What is machine learning in marketing?
Machine learning leverages artificial intelligence (AI) to optimize marketing strategies and improve customer experiences. It involves the use of algorithms and computational models to analyze large amounts of data, identify patterns, and make predictions or decisions without explicit programming. This technology has revolutionized the marketing industry by providing valuable insights, personalizing customer experiences, and automating repetitive tasks.
As the digital landscape continues to evolve, machine learning is becoming an indispensable tool for marketers. It enables them to understand customer behavior, predict future trends, and deliver personalized content at scale. This article will delve into the intricacies of machine learning in marketing, explaining its key concepts, applications, benefits, and challenges in comprehensive detail.
Understanding Machine Learning
Machine learning is a subset of artificial intelligence that focuses on the development of algorithms and statistical models that computers use to perform tasks without explicit instructions. Instead, these systems learn from patterns and trends in data, improving their performance over time. Machine learning can be categorized into three main types: supervised learning, unsupervised learning, and reinforcement learning.
Supervised learning involves training a model on a labeled dataset, where the correct answers are known. The model then applies what it has learned to new data. Unsupervised learning, on the other hand, involves training a model on an unlabeled dataset, where the correct answers are unknown. The model identifies patterns and structures in the data. Reinforcement learning involves an agent that learns to perform actions based on rewards and punishments. The agent learns to make decisions that maximize the reward over time.
Key Concepts in Machine Learning
Several key concepts underpin machine learning, including algorithms, models, training, and prediction. An algorithm is a set of rules or instructions that a machine follows to solve a problem or accomplish a task. A model is a mathematical representation of a real-world process. Training involves feeding data to a machine learning algorithm to create a model, while prediction involves using the model to make forecasts or decisions based on new data.
Other important concepts include features and labels. Features are the input variables that the model uses to make predictions, while labels are the output variables that the model predicts. For example, in a marketing context, features might include customer demographics and purchasing history, while the label might be whether the customer will make a purchase in the future.
Types of Machine Learning Algorithms
There are many types of machine learning algorithms, each with its strengths and weaknesses. Some of the most common include decision trees, neural networks, support vector machines, and k-nearest neighbors. Decision trees are simple yet powerful algorithms that can handle both classification and regression tasks. Neural networks are complex algorithms inspired by the human brain, capable of learning from large amounts of data. Support vector machines are effective for classification tasks, especially in high-dimensional spaces. K-nearest neighbors is a simple algorithm that classifies new data points based on their similarity to existing data points.
Choosing the right algorithm depends on the problem at hand, the nature of the data, and the desired outcome. For example, neural networks might be suitable for image recognition tasks, while decision trees might be more appropriate for predicting customer churn.
Machine Learning in Marketing
Machine learning has a wide range of applications in marketing, from customer segmentation and targeting to content personalization and predictive analytics. By analyzing large amounts of data, machine learning algorithms can uncover hidden patterns and insights that can inform marketing strategies and drive business growth.
One of the most significant benefits of machine learning in marketing is its ability to personalize customer experiences. By analyzing customer behavior and preferences, machine learning algorithms can deliver personalized content and recommendations, improving customer engagement and loyalty. Additionally, machine learning can automate repetitive tasks, freeing up marketers to focus on strategic initiatives.
Customer Segmentation and Targeting
Machine learning can enhance customer segmentation and targeting by analyzing customer data and identifying distinct groups based on various characteristics. These groups can then be targeted with personalized marketing messages. For example, a machine learning algorithm might identify a group of customers who frequently purchase a particular product, enabling the marketer to target this group with relevant promotions.
Moreover, machine learning can predict future customer behavior based on past behavior. This can help marketers identify high-value customers, predict churn, and optimize customer lifetime value. For example, a machine learning model might predict that a customer is likely to churn based on their recent purchase history, enabling the marketer to take proactive measures to retain the customer.
Content Personalization and Recommendation
Machine learning can personalize content and recommendations based on customer behavior and preferences. This can improve customer engagement and conversion rates. For example, a machine learning algorithm might recommend products based on a customer’s browsing history, or personalize email marketing messages based on a customer’s past purchases.
Additionally, machine learning can optimize content delivery by determining the optimal time to send marketing messages. For example, a machine learning model might predict that a customer is most likely to open an email at a certain time of day, enabling the marketer to schedule the email accordingly.
Benefits of Machine Learning in Marketing
Machine learning offers numerous benefits in marketing, including improved customer insights, personalized experiences, and operational efficiency. By analyzing large amounts of data, machine learning can uncover hidden patterns and insights that can inform marketing strategies and drive business growth.
