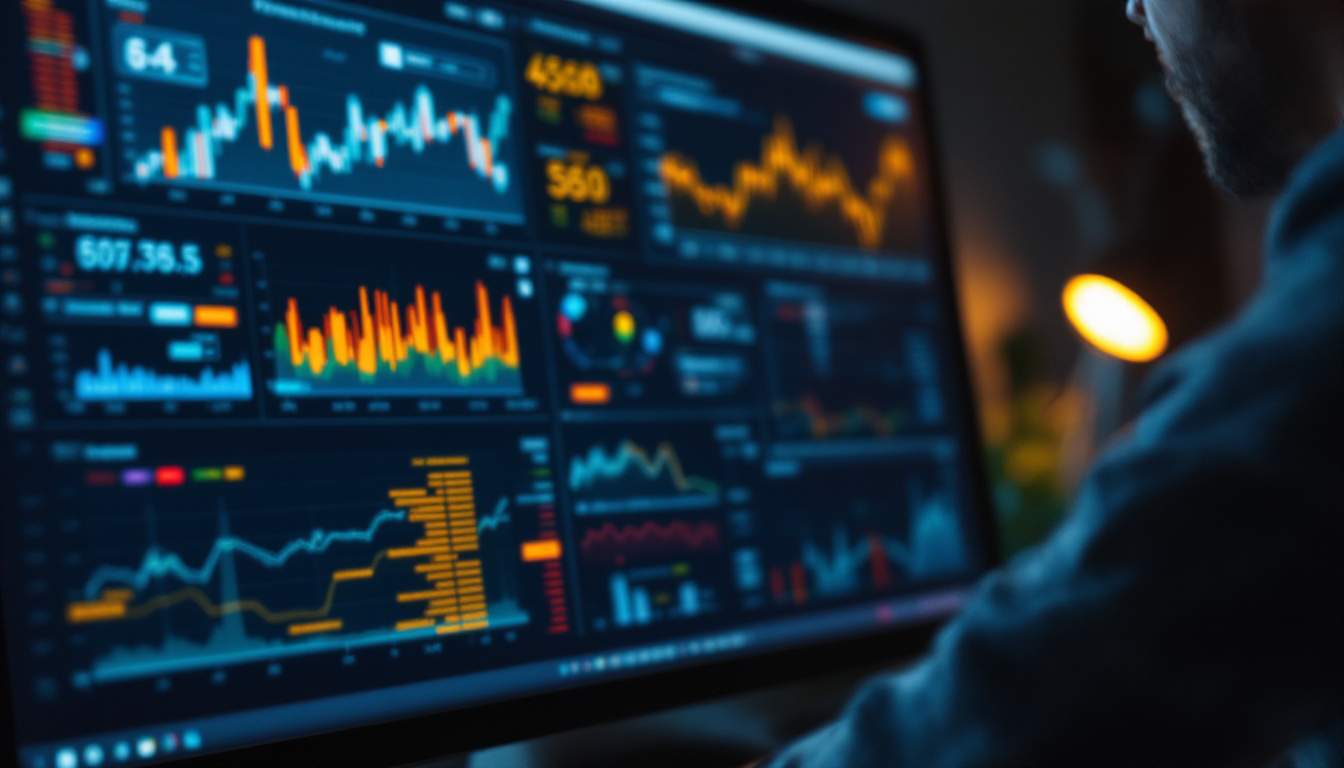
It uses many techniques from data mining, statistics, modeling, machine learning, and artificial intelligence to analyze current data to make predictions about future. It uses patterns found in historical and transactional data to identify risks and opportunities. Patterns found in historical information can be used to identify future risks and opportunities.
Components of Predictive Analytics
The process of predictive analytics can be broken down into several components. First, data is collected from various sources, which may include structured data (such as age, gender, and income level) and unstructured data (such as text, images, and voice recordings).
Next, this data is cleaned and preprocessed to remove any errors, inconsistencies, or biases that could skew the results. The cleaned data is then analyzed using statistical and machine learning algorithms to identify patterns and trends.
Types of Predictive Models
There are several types of predictive models that can be used depending on the nature of the data and the specific business problem. These include decision trees, regression models, neural networks, and time series models.
Decision trees are simple models that split the data into branches based on certain conditions, while regression models predict a continuous outcome variable based on one or more predictor variables. Neural networks are complex models that mimic the human brain to find patterns in large amounts of data, and time series models predict future values based on previously observed values.
Applications of Predictive Analytics
Predictive analytics has a wide range of applications across various industries. In marketing, it can be used to predict customer behavior, optimize marketing campaigns, and improve customer retention. In finance, it can be used to assess credit risk, detect fraud, and forecast market trends.
