What is data cleansing?
Data cleansing, also known as data cleaning or data scrubbing, refers to the process of identifying and correcting or removing errors, inaccuracies, and inconsistencies in datasets. This process is crucial in maintaining the quality of data, which is a vital asset in any business or organization. Data cleansing ensures that data is accurate, consistent, and reliable, thereby enabling businesses to make informed decisions based on this data.
With the growing importance of data in today’s digital age, the role of data cleansing has become more significant than ever. Businesses collect vast amounts of data from various sources, and this data is often riddled with errors and inconsistencies. These errors can lead to incorrect conclusions and poor business decisions. Therefore, data cleansing is an essential process in ensuring the integrity and reliability of data.
Understanding Data Cleansing
Data cleansing is a multi-step process that involves various techniques and methodologies. It begins with data auditing, where the data is initially analyzed to identify any errors or inconsistencies. This is followed by workflow specification, where the process of how the data will be cleaned is defined. The next step is workflow execution, where the actual cleaning of the data takes place. Finally, post-processing and controlling are done to ensure the quality of the cleaned data.
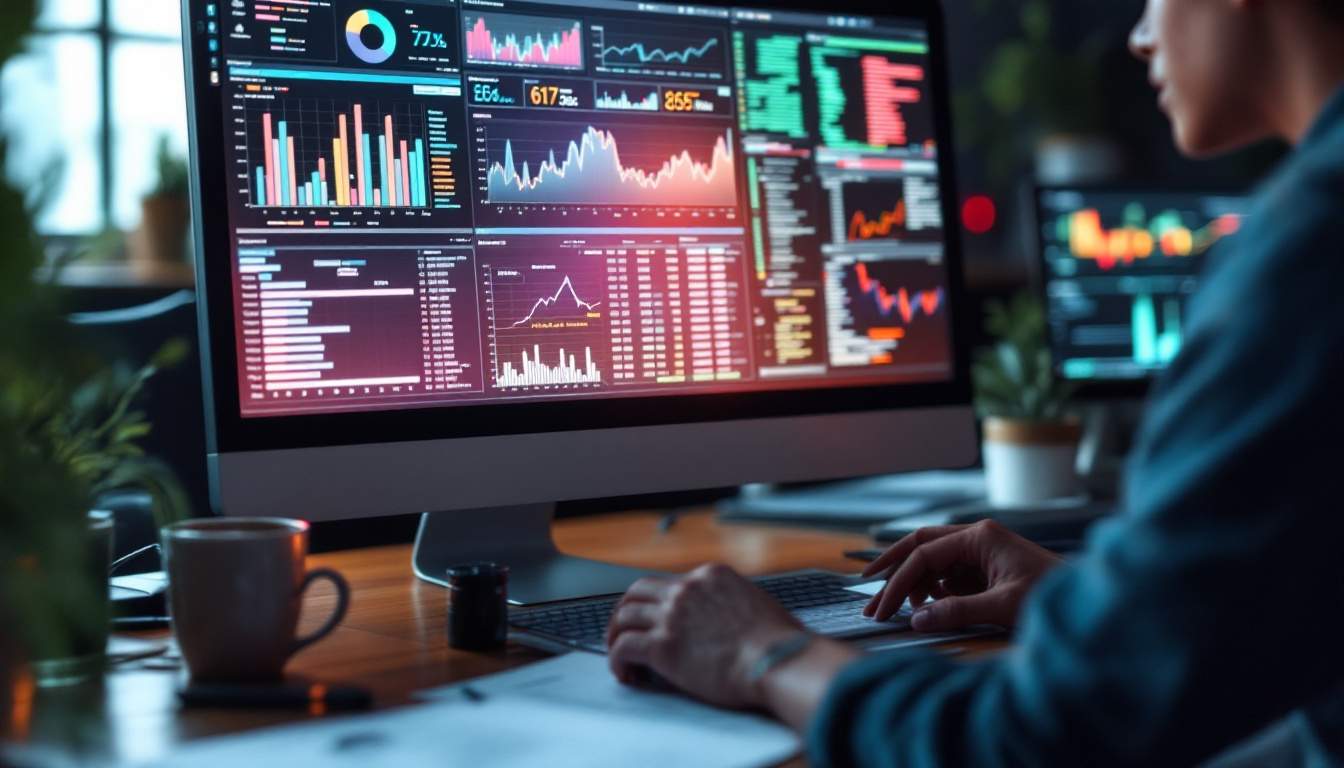
The process of data cleansing can be done manually or with the help of data cleansing tools. Manual data cleansing involves a person or a team of people going through the dataset and correcting errors. On the other hand, data cleansing tools are software applications that automate the process of data cleansing. These tools have algorithms and rules that can identify and correct errors in the data.