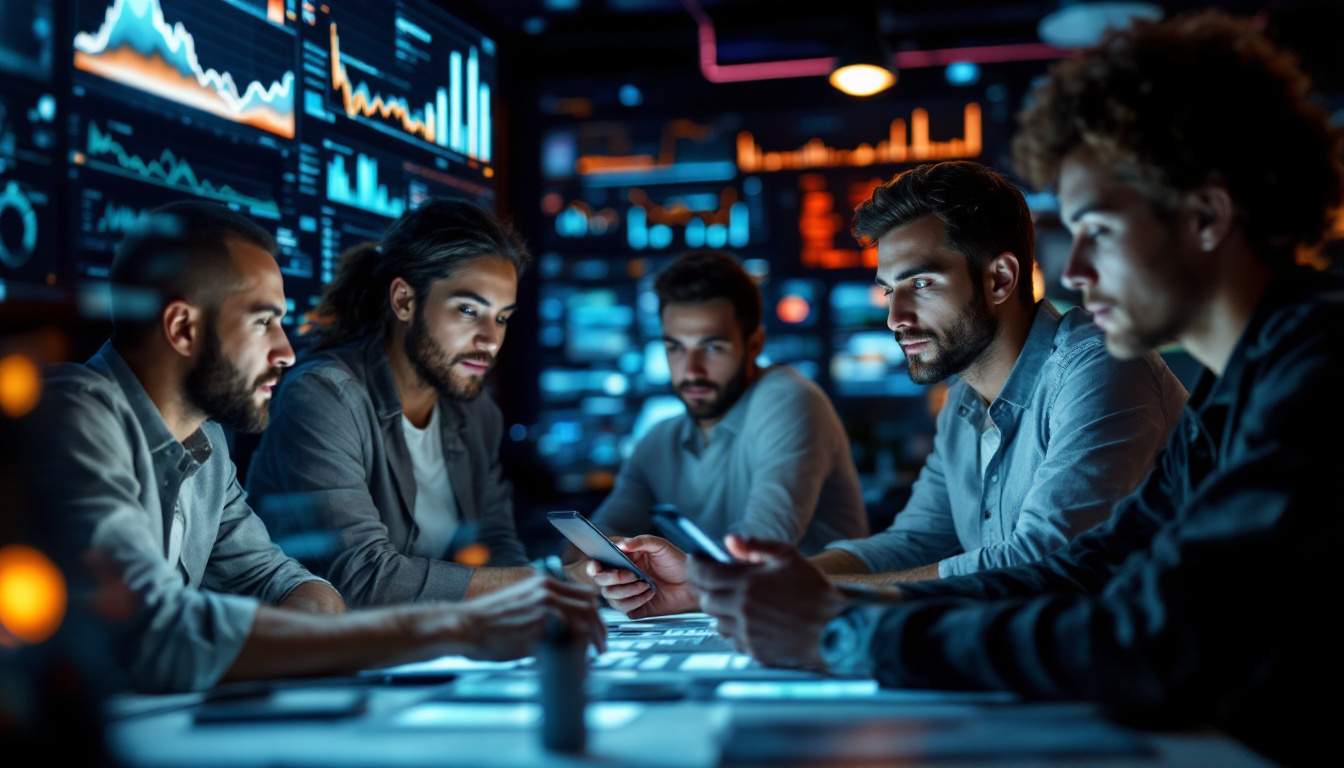
One of the most significant benefits of machine learning in marketing is its ability to personalize customer experiences. By analyzing customer behavior and preferences, machine learning algorithms can deliver personalized content and recommendations, improving customer engagement and loyalty. Additionally, machine learning can automate repetitive tasks, freeing up marketers to focus on strategic initiatives.
Improved Customer Insights
Machine learning can analyze large amounts of customer data to uncover hidden patterns and insights. This can provide a deeper understanding of customer behavior and preferences, informing marketing strategies and driving business growth. For example, a machine learning algorithm might identify trends in customer purchasing behavior, enabling the marketer to tailor their product offerings accordingly.
Moreover, machine learning can predict future customer behavior based on past behavior. This can help marketers identify high-value customers, predict churn, and optimize customer lifetime value. For example, a machine learning model might predict that a customer is likely to churn based on their recent purchase history, enabling the marketer to take proactive measures to retain the customer.