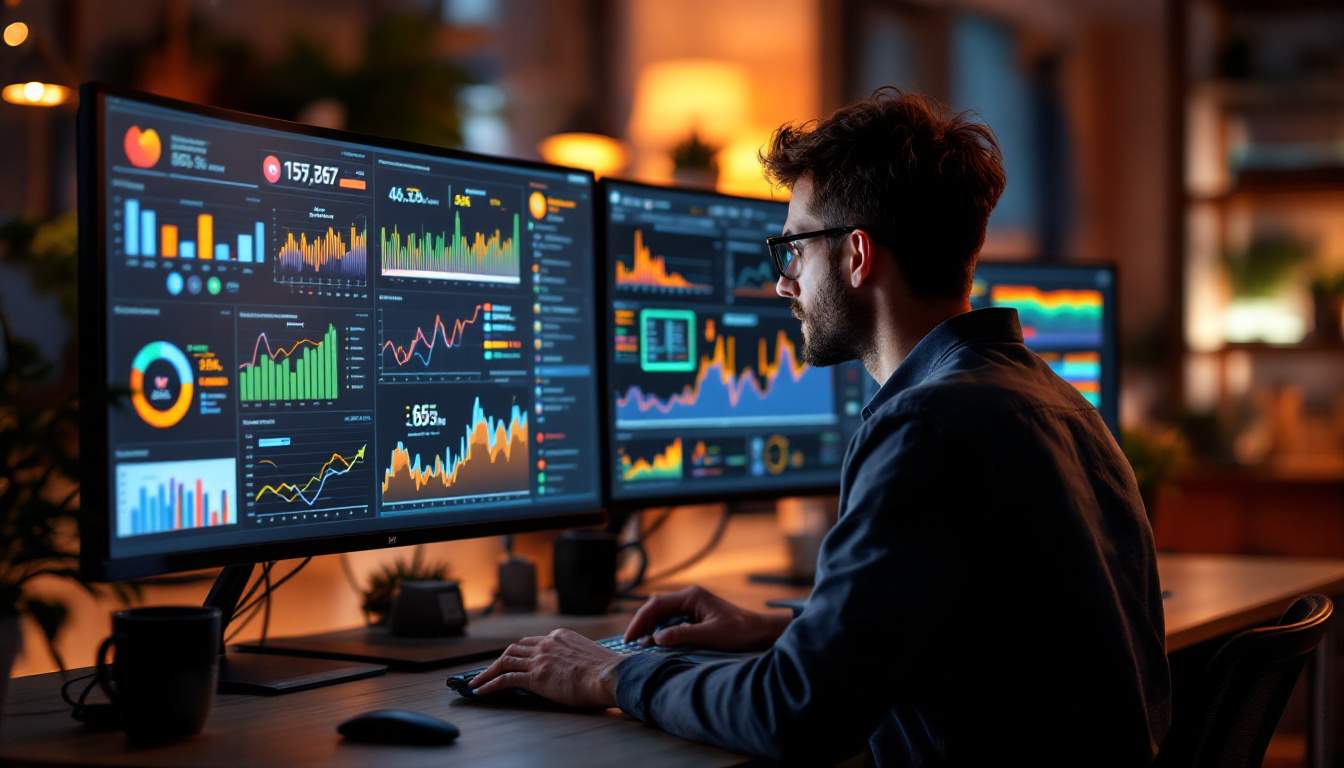
In healthcare, predictive analytics can be used to predict disease outbreaks, identify high-risk patients, and improve patient care. In retail, it can be used to forecast sales, optimize pricing, and manage inventory. In transportation, it can be used to optimize routes, predict maintenance needs, and improve fuel efficiency.
Benefits of Predictive Analytics
There are several benefits of using predictive analytics in business. One of the main benefits is the ability to make more informed decisions. By predicting future trends and outcomes, businesses can plan and prepare for the future more effectively.
Another benefit is the ability to identify opportunities and risks early on. This can give businesses a competitive advantage and help them avoid potential problems. Additionally, predictive analytics can help businesses improve their operations and increase their efficiency and productivity.
Challenges of Predictive Analytics
Despite its many benefits, predictive analytics also has its challenges. One of the main challenges is the quality and availability of data. For predictive analytics to be effective, it requires large amounts of high-quality data, which can be difficult to obtain.
Another challenge is the complexity of the algorithms and models used in predictive analytics. These algorithms and models can be difficult to understand and interpret, which can make it difficult for businesses to implement and use predictive analytics effectively.
Future of Predictive Analytics
The future of predictive analytics looks promising, with advancements in technology and data collection methods leading to more accurate and reliable predictions. The integration of predictive analytics with other technologies such as artificial intelligence and machine learning is also expected to drive growth in the field.
