What is customer propensity modeling?
Customer propensity modeling is a crucial aspect of modern marketing strategies. It refers to the process of using statistical models and algorithms to predict the likelihood of specific customer behaviors. These behaviors can range from making a purchase, clicking on a link, subscribing to a service, or even churning from a business. The primary objective of customer propensity modeling is to enable businesses to understand their customers better, anticipate their needs, and tailor their marketing efforts accordingly.
With the advent of big data and advanced analytics, customer propensity modeling has evolved significantly. It is no longer about merely predicting customer behaviors but also about understanding the underlying factors that drive these behaviors. This comprehensive approach allows businesses to create more personalized and effective marketing campaigns, thereby improving customer satisfaction and loyalty.
Understanding the Basics of Customer Propensity Modeling
At its core, customer propensity modeling is about predicting future customer behavior based on past behavior and other relevant data. This is achieved through the use of statistical models and machine learning algorithms that analyze historical customer data and identify patterns and trends. These patterns and trends are then used to predict future behaviors.
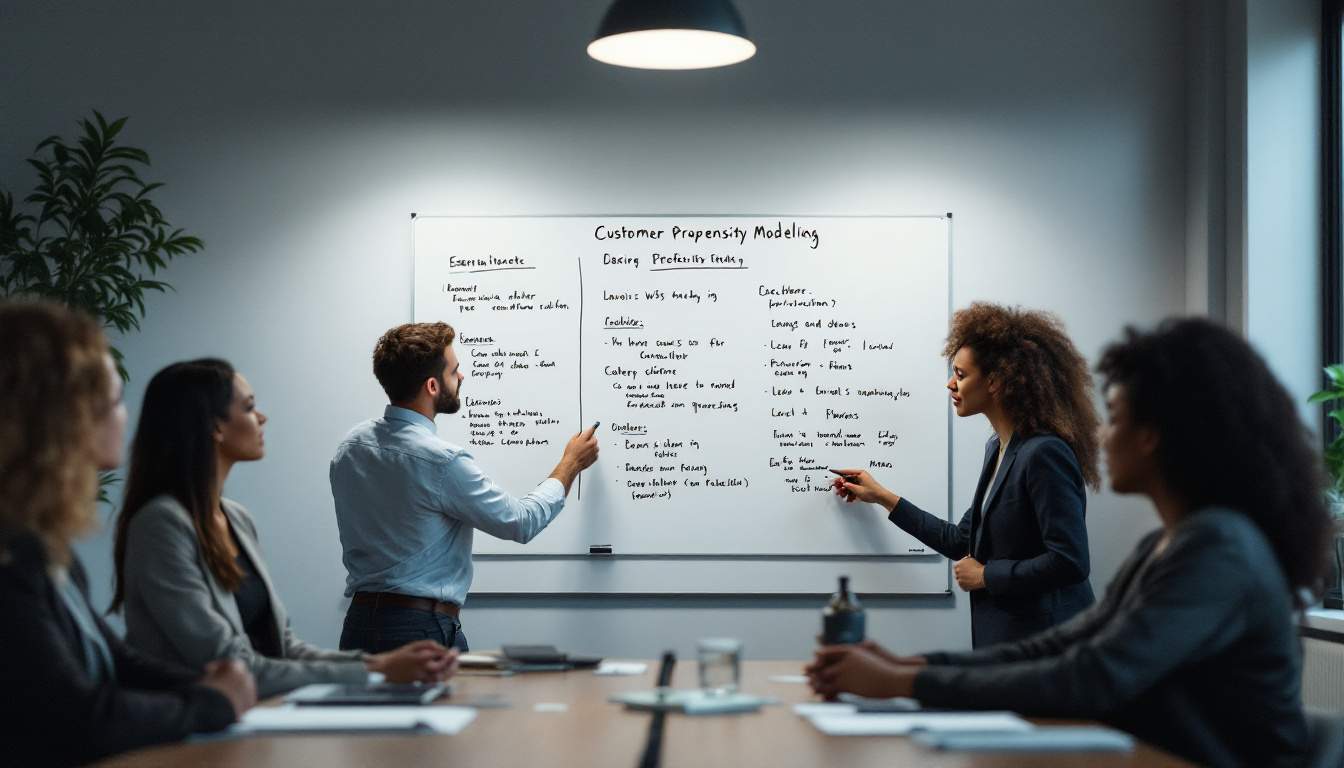
However, customer propensity modeling is not a one-size-fits-all solution. Different businesses may require different models depending on their specific needs and objectives. For instance, a retail business may be interested in predicting the likelihood of a customer making a purchase, while a telecom company may want to predict the likelihood of a customer churning.