Personalized Customer Experiences
Machine learning can personalize customer experiences by analyzing customer behavior and preferences. This can improve customer engagement and loyalty. For example, a machine learning algorithm might recommend products based on a customer’s browsing history, or personalize email marketing messages based on a customer’s past purchases.
Additionally, machine learning can optimize content delivery by determining the optimal time to send marketing messages. For example, a machine learning model might predict that a customer is most likely to open an email at a certain time of day, enabling the marketer to schedule the email accordingly.
Challenges of Machine Learning in Marketing
Despite its many benefits, machine learning also presents several challenges in marketing. These include data quality and privacy issues, the need for technical expertise, and the risk of over-reliance on algorithms.
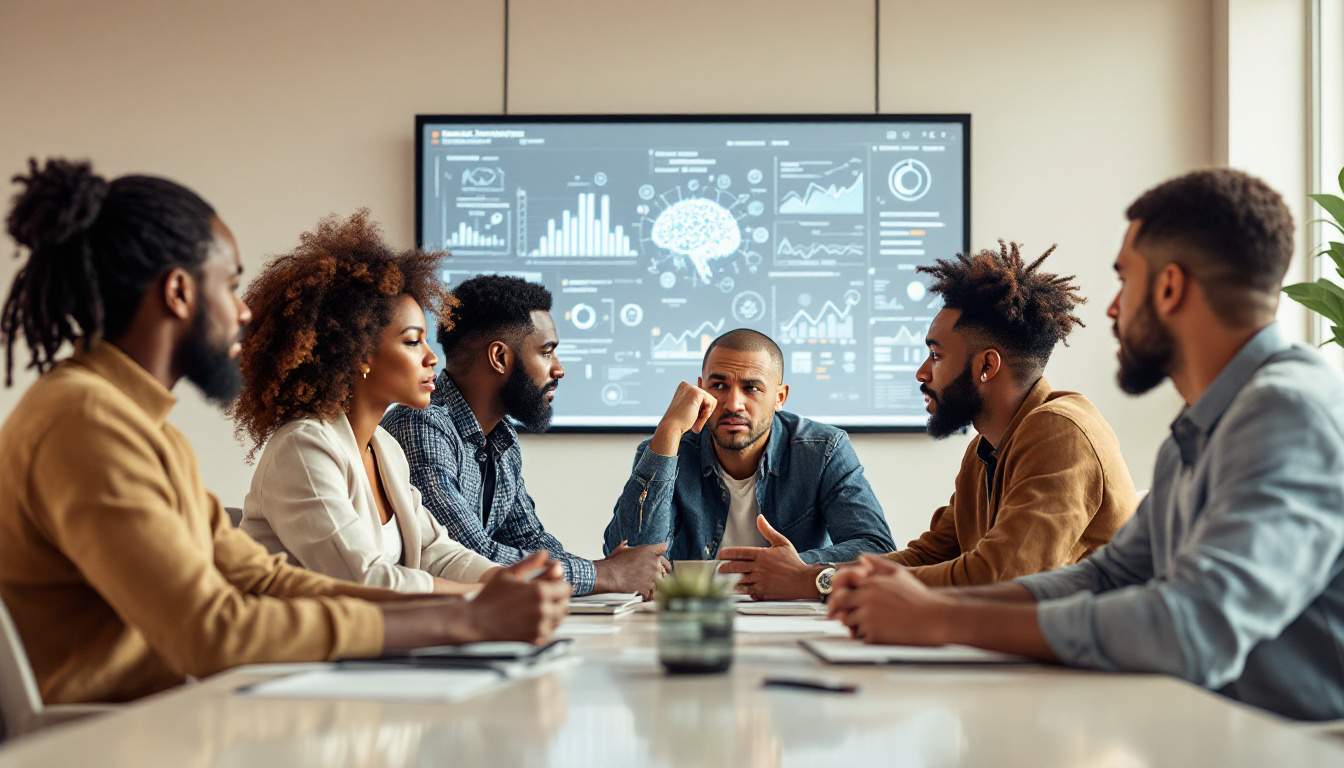
Data quality is a critical factor in the success of machine learning in marketing. Poor quality data can lead to inaccurate predictions and decisions. Additionally, privacy concerns can arise when collecting and analyzing customer data. Marketers must ensure they comply with data protection regulations and respect customer privacy.
Data Quality and Privacy
Data quality is a critical factor in the success of machine learning in marketing. Poor quality data can lead to inaccurate predictions and decisions. For example, if the data used to train a machine learning model is biased or incomplete, the model’s predictions may also be biased or inaccurate. Therefore, marketers must ensure they collect high-quality, representative data.