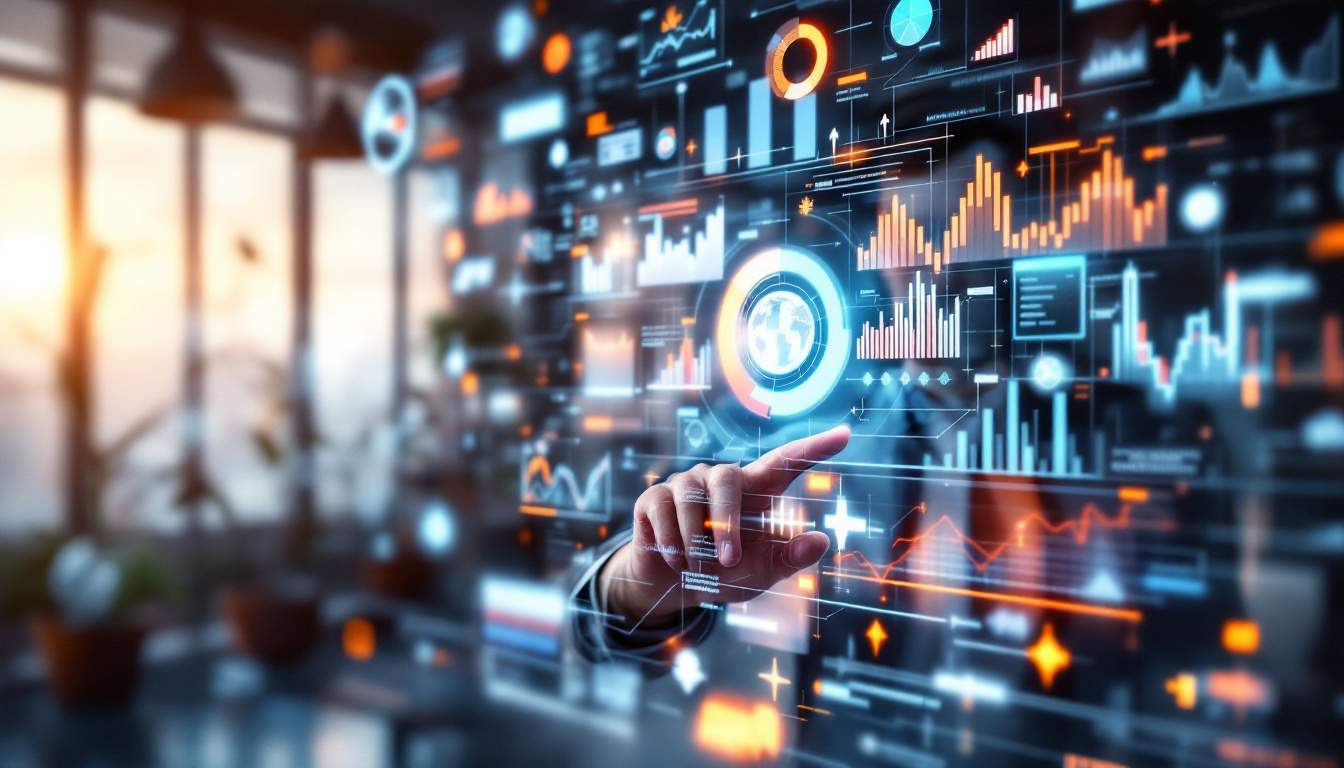
As businesses continue to recognize the value of data-driven decision making, the demand for predictive analytics is expected to grow. This will lead to more investment in predictive analytics tools and technologies, and more opportunities for businesses to leverage predictive analytics to gain a competitive advantage.
Impact of AI and Machine Learning on Predictive Analytics
Artificial intelligence and machine learning are having a significant impact on predictive analytics. These technologies are making it possible to analyze larger and more complex data sets, leading to more accurate and detailed predictions.
Machine learning algorithms can also learn and improve over time, which can lead to more accurate predictions. This is particularly useful in fields where patterns and trends can change rapidly, such as finance and marketing.
Role of Big Data in Predictive Analytics
Big data is playing a crucial role in the advancement of predictive analytics. The availability of large amounts of data is making it possible to make more accurate and detailed predictions. This is particularly important in fields where the accuracy of predictions can have a significant impact, such as healthcare and finance.
However, the use of big data in predictive analytics also presents challenges, such as data privacy and security concerns. It is therefore important for businesses to have robust data management and security measures in place when using big data for predictive analytics.
Conclusion
Predictive analytics is a powerful tool that can help businesses make more informed decisions, identify opportunities and risks, and improve their operations. However, it also presents challenges, such as data quality and availability, and the complexity of the algorithms and models used.
Despite these challenges, the future of predictive analytics looks promising, with advancements in technology and data collection methods leading to more accurate and reliable predictions. As businesses continue to recognize the value of data-driven decision making, the demand for predictive analytics is expected to grow, leading to more investment in predictive analytics tools and technologies, and more opportunities for businesses to leverage predictive analytics to gain a competitive advantage.