Types of Customer Propensity Models
There are several types of customer propensity models, each with its own strengths and weaknesses. Some of the most common types include logistic regression, decision trees, random forests, and neural networks. The choice of model depends on various factors such as the nature of the data, the complexity of the problem, and the specific objectives of the business.
Logistic regression, for instance, is a simple and widely used model that is particularly effective for binary classification problems. Decision trees and random forests, on the other hand, are more complex models that can handle multi-class classification problems and feature interactions. Neural networks are even more complex and can model non-linear relationships, but they require large amounts of data and computational resources.
Key Components of Customer Propensity Models
Regardless of the type of model used, there are several key components that are common to all customer propensity models. These include the target variable, the predictor variables, and the model parameters.
The target variable is the behavior that the model aims to predict. This could be a binary variable (e.g., whether a customer will make a purchase or not) or a continuous variable (e.g., the amount a customer will spend). The predictor variables are the factors that are believed to influence the target variable. These could include demographic data, transaction history, browsing behavior, and more. The model parameters are the weights assigned to each predictor variable, which determine their influence on the target variable.
Applications of Customer Propensity Modeling
Customer propensity modeling has a wide range of applications in marketing. These include customer segmentation, targeted marketing, customer retention, and customer lifetime value prediction.
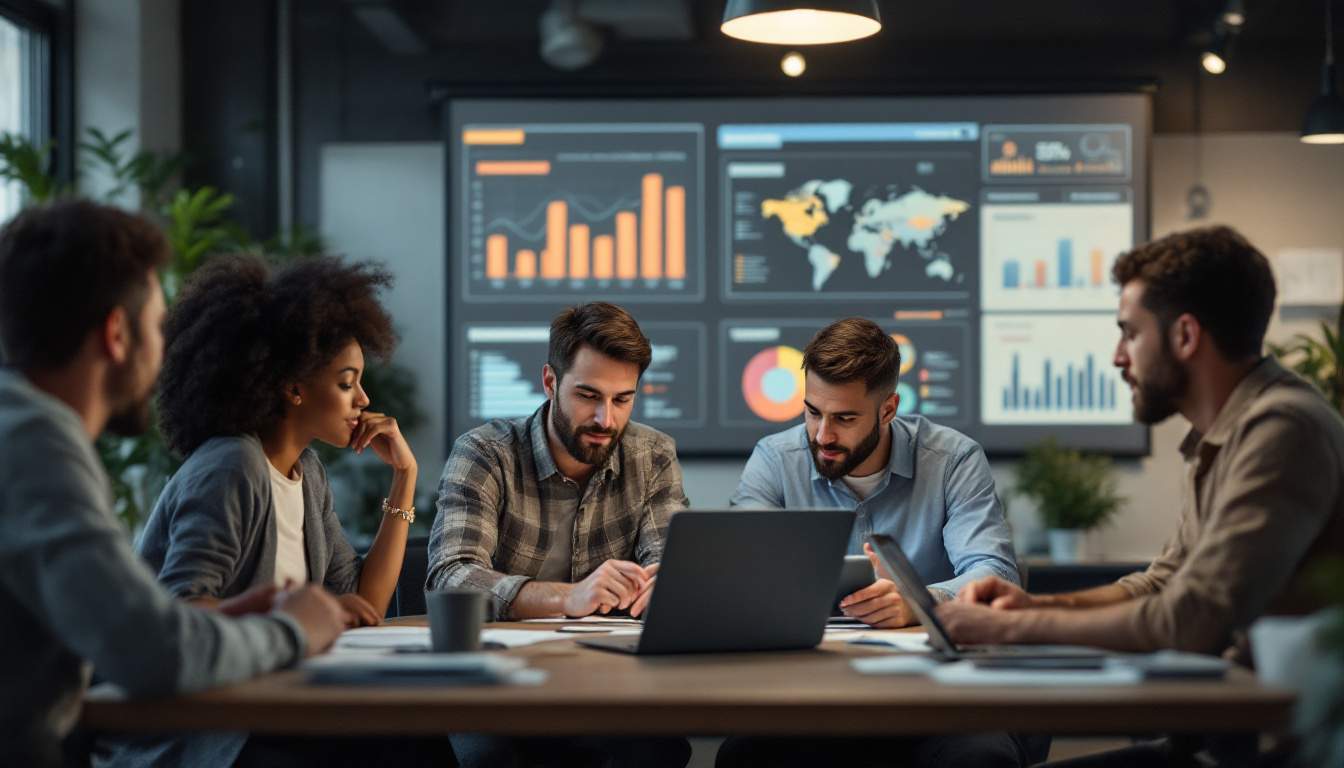
Customer segmentation involves dividing a customer base into distinct groups based on their behaviors, preferences, and characteristics. Customer propensity models can help identify these groups and understand their behaviors, thereby enabling businesses to tailor their marketing efforts to each group’s needs and preferences.