Importance of Data Cleansing
Data cleansing plays a crucial role in maintaining the quality of data. High-quality data is essential in making accurate and reliable business decisions. With clean data, businesses can gain valuable insights, make accurate forecasts, and develop effective strategies. Moreover, data cleansing can also help in improving the efficiency of business operations by eliminating redundant data.
Furthermore, data cleansing is also important in complying with regulations. Many industries have strict regulations regarding data accuracy and consistency. By ensuring that their data is clean, businesses can avoid penalties and legal issues associated with non-compliance.
Challenges in Data Cleansing
Despite its importance, data cleansing can be a challenging process. One of the main challenges is the sheer volume of data that needs to be cleaned. With the advent of big data, businesses are dealing with vast amounts of data that can be overwhelming to clean.
Another challenge is the complexity of data. Data comes in various formats and from various sources, making it difficult to clean. Moreover, the process of data cleansing itself can be complex, requiring specialized skills and knowledge.
Methods of Data Cleansing
Data cleansing can be done using various methods, depending on the nature of the data and the specific requirements of the business. Some of the common methods of data cleansing include data profiling, data standardization, data matching, data enrichment, and data monitoring.
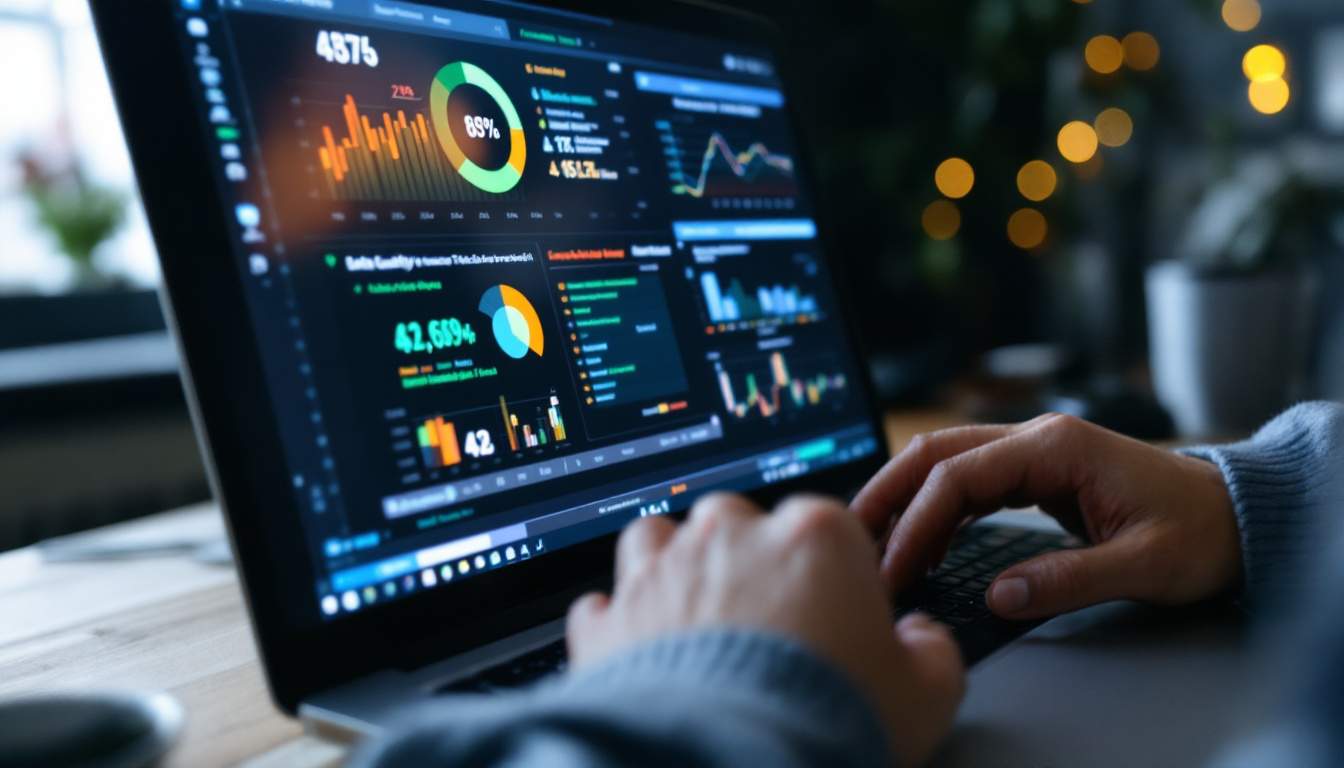
Data profiling involves analyzing the data to understand its structure, content, and quality. Data standardization involves converting the data into a common format. Data matching involves identifying and linking related records within or across datasets. Data enrichment involves enhancing the data with additional information. Finally, data monitoring involves continuously checking the data to ensure its quality over time.
Data Cleansing Tools
There are several data cleansing tools available in the market that can automate the process of data cleansing. These tools can handle large volumes of data and can clean data from various sources and in various formats. Some of the popular data cleansing tools include Data Ladder, IBM InfoSphere QualityStage, Informatica Data Quality, and Talend Data Quality.
These tools have various features that can aid in the process of data cleansing. For example, they can detect and correct errors in the data, standardize data, match and link related records, enrich data with additional information, and monitor data quality over time. By using these tools, businesses can significantly improve the efficiency and effectiveness of their data cleansing process.
Manual Data Cleansing
While data cleansing tools can automate the process of data cleansing, there are instances where manual data cleansing is necessary. For example, if the data is small in volume or if the errors in the data are complex and require human judgment, manual data cleansing may be the best option.
Manual data cleansing involves a person or a team of people going through the dataset and correcting errors. This process can be time-consuming and labor-intensive, but it can also be more accurate and thorough, especially for complex errors that cannot be detected by data cleansing tools.
Benefits of Data Cleansing
Data cleansing offers numerous benefits to businesses. One of the main benefits is improved decision-making. With clean data, businesses can make accurate and reliable decisions, leading to better business outcomes. Clean data can provide valuable insights, accurate forecasts, and effective strategies.