Privacy concerns can also arise when collecting and analyzing customer data. Marketers must ensure they comply with data protection regulations and respect customer privacy. This includes obtaining consent for data collection and use, providing transparency about how data is used, and implementing measures to protect data from unauthorized access and breaches.
Technical Expertise and Over-reliance on Algorithms
Implementing machine learning in marketing requires technical expertise. Marketers must understand the basics of machine learning, including algorithms, models, and data analysis techniques. They must also be able to interpret the results of machine learning models and apply them to marketing strategies.
There is also a risk of over-reliance on algorithms in marketing. While machine learning can provide valuable insights and automate tasks, it should not replace human judgment and creativity. Marketers must balance the use of machine learning with human intuition and experience.
Conclusion
Machine learning in marketing is a powerful tool that can provide valuable insights, personalize customer experiences, and automate tasks. However, it also presents challenges, including data quality and privacy issues, the need for technical expertise, and the risk of over-reliance on algorithms.
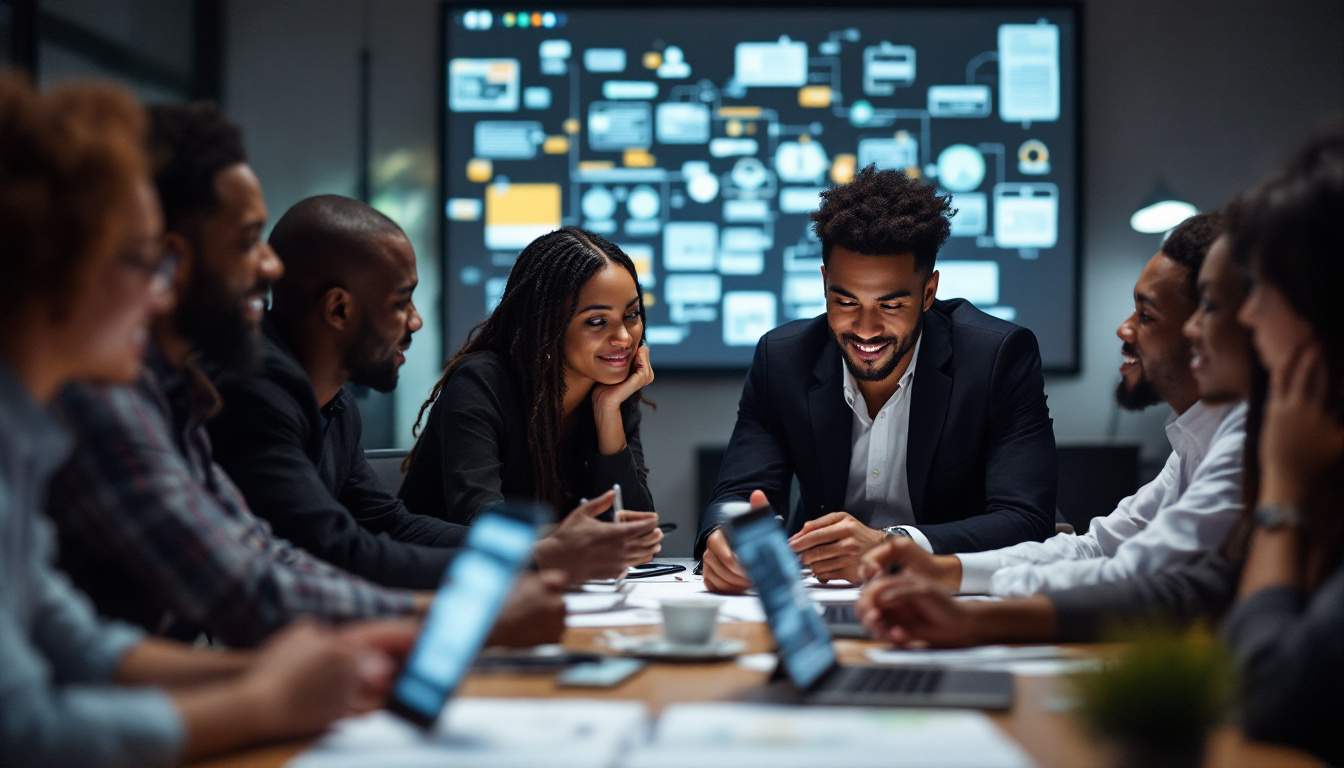
Despite these challenges, the benefits of machine learning in marketing are significant. By understanding and leveraging this technology, marketers can drive business growth and stay ahead in the competitive digital landscape.