What is churn prediction?
Churn prediction refers to the process of identifying customers who are likely to stop using your product or service in the future. This predictive analysis is crucial for businesses as it allows them to take proactive measures to retain these customers, thereby reducing churn rate and increasing profitability.
Churn prediction is not a standalone concept but is deeply intertwined with other marketing concepts like customer lifetime value, customer retention, and customer satisfaction. It is a proactive approach towards customer management, focusing on understanding customer behavior and preferences to provide personalized experiences and thereby increase customer loyalty.
Understanding Churn Prediction
Churn prediction is based on the principle of predictive analytics, which involves using statistical algorithms and machine learning techniques to predict future outcomes based on historical data. In the context of churn prediction, this data is usually customer usage data, customer interaction data, and other relevant customer information.
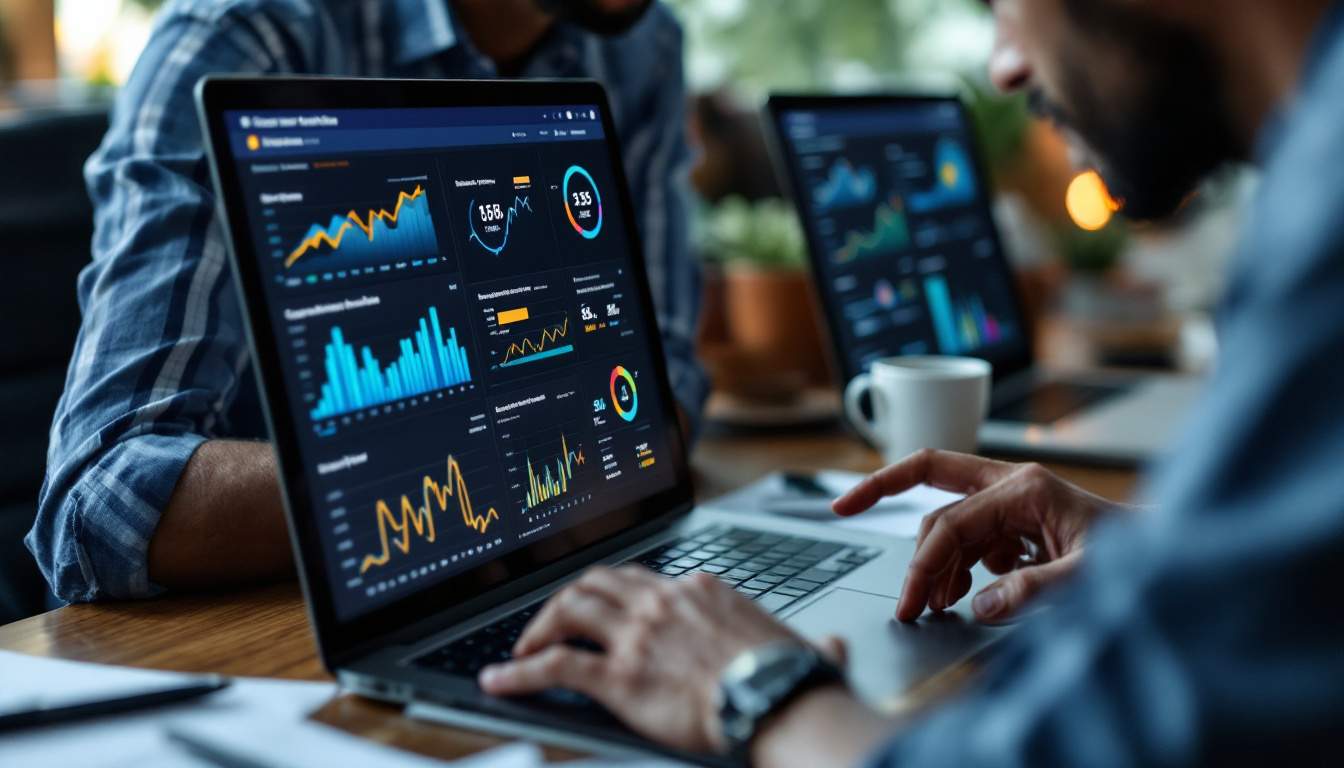
The goal of churn prediction is to identify the likelihood of a customer discontinuing their business with a company. This information is then used to implement strategies aimed at preventing this churn, thereby increasing customer retention and ultimately, the company’s bottom line.
