What are personalized recommendations?
Personalized recommendations, also known as personalized marketing or one-to-one marketing, is a marketing strategy that leverages data analysis and digital technology to deliver individualized messages and product offerings to current or prospective customers. This strategy is based on the idea that personalization can enhance customer engagement, increase customer loyalty, and drive more sales by providing customers with what they want, when they want it, and how they want it.
Personalized recommendations have become increasingly important in the digital age, where consumers are bombarded with an overwhelming amount of information and choices. By tailoring the marketing content to the individual’s needs and preferences, businesses can cut through the noise and make their messages more relevant and compelling. This approach can also help businesses to build deeper and more meaningful relationships with their customers, which can lead to higher customer retention rates and lifetime value.
Understanding Personalized Recommendations
Personalized recommendations are based on the premise that different customers have different needs, preferences, and behaviors. Therefore, a one-size-fits-all approach to marketing is unlikely to be effective. Instead, businesses should strive to understand each customer as an individual and tailor their marketing efforts accordingly. This can involve personalizing various aspects of the marketing mix, including the product, price, place, and promotion, to better meet the individual’s needs and preferences.
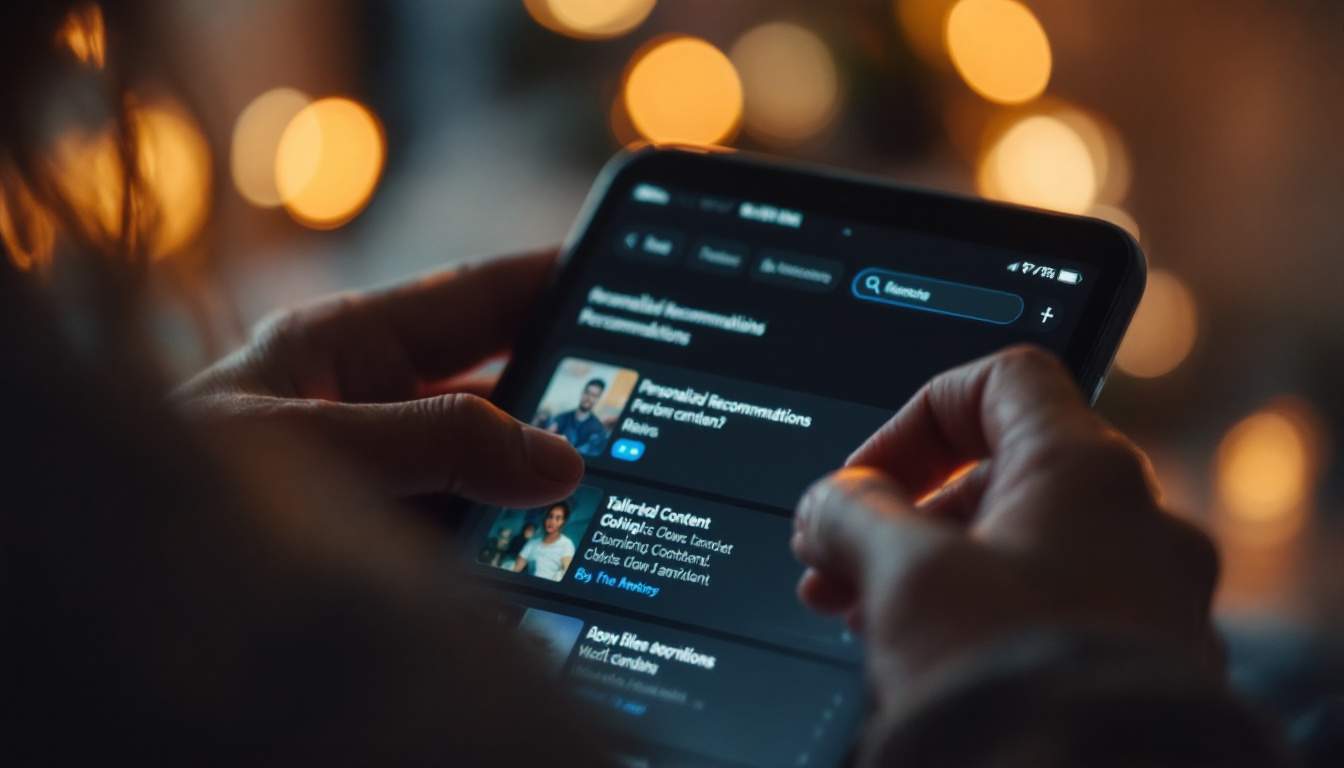
Personalized recommendations can be delivered in various ways, depending on the business’s capabilities and the customer’s preferences. For example, a business might send personalized emails to its customers, recommending products based on their past purchases. Alternatively, a business might use personalized pop-up ads on its website, recommending products based on the customer’s browsing history. The key is to deliver the right message to the right person at the right time, in a way that is relevant and engaging.