Targeted Marketing
One of the most common applications of customer propensity modeling is in targeted marketing. By predicting the likelihood of a customer responding to a particular marketing campaign, businesses can focus their efforts on those customers who are most likely to respond positively. This not only improves the effectiveness of the marketing campaign but also reduces costs by avoiding wasted efforts on unresponsive customers.
For instance, an online retailer could use a customer propensity model to predict the likelihood of a customer clicking on a promotional email. Based on this prediction, the retailer could then decide whether to send the email to that customer or not.
Customer Retention
Customer propensity modeling can also be used to improve customer retention. By predicting the likelihood of a customer churning, businesses can take proactive measures to retain those customers who are at risk of leaving. This could involve offering special discounts, personalized offers, or improved customer service.
For example, a telecom company could use a customer propensity model to predict the likelihood of a customer churning. Based on this prediction, the company could then take appropriate measures to retain that customer, such as offering a better plan or addressing any issues the customer may have.
Challenges in Customer Propensity Modeling
Despite its many benefits, customer propensity modeling is not without its challenges. These include data quality issues, model complexity, and ethical considerations.
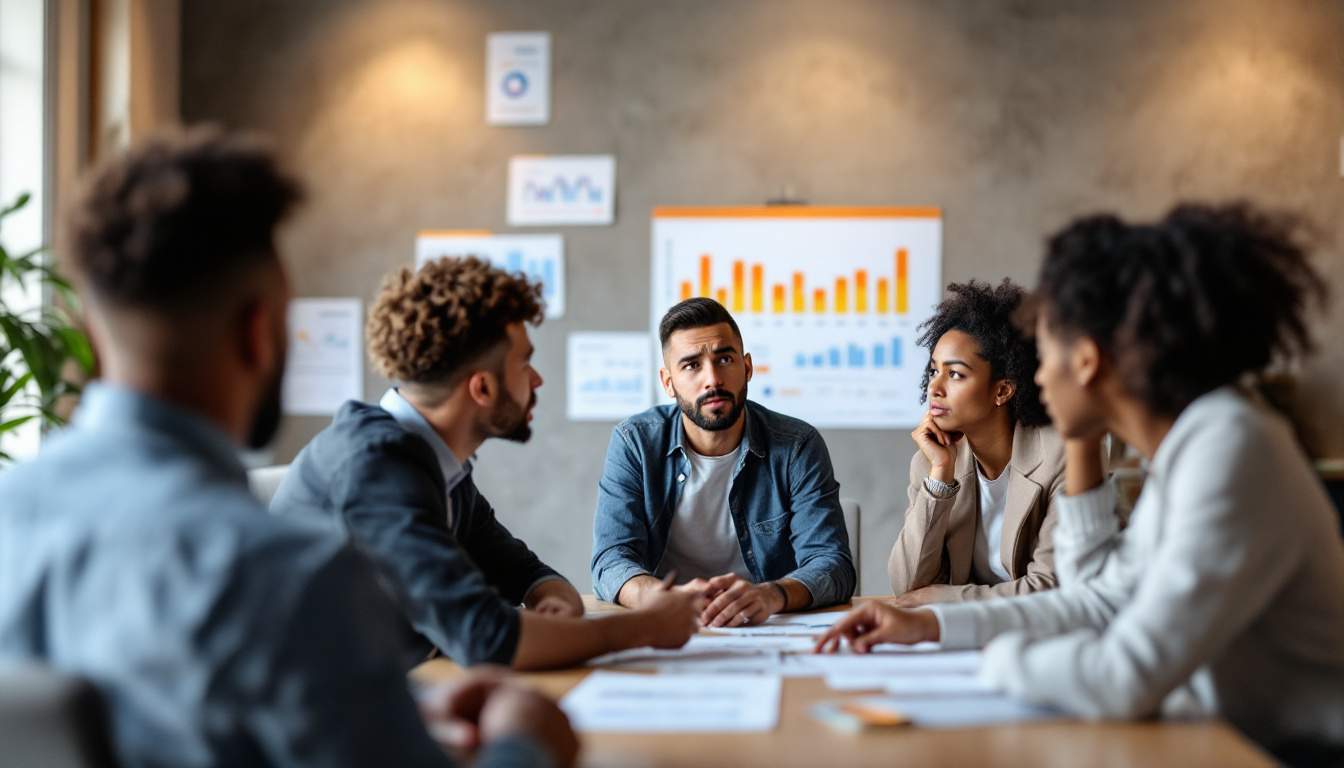
Data quality is a major concern in customer propensity modeling. The accuracy of the model’s predictions depends largely on the quality of the data used to train the model. If the data is incomplete, inaccurate, or biased, the model’s predictions will also be inaccurate or biased. Therefore, it is crucial to ensure that the data used for customer propensity modeling is clean, accurate, and representative of the customer base.
Model Complexity