Importance of Churn Prediction
Churn prediction is of immense importance in today’s competitive business environment. With the cost of acquiring a new customer being five times more than retaining an existing one, businesses cannot afford to ignore the importance of customer retention. Churn prediction helps businesses identify at-risk customers in advance, allowing them to take proactive measures to retain these customers.
Moreover, churn prediction also helps businesses understand why customers are leaving. This understanding can then be used to improve the product or service, thereby reducing the overall churn rate. In this way, churn prediction not only helps in customer retention but also in improving the overall customer experience.
Churn Prediction Models
There are several models used for churn prediction, each with its own strengths and weaknesses. Some of the most commonly used models include logistic regression, decision trees, random forests, and neural networks. The choice of model depends on the nature of the data, the business context, and the specific requirements of the churn prediction task.
For instance, logistic regression is a simple and interpretable model that works well when the relationship between the predictors and the outcome is linear. On the other hand, decision trees and random forests are more flexible and can capture complex non-linear relationships, but they may be harder to interpret. Neural networks, on the other hand, are extremely powerful and can model complex non-linear relationships, but they require large amounts of data and can be difficult to interpret.
Implementing Churn Prediction
Implementing churn prediction involves several steps, starting with data collection and ending with model deployment. The first step is to collect relevant customer data, which can include demographic data, usage data, interaction data, and more. This data is then preprocessed to remove any inconsistencies or errors.
The next step is feature engineering, which involves creating predictive features from the data. These features are then used to train a predictive model, which is then validated and optimized. Finally, the model is deployed and used to predict customer churn.
Data Collection and Preprocessing
Data collection is the first step in implementing churn prediction. This involves collecting relevant customer data, which can include demographic data, usage data, interaction data, and more. The quality and quantity of the data collected will directly impact the accuracy of the churn prediction model.
Once the data is collected, it needs to be preprocessed. This involves cleaning the data to remove any inconsistencies or errors, dealing with missing values, and transforming the data into a format suitable for modeling. This step is crucial as the quality of the data directly impacts the accuracy of the churn prediction model.
Feature Engineering
Feature engineering is the process of creating predictive features from the data. These features are the variables that the model uses to make predictions. Feature engineering can involve creating new features from existing ones, transforming features to make them more suitable for modeling, and selecting the most predictive features.
The goal of feature engineering is to create a set of features that capture the underlying patterns in the data and are predictive of the outcome. This step is crucial as the quality of the features directly impacts the accuracy of the churn prediction model.
Challenges in Churn Prediction
Despite its importance, churn prediction is not without its challenges. One of the main challenges is the availability and quality of data. For churn prediction to be effective, businesses need access to comprehensive and accurate customer data. However, collecting such data can be difficult, particularly for small businesses or businesses in industries where customer interactions are less frequent.