Benefits of Personalized Recommendations
Personalized recommendations can offer numerous benefits to businesses. First and foremost, they can help to increase sales by making the shopping experience more relevant and engaging for customers. By recommending products that are tailored to the customer’s needs and preferences, businesses can increase the likelihood that the customer will make a purchase. This can lead to higher conversion rates and average order values, which can boost the business’s bottom line.
Personalized recommendations can also help to enhance customer loyalty and retention. By showing customers that the business understands and values them as individuals, personalized recommendations can help to build a stronger emotional connection between the customer and the business. This can increase the customer’s satisfaction and loyalty, which can lead to repeat purchases and positive word-of-mouth referrals.
Challenges of Personalized Recommendations
While personalized recommendations can offer numerous benefits, they also present several challenges. One of the main challenges is the need for accurate and comprehensive data. In order to deliver personalized recommendations, businesses need to collect and analyze a large amount of data about their customers, including their demographic characteristics, purchase history, browsing behavior, and feedback. This can be a complex and time-consuming process, and it requires a robust data management system.
Another challenge is the need for advanced analytics capabilities. To make sense of the data and generate meaningful insights, businesses need to use sophisticated analytics techniques, such as machine learning and predictive modeling. These techniques require specialized skills and resources, which can be a barrier for some businesses. Furthermore, businesses need to be able to act on the insights in a timely and effective manner, which requires a flexible and responsive marketing strategy.
Types of Personalized Recommendations
There are several types of personalized recommendations, each with its own strengths and weaknesses. The most common types include collaborative filtering, content-based filtering, and hybrid approaches.
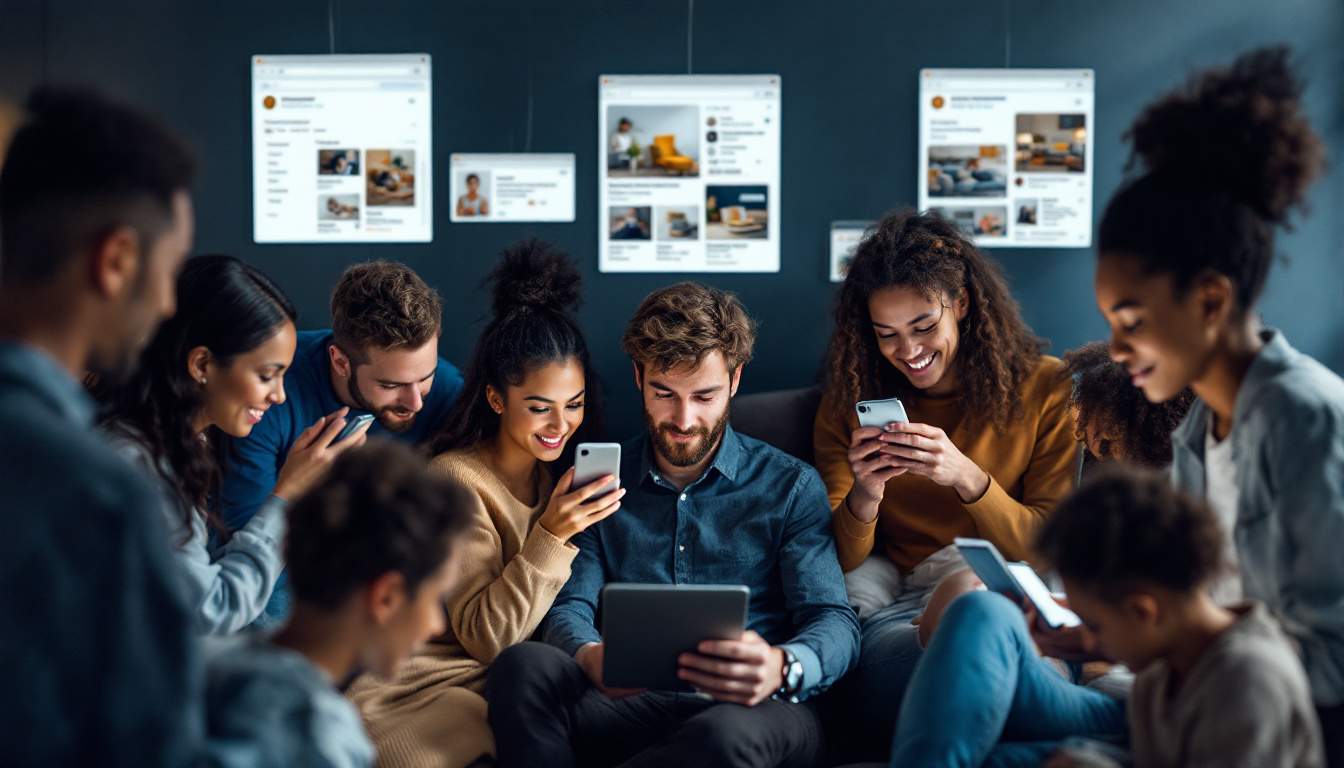