Another challenge in customer propensity modeling is model complexity. While complex models can capture more nuanced relationships and interactions, they are also more difficult to interpret and validate. This can make it difficult to understand why the model is making certain predictions, which can be problematic in certain contexts.
For instance, if a customer propensity model is used to deny a customer a loan or a credit card, it is important to be able to explain why the model made that decision. If the model is too complex to interpret, this could lead to legal and ethical issues.
Ethical Considerations
Finally, there are ethical considerations to take into account when using customer propensity modeling. While these models can be very powerful tools for understanding and predicting customer behavior, they can also be misused to manipulate customers or invade their privacy.
For instance, if a customer propensity model is used to target vulnerable customers with predatory marketing tactics, this could be considered unethical. Similarly, if the model uses sensitive personal data without the customer’s consent, this could be a violation of privacy laws. Therefore, it is crucial to use customer propensity modeling responsibly and ethically.
Conclusion
Customer propensity modeling is a powerful tool for understanding and predicting customer behavior. It can help businesses tailor their marketing efforts, improve customer retention, and increase customer lifetime value. However, it is not without its challenges, including data quality issues, model complexity, and ethical considerations. Therefore, it is crucial to approach customer propensity modeling with care, ensuring that the data used is accurate and representative, the models are interpretable and valid, and the ethical implications are carefully considered.
Despite these challenges, customer propensity modeling has the potential to revolutionize the way businesses interact with their customers. By providing a deeper understanding of customer behavior, it can enable businesses to create more personalized and effective marketing campaigns, thereby improving customer satisfaction and loyalty. As such, it is likely to remain a key component of modern marketing strategies for the foreseeable future.