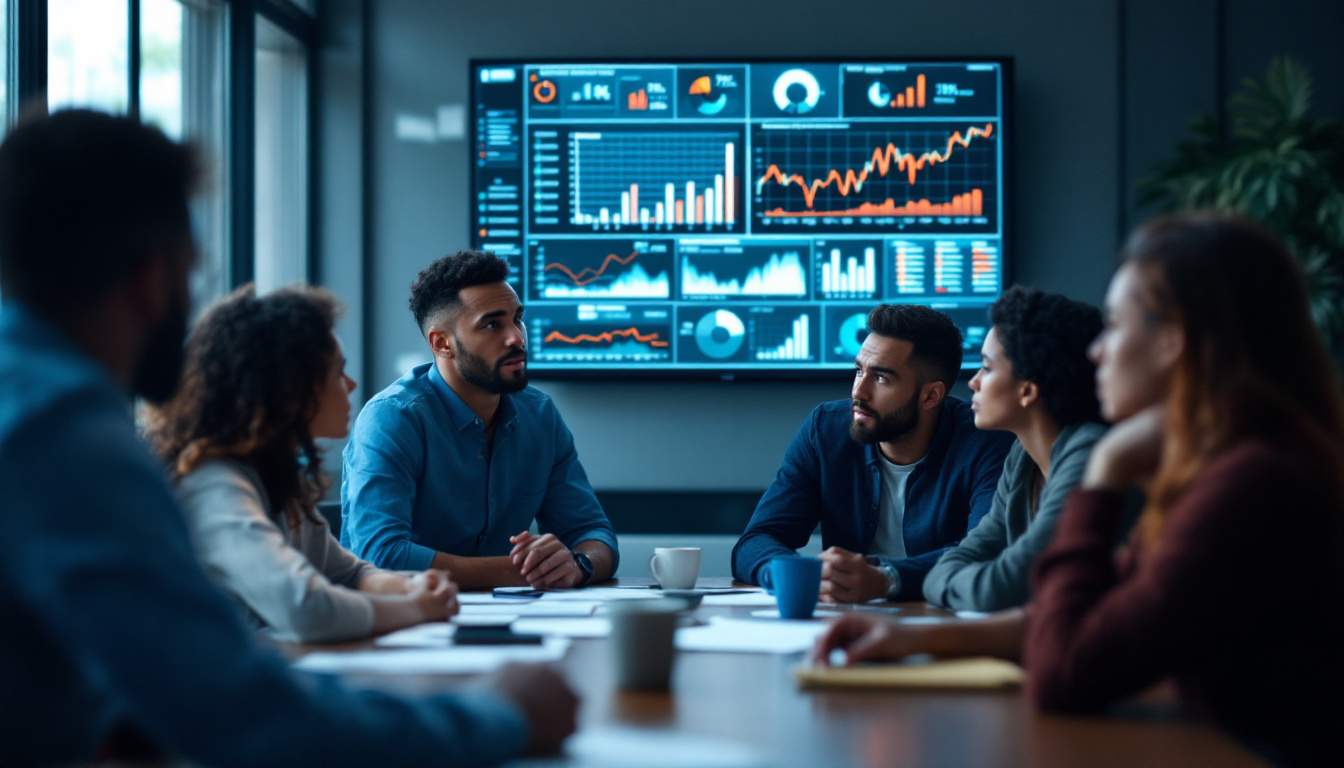
Another challenge is the dynamic nature of customer behavior. Customer preferences and behaviors can change over time, and a model that was accurate a few months ago may no longer be accurate. This requires businesses to continuously update and retrain their churn prediction models, which can be resource-intensive.
Overcoming Challenges
Despite these challenges, there are ways to improve the effectiveness of churn prediction. One approach is to use advanced machine learning techniques that can handle complex and dynamic data. These techniques can model complex relationships and adapt to changes in customer behavior, making them more accurate and robust.
Another approach is to invest in data collection and management. This involves not only collecting more data but also improving the quality of the data collected. This can be achieved through better data collection practices, data cleaning, and data integration.
Future of Churn Prediction
With the advancements in artificial intelligence and machine learning, the future of churn prediction looks promising. These technologies are enabling businesses to make more accurate predictions, handle larger amounts of data, and adapt to changes in customer behavior more quickly.