Collaborative filtering is a method of making automatic predictions about the interests of a user by collecting preferences from many users. This method assumes that if two users agree on one issue, they are likely to agree on others as well. For example, if user A and user B both liked product X, and user A also liked product Y, then the system would recommend product Y to user B.
Collaborative Filtering
Collaborative filtering is one of the most popular methods of personalized recommendations. It works by analyzing the behavior of many users to find similarities. Once these similarities are found, the system can recommend items that similar users have liked in the past. This method is particularly effective for recommending items that are popular among a large number of users.
However, collaborative filtering has some limitations. It requires a large amount of data to work effectively, which can be a challenge for new or small businesses. It also struggles with the “cold start” problem, where it is difficult to make recommendations for new users or items that have little to no data. Furthermore, collaborative filtering tends to recommend popular items, which can lead to a lack of diversity in the recommendations.
Content-Based Filtering
Content-based filtering is another common method of personalized recommendations. It works by analyzing the content of items to find similarities. Once these similarities are found, the system can recommend items that are similar to the ones the user has liked in the past. This method is particularly effective for recommending items that are similar in content, such as books in the same genre or clothes in the same style.
However, content-based filtering also has some limitations. It requires a detailed understanding of the content, which can be a challenge for complex or diverse items. It also struggles with the “new user” problem, where it is difficult to make recommendations for users who have little to no data. Furthermore, content-based filtering tends to recommend similar items, which can lead to a lack of novelty in the recommendations.
Implementing Personalized Recommendations
Implementing personalized recommendations requires a combination of data, technology, and strategy. First, businesses need to collect and manage a large amount of data about their customers. This can involve integrating various data sources, such as transaction data, web analytics data, and customer feedback, into a single customer database. Businesses also need to ensure that the data is accurate, up-to-date, and compliant with privacy regulations.