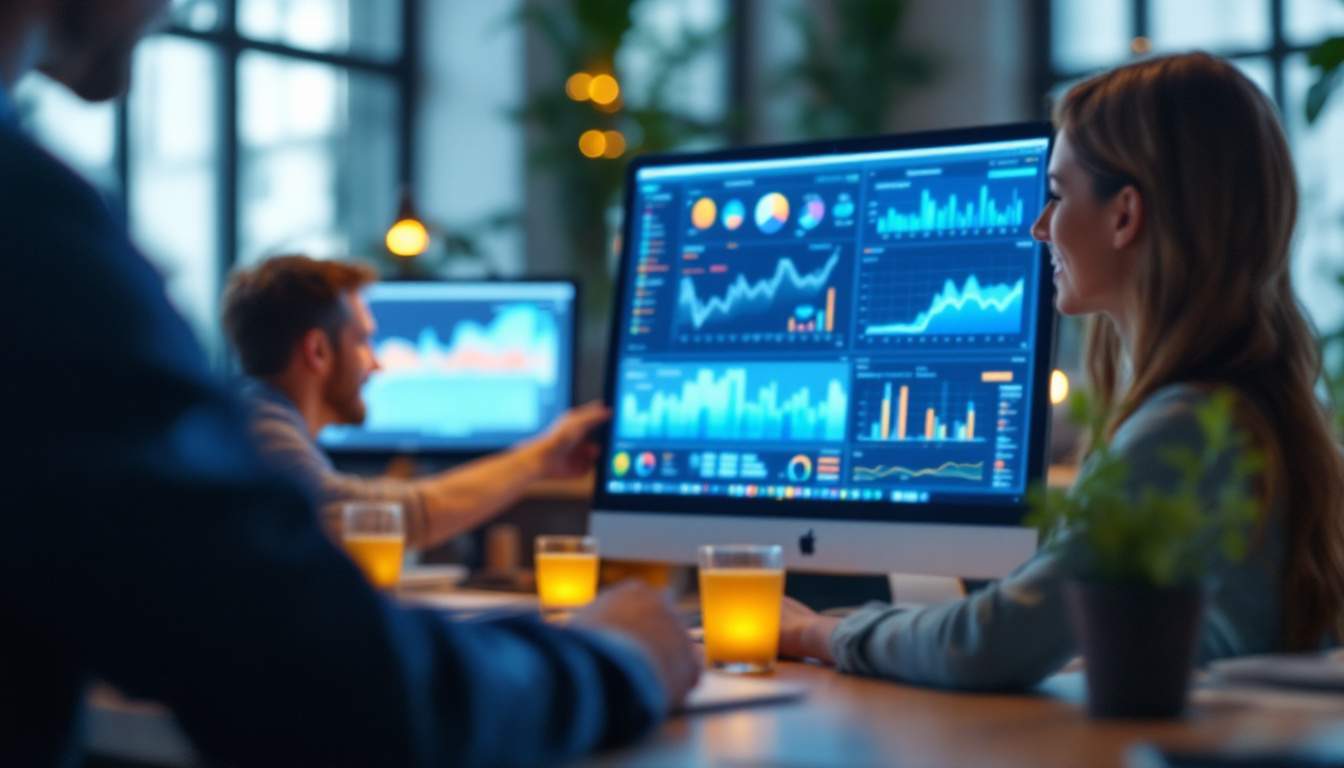
Another benefit of data cleansing is increased operational efficiency. By eliminating redundant data, businesses can streamline their operations and improve their efficiency. Moreover, clean data can also lead to improved customer service, as businesses can provide more accurate and personalized services to their customers based on clean data.
Compliance with Regulations
Data cleansing can also help businesses comply with regulations. Many industries have strict regulations regarding data accuracy and consistency. By ensuring that their data is clean, businesses can avoid penalties and legal issues associated with non-compliance.
Furthermore, data cleansing can also enhance the reputation of a business. Businesses that maintain high-quality data are often seen as reliable and trustworthy, which can improve their reputation in the market.
Cost Savings
Data cleansing can also lead to cost savings. By eliminating redundant data, businesses can save on storage costs. Moreover, clean data can also reduce the costs associated with bad decisions made based on inaccurate data.
Furthermore, by automating the process of data cleansing with data cleansing tools, businesses can save on labor costs. These tools can clean data faster and more efficiently than manual data cleansing, leading to significant cost savings.
Conclusion
In conclusion, data cleansing is a crucial process in maintaining the quality of data. It involves identifying and correcting or removing errors, inaccuracies, and inconsistencies in datasets. Despite its challenges, data cleansing offers numerous benefits, including improved decision-making, increased operational efficiency, compliance with regulations, and cost savings.
With the growing importance of data in today’s digital age, the role of data cleansing has become more significant than ever. Therefore, businesses should invest in effective data cleansing strategies and tools to ensure the integrity and reliability of their data.