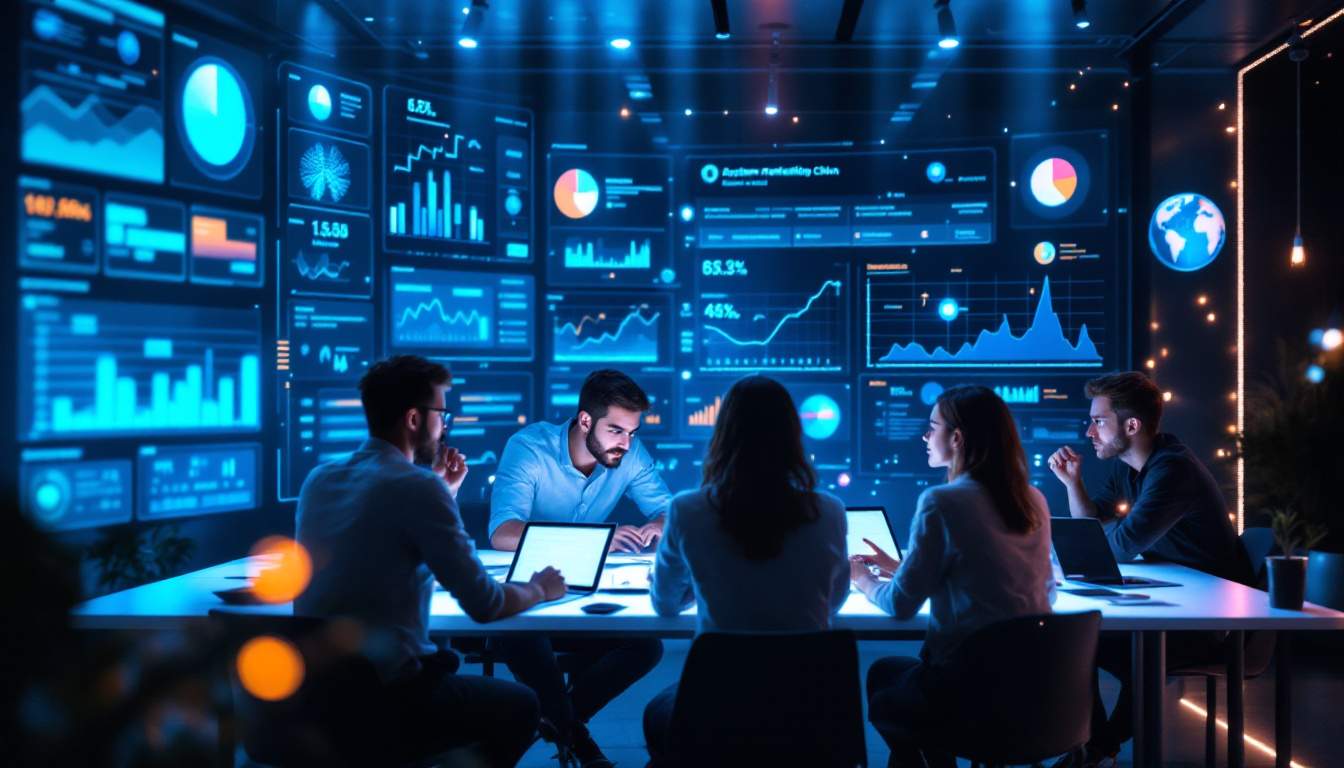
Moreover, as businesses become more customer-centric, the importance of churn prediction is only likely to increase. By predicting churn, businesses can not only retain existing customers but also improve their products and services, leading to higher customer satisfaction and loyalty.
Role of AI and Machine Learning
Artificial intelligence and machine learning are playing a key role in the future of churn prediction. These technologies are enabling businesses to handle larger amounts of data, make more accurate predictions, and adapt to changes in customer behavior more quickly.
For instance, deep learning, a subset of machine learning, is being used to model complex non-linear relationships in customer data, leading to more accurate churn predictions. Similarly, reinforcement learning is being used to optimize customer retention strategies, leading to lower churn rates.
Importance of Customer-Centricity
As businesses become more customer-centric, the importance of churn prediction is only likely to increase. By predicting churn, businesses can not only retain existing customers but also improve their products and services, leading to higher customer satisfaction and loyalty.
Moreover, with the rise of digital technologies, businesses have access to more customer data than ever before. This data can be used to understand customer behavior and preferences, leading to more personalized and effective customer retention strategies.