Next, businesses need to analyze the data to generate insights about their customers. This can involve using advanced analytics techniques, such as machine learning and predictive modeling, to identify patterns and trends in the data. Businesses also need to interpret the insights in the context of their business objectives and customer needs.
Technology for Personalized Recommendations
Technology plays a crucial role in implementing personalized recommendations. Businesses need to use a variety of technologies to collect, manage, and analyze data, as well as to deliver personalized recommendations. These technologies can include customer relationship management (CRM) systems, data management platforms (DMPs), analytics tools, and recommendation engines.
CRM systems can help businesses to manage their customer data and interactions in a systematic and efficient manner. DMPs can help businesses to collect, integrate, and manage large amounts of data from various sources. Analytics tools can help businesses to analyze the data and generate insights. Recommendation engines can help businesses to generate and deliver personalized recommendations based on the data and insights.
Strategy for Personalized Recommendations
Strategy is also important in implementing personalized recommendations. Businesses need to align their personalization efforts with their overall business objectives and customer strategy. This can involve defining clear goals for personalization, such as increasing sales, enhancing customer loyalty, or improving customer experience. Businesses also need to identify the key metrics to measure the success of their personalization efforts, such as conversion rate, customer satisfaction, or return on investment.
Furthermore, businesses need to develop a comprehensive personalization plan that outlines the key steps and resources required for implementation. This can involve identifying the key data sources, technologies, and skills required, as well as the key processes and workflows to be established. Businesses also need to ensure that their personalization efforts are customer-centric, meaning that they are designed to meet the needs and preferences of the customer, rather than the needs and preferences of the business.
Future of Personalized Recommendations
The future of personalized recommendations looks promising, with advances in technology and analytics paving the way for more sophisticated and effective personalization strategies. One of the key trends is the increasing use of artificial intelligence (AI) in personalization. AI can help businesses to analyze large amounts of data more quickly and accurately, generate more sophisticated insights, and deliver more personalized and dynamic recommendations.
Another key trend is the increasing importance of privacy in personalization. With the rise of data breaches and privacy scandals, consumers are becoming more aware and concerned about their data privacy. Therefore, businesses need to ensure that their personalization efforts are transparent, secure, and compliant with privacy regulations. This can involve obtaining explicit consent from customers, anonymizing personal data, and implementing robust data security measures.
Artificial Intelligence and Personalized Recommendations
Artificial intelligence is playing an increasingly important role in personalized recommendations. AI can help businesses to analyze large amounts of data more quickly and accurately, generate more sophisticated insights, and deliver more personalized and dynamic recommendations. For example, AI can use machine learning algorithms to learn from past data and predict future behavior, or natural language processing algorithms to understand and respond to customer queries in a more human-like manner.
However, the use of AI in personalized recommendations also presents several challenges. One of the main challenges is the need for high-quality data. AI algorithms require large amounts of accurate and relevant data to learn effectively, which can be a challenge for businesses with limited or poor-quality data. Another challenge is the need for transparency and explainability. AI algorithms can be complex and opaque, making it difficult for businesses to understand and explain how they work. This can lead to trust issues and regulatory concerns.
Privacy and Personalized Recommendations
Privacy is becoming increasingly important in personalized recommendations. With the rise of data breaches and privacy scandals, consumers are becoming more aware and concerned about their data privacy. Therefore, businesses need to ensure that their personalization efforts are transparent, secure, and compliant with privacy regulations. This can involve obtaining explicit consent from customers, anonymizing personal data, and implementing robust data security measures.
However, balancing privacy and personalization can be a challenge. On one hand, businesses need to collect and use personal data to deliver personalized recommendations. On the other hand, businesses need to respect the privacy rights of their customers and comply with privacy regulations. This requires a careful and thoughtful approach to data management and personalization strategy.
Conclusion
Personalized recommendations are a powerful tool for businesses to enhance customer engagement, increase customer loyalty, and drive more sales. However, implementing personalized recommendations requires a combination of data, technology, and strategy, as well as a careful balance of personalization and privacy. With advances in technology and analytics, the future of personalized recommendations looks promising, but also presents new challenges and opportunities for businesses.
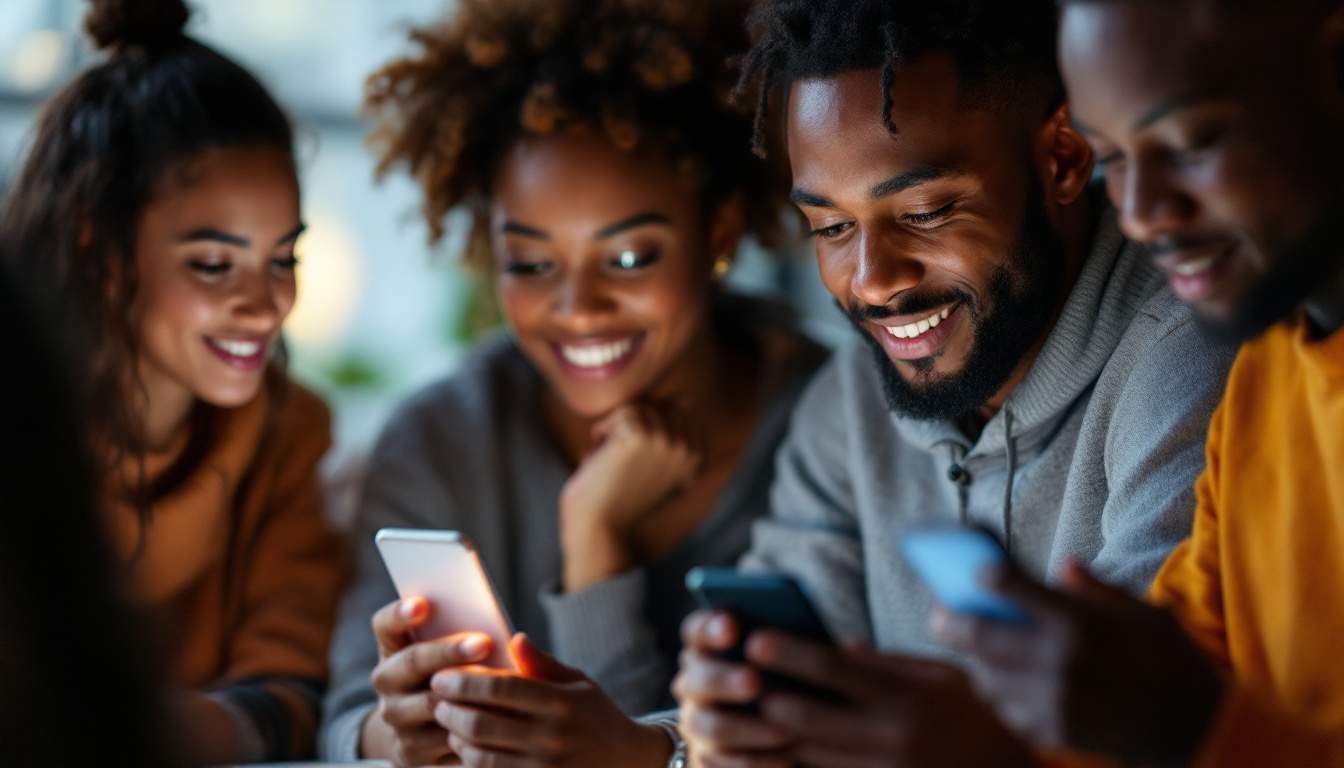
As personalized recommendations continue to evolve, businesses need to stay abreast of the latest trends and best practices, and continually adapt their personalization strategies to meet the changing needs and preferences of their customers. By doing so, businesses can deliver more relevant and compelling marketing content, build deeper and more meaningful relationships with their customers, and achieve greater business success.